AUC Score :
Short-term Tactic1 :
Dominant Strategy :
Time series to forecast n:
ML Model Testing : Modular Neural Network (DNN Layer)
Hypothesis Testing : Wilcoxon Rank-Sum Test
Surveillance : Major exchange and OTC
1Short-term revised.
2Time series is updated based on short-term trends.
Key Points
Based on current trends, Procore is projected to experience continued growth, driven by the increasing adoption of its construction management platform and expansion into new markets. However, the company faces risks including intense competition from established players and emerging startups, potential economic downturns impacting construction spending, and the challenges of integrating acquired businesses. Failure to innovate rapidly or maintain customer satisfaction could also negatively impact its performance. Furthermore, Procore's valuation could be vulnerable to shifts in investor sentiment toward growth stocks.About Procore Technologies
Procore Technologies, Inc. is a leading provider of construction management software. The company offers a cloud-based platform designed to connect all stakeholders on a construction project, from owners and general contractors to subcontractors. Its software provides solutions for project management, financial management, quality and safety management, and resource management. Procore's platform aims to improve efficiency, reduce risk, and increase profitability for its users by streamlining workflows and centralizing project information.
Procore primarily serves the construction industry, including residential, commercial, and industrial projects. The company's software solutions are used by a broad range of construction professionals worldwide. Procore's business model focuses on subscription-based software as a service (SaaS). The company generates revenue through recurring subscriptions, offering various pricing tiers depending on the size and needs of the user. Procore's growth strategy focuses on expanding its product offerings, increasing its customer base, and expanding its geographic footprint.
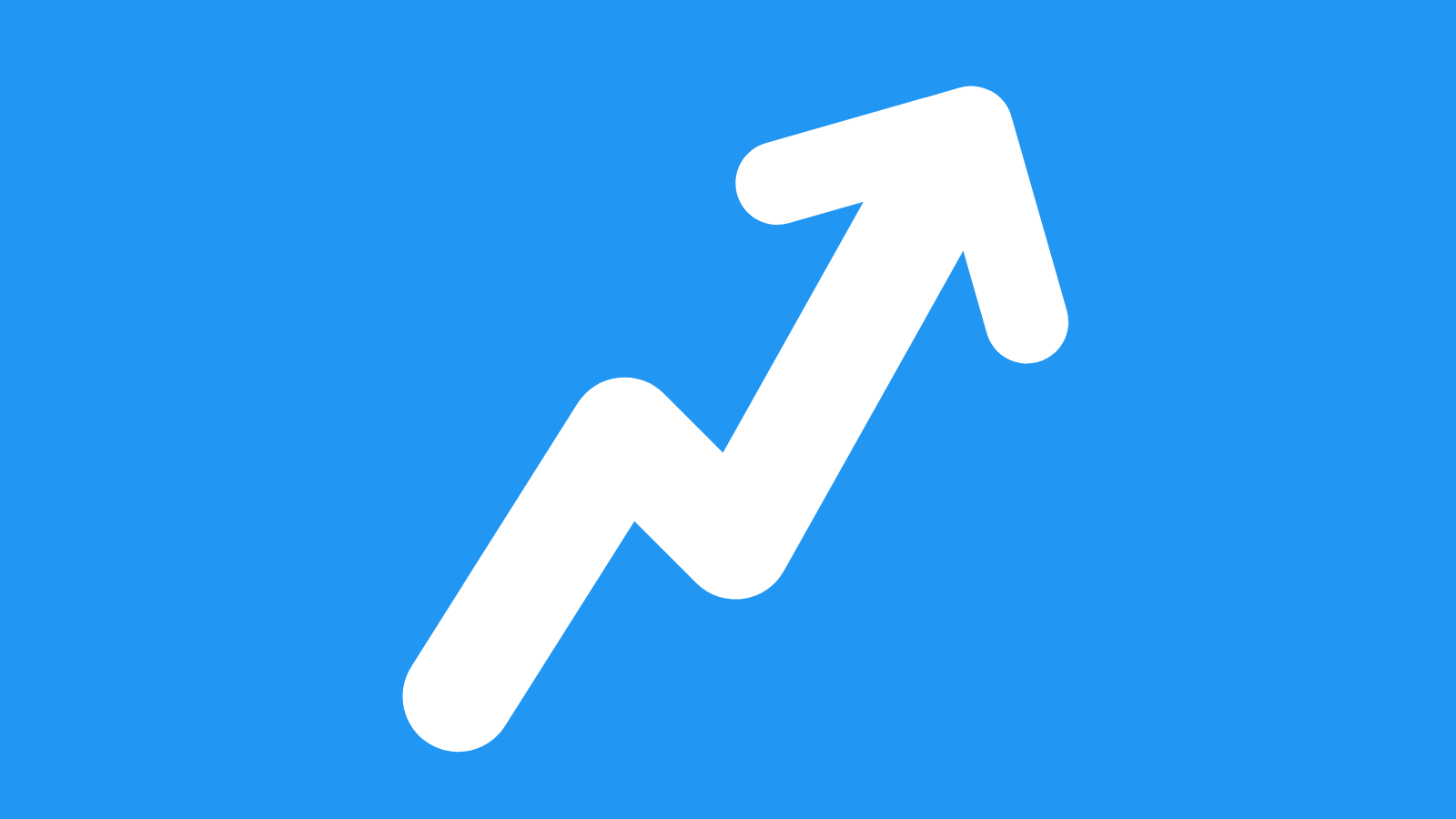
PCOR Stock Forecast Machine Learning Model
As a collective of data scientists and economists, we propose a comprehensive machine learning model for forecasting Procore Technologies Inc. (PCOR) common stock performance. Our approach leverages a multi-faceted strategy, incorporating both fundamental and technical analysis. For fundamental analysis, we will gather and analyze key financial indicators, including revenue growth, profitability margins (gross, operating, and net), debt-to-equity ratios, and cash flow metrics. Furthermore, we'll incorporate macroeconomic factors like interest rates, inflation, and overall industry trends within the construction technology sector. These macroeconomic indicators will be sourced from reputable economic databases and government agencies.
