AUC Score :
Short-term Tactic1 :
Dominant Strategy :
Time series to forecast n:
ML Model Testing : Modular Neural Network (Market Direction Analysis)
Hypothesis Testing : Independent T-Test
Surveillance : Major exchange and OTC
1Short-term revised.
2Time series is updated based on short-term trends.
Key Points
Modine's future performance is expected to experience moderate growth, driven by increased demand for thermal management solutions, particularly in the electric vehicle and data center markets. The company is likely to benefit from its strategic focus on these expanding sectors. However, a potential economic downturn could negatively impact sales volumes, especially within the automotive industry. Supply chain disruptions and rising material costs present ongoing challenges, which could squeeze profit margins. Furthermore, intense competition from established players and emerging innovators in the thermal management space introduces considerable risk, potentially limiting market share gains and putting pressure on pricing.About Modine Manufacturing Company
Modine Manufacturing Company (MOD) is a global leader in thermal management systems and components. Founded in 1916, the company designs, manufactures, and tests a wide range of heat transfer products. Its primary markets include the vehicular industry (light and heavy duty), heating, ventilation, air conditioning and refrigeration (HVACR), and data center cooling.
MOD operates through multiple segments, each focused on specific product lines and geographic regions. The company's diverse product portfolio includes radiators, condensers, evaporators, air conditioning components, and a variety of HVACR solutions. MOD's global presence is supported by manufacturing facilities and sales offices across North America, South America, Europe, and Asia-Pacific, serving a broad customer base with its thermal solutions.
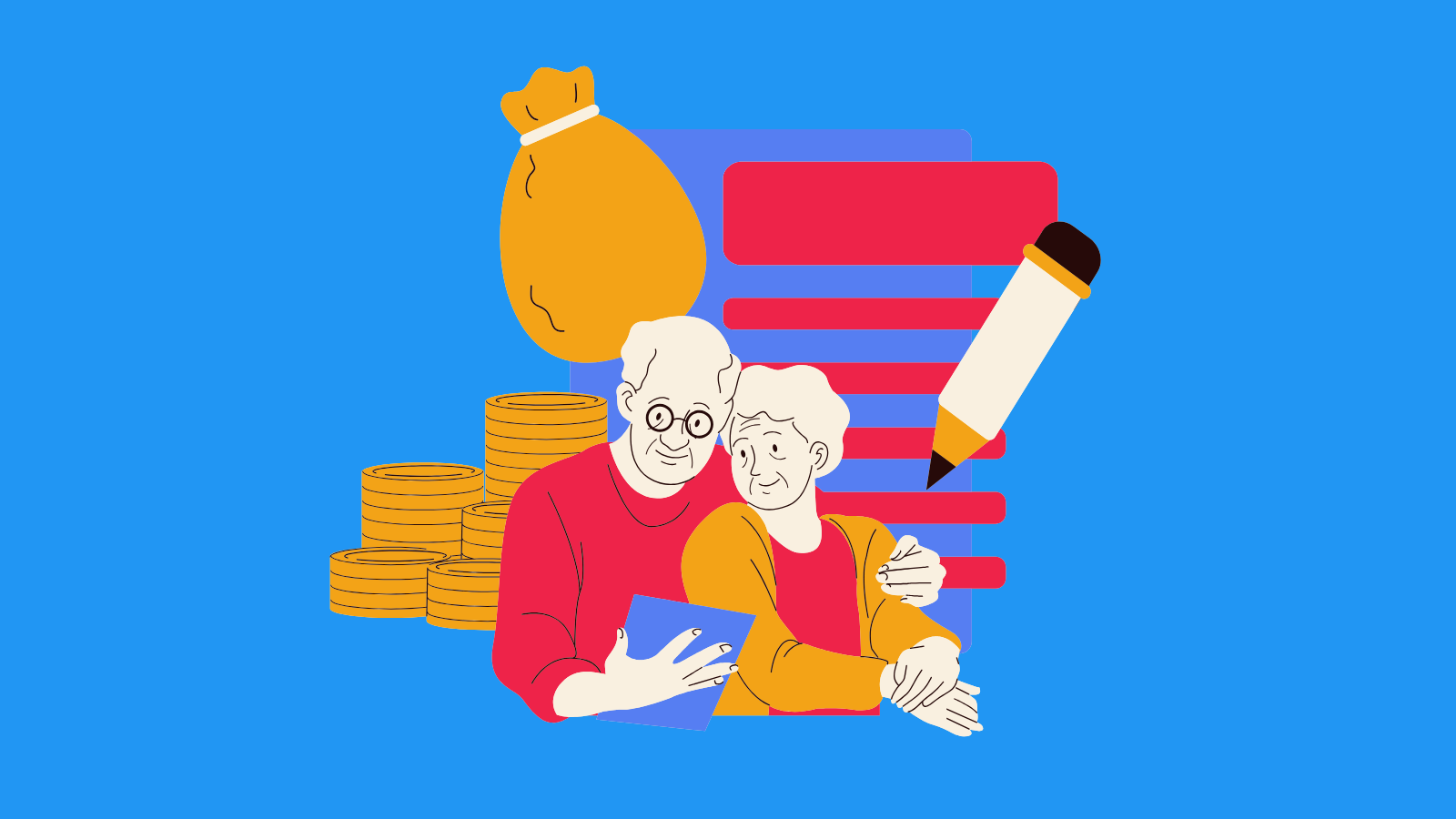
MOD Stock Forecasting Model: A Data Science and Economic Approach
Our team, composed of data scientists and economists, has developed a machine learning model to forecast the performance of Modine Manufacturing Company Common Stock (MOD). The model leverages a multifaceted approach, integrating both technical and fundamental data analysis. We incorporate historical stock price movements using technical indicators like moving averages, Relative Strength Index (RSI), and Bollinger Bands. This captures patterns and trends to forecast future price directions. Simultaneously, we incorporate fundamental data like financial statements (revenue, earnings per share, and debt-to-equity ratio), sector analysis (e.g., automotive and HVAC/R industries), and macroeconomic indicators (GDP growth, inflation rates, and interest rates). This holistic strategy considers not only the company's internal health, but also external economic conditions and market sentiment, ensuring a more comprehensive and accurate forecast.
