AUC Score :
Short-term Tactic1 :
Dominant Strategy :
Time series to forecast n:
ML Model Testing : Transfer Learning (ML)
Hypothesis Testing : Wilcoxon Rank-Sum Test
Surveillance : Major exchange and OTC
1Short-term revised.
2Time series is updated based on short-term trends.
Key Points
The OMXS30 index is expected to experience a period of moderate growth, driven by positive sentiment in the global markets and resilient performance from key sectors such as technology and healthcare. However, this positive outlook is tempered by several risks, including potential volatility stemming from geopolitical tensions, inflationary pressures, and uncertainty surrounding central bank policies. A sharper-than-anticipated economic slowdown in major trading partners or unforeseen negative developments in the financial sector could also exert significant downward pressure on the index, leading to unexpected and potentially substantial losses.About OMXS30 Index
OMXS30, also known as the OMX Stockholm 30, represents the thirty most actively traded stocks on the Nasdaq Stockholm exchange. It serves as a benchmark for the overall performance of the Swedish stock market, reflecting the collective value of its leading companies. The index is market capitalization-weighted, meaning that the influence of each company on the index's movement is proportional to its market capitalization, providing a snapshot of the broader economic landscape of Sweden and how it reflects in the most influential business in the country.
Regularly reviewed and rebalanced, the composition of OMXS30 can change to ensure its continued relevance. The index is widely utilized by investors for various purposes, including performance tracking, benchmarking of portfolios, and as an underlying asset for financial derivatives. It's a key indicator of Swedish financial health, often monitored by both domestic and international investors seeking insights into market trends and investment opportunities within the Swedish economy.
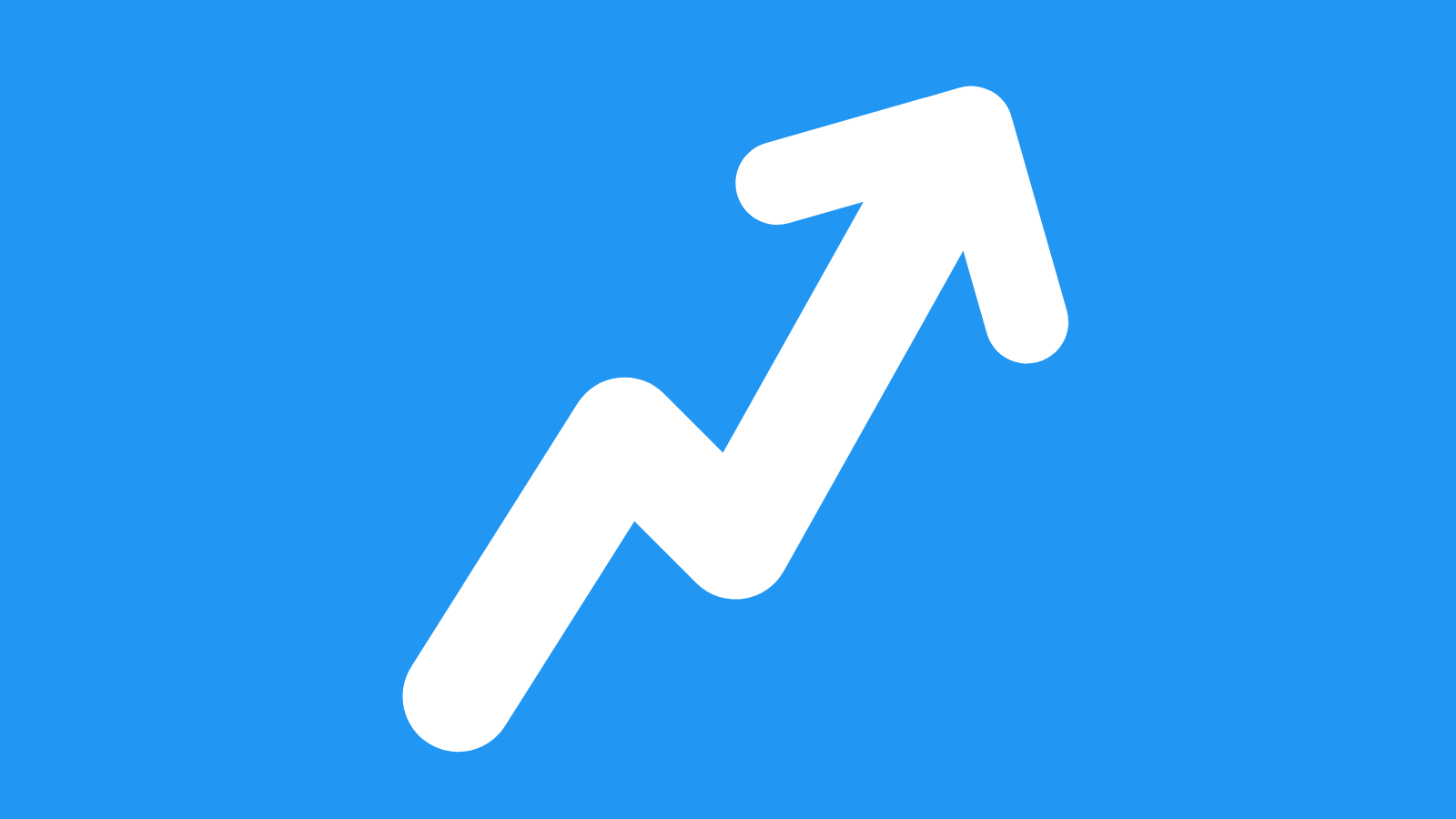
OMXS30 Index Forecasting Machine Learning Model
Our approach to forecasting the OMXS30 index involves a hybrid machine learning model leveraging both time series analysis and external economic indicators. We will employ a Recurrent Neural Network (RNN), specifically a Long Short-Term Memory (LSTM) network, to capture the temporal dependencies within the historical OMXS30 data. The LSTM architecture is chosen for its ability to handle long-range dependencies and mitigate the vanishing gradient problem often encountered in standard RNNs. Input features for the LSTM model will include lagged values of the OMXS30 index itself (e.g., past closing prices), as well as technical indicators such as moving averages, Relative Strength Index (RSI), and trading volume. To augment the predictive power, the model will integrate macroeconomic variables.