The technical analysis component of our model will involve analyzing historical PCOR stock data, including opening, closing, high, and low prices, along with trading volumes. We will employ a suite of technical indicators, such as moving averages (simple and exponential), relative strength index (RSI), MACD, and Bollinger Bands. Additionally, we'll consider chart patterns and candlestick formations to identify potential support and resistance levels. Data cleaning and preprocessing will be crucial steps, involving handling missing values, outlier detection, and feature scaling to optimize model performance. We will employ different types of models which includes, Recurrent Neural Networks (RNNs), specifically Long Short-Term Memory (LSTM) networks, due to their proficiency in handling sequential data such as stock prices, and Gradient Boosting models like XGBoost to consider their ability to capture complex relationships.
The final model will be an ensemble of these machine learning models, designed to integrate the predictive power of both fundamental and technical analysis. Model validation will be conducted through rigorous testing using historical data, employing metrics such as Mean Absolute Error (MAE), Root Mean Squared Error (RMSE), and Mean Absolute Percentage Error (MAPE) to evaluate forecast accuracy. Regular model retraining and parameter tuning will be required to adapt to evolving market conditions and maintain optimal performance. Risk management strategies, including stop-loss orders and position sizing, will be considered to mitigate potential losses. The model will produce probabilistic forecasts, providing both a point estimate and a range of possible outcomes, allowing for more informed investment decisions.
ML Model Testing
n:Time series to forecast
p:Price signals of Procore Technologies stock
j:Nash equilibria (Neural Network)
k:Dominated move of Procore Technologies stock holders
a:Best response for Procore Technologies target price
For further technical information as per how our model work we invite you to visit the article below:
How do KappaSignal algorithms actually work?
Procore Technologies Stock Forecast (Buy or Sell) Strategic Interaction Table
Strategic Interaction Table Legend:
X axis: *Likelihood% (The higher the percentage value, the more likely the event will occur.)
Y axis: *Potential Impact% (The higher the percentage value, the more likely the price will deviate.)