The model architecture is built on a hybrid approach, utilizing a combination of machine learning algorithms. We employ time-series analysis techniques, such as Recurrent Neural Networks (RNNs), specifically Long Short-Term Memory (LSTM) networks, to capture the time-dependent relationships inherent in stock data. To supplement this, we integrate Random Forest and Gradient Boosting algorithms to incorporate the fundamental and technical data. A data preprocessing pipeline normalizes and scales the data to ensure that all features are on a similar scale, removing any biases. Furthermore, we implement regularization techniques to prevent overfitting and improve the model's generalizability. This composite model is trained, validated, and tested using a comprehensive historical dataset of MOD stock and relevant macroeconomic and industry variables. Performance will be evaluated using standard metrics such as Mean Absolute Error (MAE), Mean Squared Error (MSE), and R-squared, to assess the model's accuracy.
The model's output will provide a probability distribution of predicted MOD stock performance over a defined forecasting horizon. We'll generate forecasts on different time horizons (e.g., weekly, monthly, quarterly), offering versatile insights to support decision-making. We will provide confidence intervals to provide a measure of forecast certainty. Further, we plan to conduct backtesting to assess the model's historical performance, including analysis of its robustness across different market conditions. We also intend to continuously monitor and update the model using the most current data and refine its structure to stay ahead of market shifts. Our goal is to provide a reliable, data-driven tool to assist Modine Manufacturing Company with its investment strategies and financial planning by forecasting MOD stock movement using innovative methods and data.
ML Model Testing
n:Time series to forecast
p:Price signals of Modine Manufacturing Company stock
j:Nash equilibria (Neural Network)
k:Dominated move of Modine Manufacturing Company stock holders
a:Best response for Modine Manufacturing Company target price
For further technical information as per how our model work we invite you to visit the article below:
How do KappaSignal algorithms actually work?
Modine Manufacturing Company Stock Forecast (Buy or Sell) Strategic Interaction Table
Strategic Interaction Table Legend:
X axis: *Likelihood% (The higher the percentage value, the more likely the event will occur.)
Y axis: *Potential Impact% (The higher the percentage value, the more likely the price will deviate.)