To account for external factors influencing the OMXS30, the model will incorporate economic indicators known to correlate with stock market performance. These include, but are not limited to: GDP growth, inflation rates, interest rates (both domestic and Eurozone), unemployment figures, and consumer confidence indices. Furthermore, we will incorporate sentiment analysis derived from news articles and social media data related to the Swedish economy and the broader global markets. These external indicators will be preprocessed and scaled before integration with the LSTM model. We will use a combination of feature engineering techniques, such as creating time-lagged versions and deriving interaction terms, to refine and optimize the feature set.
The training and validation process will be rigorously conducted. The historical data will be split into training, validation, and testing sets, and the model will be evaluated using appropriate metrics, such as Mean Squared Error (MSE), Root Mean Squared Error (RMSE), and Mean Absolute Error (MAE). Cross-validation techniques will be applied to ensure the robustness of the model. Regularization techniques (e.g., dropout, L1/L2 regularization) will be implemented to prevent overfitting. Hyperparameter tuning, encompassing the number of LSTM layers, the number of neurons in each layer, the optimization algorithm, and the learning rate, will be performed using techniques such as grid search or random search. The final, validated model will then be used to generate forecasts for the OMXS30 index, which will be continuously monitored and updated with new data to maintain forecasting accuracy.
ML Model Testing
n:Time series to forecast
p:Price signals of OMXS30 index
j:Nash equilibria (Neural Network)
k:Dominated move of OMXS30 index holders
a:Best response for OMXS30 target price
For further technical information as per how our model work we invite you to visit the article below:
How do KappaSignal algorithms actually work?
OMXS30 Index Forecast Strategic Interaction Table
Strategic Interaction Table Legend:
X axis: *Likelihood% (The higher the percentage value, the more likely the event will occur.)
Y axis: *Potential Impact% (The higher the percentage value, the more likely the price will deviate.)
Z axis (Grey to Black): *Technical Analysis%
OMXS30 Index: Financial Outlook and Forecast
The financial outlook for the OMXS30 index, representing the 30 most actively traded companies on the Nasdaq Stockholm, reflects a nuanced landscape shaped by global economic conditions, domestic Swedish policies, and sector-specific performance. Overall, the outlook points to moderate growth potential, albeit with inherent uncertainties. The Swedish economy, a significant driver of the OMXS30's performance, exhibits resilience, supported by a strong labor market and a relatively stable financial sector. However, this resilience is tested by the impact of persistent inflation and subsequent monetary policy tightening undertaken by Riksbank, Sweden's central bank. Furthermore, global headwinds, including the ongoing war in Ukraine, the slowdown in the Chinese economy, and persistent supply chain disruptions, pose risks to export-oriented Swedish companies, which constitute a considerable portion of the OMXS30 constituents.