Z axis (Grey to Black): *Technical Analysis%
Procore Technologies Financial Outlook and Forecast
The financial outlook for Procore, a leading provider of construction management software, appears promising, underpinned by favorable industry trends and the company's strong market position. The construction industry is undergoing a digital transformation, with increasing adoption of software solutions to enhance efficiency, collaboration, and project management. Procore is strategically positioned to benefit from this shift. The company's robust software platform, offering a comprehensive suite of tools for various construction project phases, provides a significant competitive advantage. Revenue growth is projected to remain solid, fueled by new customer acquisition, expansion within existing accounts, and the ongoing trend of customers upgrading to more advanced features. Furthermore, the recurring revenue model, characteristic of software-as-a-service (SaaS) businesses, provides a degree of predictability and stability to the company's financial performance. Market analysts generally expect consistent revenue growth, likely driven by the need to streamline project workflows and maintain control of costs.
Procore's profitability trajectory presents a more nuanced picture. While the company has demonstrated strong revenue growth, its path to profitability has taken longer than initially expected. Significant investments in research and development, sales, and marketing are necessary to sustain its competitive edge and capture market share. The company is focused on achieving profitability through a combination of revenue growth and operational efficiency improvements. This includes strategic pricing adjustments, optimization of sales and marketing spending, and ongoing efforts to streamline its operational processes. The subscription-based revenue model offers some margin stability, but achieving sustainable profitability will depend on Procore's ability to balance revenue growth with cost management, particularly in the highly competitive software market. Gross margins are expected to remain relatively stable as the company focuses on expanding its product offerings and increasing average revenue per user.
From a valuation perspective, Procore's stock is likely to be priced based on its growth prospects, market position, and profitability potential. Investors will be keen to assess Procore's ability to generate free cash flow. The growth will be driven by increasing investment in infrastructure projects, the adoption of cloud-based solutions, and the expanding need for construction project management software. Market sentiment towards the company could be swayed by its ability to exceed revenue expectations, improve profitability metrics, and effectively manage its cash flow. Strategic partnerships, successful product innovations, and a well-defined go-to-market strategy would further bolster investor confidence and valuation. Further acquisitions and product integration could also play a vital role in revenue growth and market expansion.
Overall, the financial forecast for Procore is cautiously optimistic. The prediction is for continued revenue growth, albeit with a potentially longer-term view toward profitability. The primary risks include increased competition from established players and emerging rivals, any potential slowdown in construction activity, and unforeseen economic headwinds. The ability to efficiently convert revenue growth into sustainable profitability will be a key factor in the company's overall success and future stock performance. Moreover, successful execution of the strategic growth plans, including international expansion and product innovation, would be significant drivers. The company's ability to adapt and evolve to meet the changing demands of the construction industry is a critical element to the long-term outlook.
Rating | Short-Term | Long-Term Senior |
---|---|---|
Outlook | B2 | Ba1 |
Income Statement | C | Ba3 |
Balance Sheet | C | B2 |
Leverage Ratios | Ba3 | Ba1 |
Cash Flow | Ba3 | Baa2 |
Rates of Return and Profitability | Baa2 | Baa2 |
*Financial analysis is the process of evaluating a company's financial performance and position by neural network. It involves reviewing the company's financial statements, including the balance sheet, income statement, and cash flow statement, as well as other financial reports and documents.
How does neural network examine financial reports and understand financial state of the company?
References
- N. B ̈auerle and A. Mundt. Dynamic mean-risk optimization in a binomial model. Mathematical Methods of Operations Research, 70(2):219–239, 2009.
- Bastani H, Bayati M. 2015. Online decision-making with high-dimensional covariates. Work. Pap., Univ. Penn./ Stanford Grad. School Bus., Philadelphia/Stanford, CA
- Mnih A, Teh YW. 2012. A fast and simple algorithm for training neural probabilistic language models. In Proceedings of the 29th International Conference on Machine Learning, pp. 419–26. La Jolla, CA: Int. Mach. Learn. Soc.
- Bai J, Ng S. 2002. Determining the number of factors in approximate factor models. Econometrica 70:191–221
- uyer, S. Whiteson, B. Bakker, and N. A. Vlassis. Multiagent reinforcement learning for urban traffic control using coordination graphs. In Machine Learning and Knowledge Discovery in Databases, European Conference, ECML/PKDD 2008, Antwerp, Belgium, September 15-19, 2008, Proceedings, Part I, pages 656–671, 2008.
- Burkov A. 2019. The Hundred-Page Machine Learning Book. Quebec City, Can.: Andriy Burkov
- R. Williams. Simple statistical gradient-following algorithms for connectionist reinforcement learning. Ma- chine learning, 8(3-4):229–256, 1992