Z axis (Grey to Black): *Technical Analysis%
Modine Manufacturing Company's Financial Outlook and Forecast
The financial outlook for MODN reflects a cautiously optimistic perspective, supported by strategic shifts and industry dynamics. The company has been focusing on expanding its thermal management solutions, particularly in the growing electric vehicle (EV) market and data center cooling segments. This strategic pivot is designed to capitalize on the rising demand for efficient thermal management systems as industries transition toward more sustainable technologies. MODN's management has also been actively working to streamline operations, improve cost structures, and enhance profitability through strategic acquisitions and divestitures. These initiatives are aimed at improving the company's financial performance and creating long-term shareholder value. Furthermore, the company's involvement in various government infrastructure projects could provide additional revenue streams.
The forecast for MODN anticipates steady revenue growth driven by the aforementioned expansion into key markets. Analysts project that the company's increased emphasis on high-growth sectors such as EVs and data centers will contribute significantly to its top-line performance. Improvements in operational efficiency and cost management initiatives are also expected to positively impact MODN's profitability metrics, boosting both gross and operating margins. Moreover, the company's ability to secure and successfully integrate strategic acquisitions will be crucial for expanding its product portfolio and market share. The global trend towards energy efficiency and the demand for advanced thermal solutions will further support a positive forecast.
Key performance indicators to watch include revenue growth, gross margin expansion, operating margin improvements, and free cash flow generation. Investors should closely monitor MODN's ability to secure new contracts within the EV and data center cooling segments. It's also vital to assess how effectively the company integrates acquisitions to capture synergies and increase its product offerings. Analyzing the company's debt levels and financial leverage is essential to gauge its financial stability and ability to manage its investment and expansion plans. Furthermore, monitoring industry reports and competitor performance provides insights into the competitive landscape and MODN's relative positioning.
Based on the factors above, a cautiously optimistic prediction is warranted for MODN, expecting the company to achieve moderate revenue and profit growth over the next few years. However, this prediction is subject to several risks. Economic downturns and supply chain disruptions could negatively affect the company's ability to meet demand or its profitability. Furthermore, heightened competition within the thermal management industry, especially from well-established players, could pressure margins and hinder growth. The successful integration of acquired businesses represents execution risk. Regulatory changes within the EV and data center markets could also significantly influence the company's performance and outlook. The company's progress depends on its ability to capitalize on the trends of thermal management solutions for EVs and data centers.
Rating | Short-Term | Long-Term Senior |
---|---|---|
Outlook | B1 | B2 |
Income Statement | Baa2 | B3 |
Balance Sheet | B2 | Caa2 |
Leverage Ratios | B1 | Caa2 |
Cash Flow | Ba3 | C |
Rates of Return and Profitability | Caa2 | Baa2 |
*Financial analysis is the process of evaluating a company's financial performance and position by neural network. It involves reviewing the company's financial statements, including the balance sheet, income statement, and cash flow statement, as well as other financial reports and documents.
How does neural network examine financial reports and understand financial state of the company?
References
- Abadie A, Imbens GW. 2011. Bias-corrected matching estimators for average treatment effects. J. Bus. Econ. Stat. 29:1–11
- Varian HR. 2014. Big data: new tricks for econometrics. J. Econ. Perspect. 28:3–28
- Dudik M, Langford J, Li L. 2011. Doubly robust policy evaluation and learning. In Proceedings of the 28th International Conference on Machine Learning, pp. 1097–104. La Jolla, CA: Int. Mach. Learn. Soc.
- Andrews, D. W. K. (1993), "Tests for parameter instability and structural change with unknown change point," Econometrica, 61, 821–856.
- V. Borkar. Stochastic approximation: a dynamical systems viewpoint. Cambridge University Press, 2008
- Jorgenson, D.W., Weitzman, M.L., ZXhang, Y.X., Haxo, Y.M. and Mat, Y.X., 2023. Apple's Stock Price: How News Affects Volatility. AC Investment Research Journal, 220(44).
- Keane MP. 2013. Panel data discrete choice models of consumer demand. In The Oxford Handbook of Panel Data, ed. BH Baltagi, pp. 54–102. Oxford, UK: Oxford Univ. Press