Sector-specific analysis reveals varying prospects. The technology sector, a prominent component of the OMXS30, is subject to both opportunities and risks. The shift towards digitalization and sustainability is offering substantial growth prospects for companies involved in software, cloud computing, and renewable energy. However, this sector is simultaneously susceptible to fluctuations in investor sentiment, increased competition, and potential shifts in regulatory policies. Furthermore, the industrial sector, another significant contributor to the index, is facing challenges stemming from rising energy prices, supply chain disruptions, and changes in global trade patterns. Conversely, sectors such as healthcare and consumer staples, with relatively stable demand and less sensitivity to economic cycles, are anticipated to provide more consistent contributions to the index's overall performance. Strong earnings reports from key companies, and successful cost management could act as potential catalysts and positive drivers.
Macroeconomic factors play a critical role in shaping the forecast. Interest rate decisions by Riksbank are particularly significant. Further interest rate hikes to combat inflation could potentially hinder economic growth and negatively affect borrowing costs for Swedish businesses, impacting their profitability. Conversely, a more dovish approach from the Riksbank, potentially in response to a slowdown in economic activity, could stimulate investment and consumer spending, thus supporting the index. Fiscal policy also plays a role, and government initiatives aimed at promoting innovation, investing in infrastructure, and supporting green transition could positively influence the index's performance. International trade flows, particularly developments in the European Union and China, will have a notable impact given the Swedish economy's high degree of openness. Changes in the value of the Swedish Krona, affected by global sentiment, may also influence the returns on the OMXS30 for international investors.
The forecast for the OMXS30 index is moderately positive, with the potential for modest gains over the next year. The outlook relies on the resilience of the Swedish economy, the growth potential of key sectors, and the effectiveness of monetary and fiscal policies. However, this prediction is associated with several risks. Persistent inflation could lead to further interest rate hikes, dampening economic growth. Global economic slowdown and political instability would have a detrimental impact on export-oriented companies and investor confidence. Geopolitical tensions, especially the war in Ukraine and its consequences, represent major uncertainty. Finally, any unexpected shock to the financial markets worldwide could amplify market volatility. Therefore, investors should adopt a cautious approach, diversify their portfolios, and regularly monitor key economic indicators and sector-specific developments.
Rating | Short-Term | Long-Term Senior |
---|---|---|
Outlook | B2 | B2 |
Income Statement | B1 | Ba1 |
Balance Sheet | Caa2 | C |
Leverage Ratios | B1 | Baa2 |
Cash Flow | Caa2 | Caa2 |
Rates of Return and Profitability | B2 | C |
*An aggregate rating for an index summarizes the overall sentiment towards the companies it includes. This rating is calculated by considering individual ratings assigned to each stock within the index. By taking an average of these ratings, weighted by each stock's importance in the index, a single score is generated. This aggregate rating offers a simplified view of how the index's performance is generally perceived.
How does neural network examine financial reports and understand financial state of the company?
References
- Meinshausen N. 2007. Relaxed lasso. Comput. Stat. Data Anal. 52:374–93
- Mullainathan S, Spiess J. 2017. Machine learning: an applied econometric approach. J. Econ. Perspect. 31:87–106
- Athey S, Bayati M, Imbens G, Zhaonan Q. 2019. Ensemble methods for causal effects in panel data settings. NBER Work. Pap. 25675
- N. B ̈auerle and J. Ott. Markov decision processes with average-value-at-risk criteria. Mathematical Methods of Operations Research, 74(3):361–379, 2011
- Li L, Chen S, Kleban J, Gupta A. 2014. Counterfactual estimation and optimization of click metrics for search engines: a case study. In Proceedings of the 24th International Conference on the World Wide Web, pp. 929–34. New York: ACM
- A. Eck, L. Soh, S. Devlin, and D. Kudenko. Potential-based reward shaping for finite horizon online POMDP planning. Autonomous Agents and Multi-Agent Systems, 30(3):403–445, 2016
- V. Borkar. Q-learning for risk-sensitive control. Mathematics of Operations Research, 27:294–311, 2002.