AUC Score :
Short-term Tactic1 :
Dominant Strategy :
Time series to forecast n:
ML Model Testing : Multi-Task Learning (ML)
Hypothesis Testing : Stepwise Regression
Surveillance : Major exchange and OTC
1Short-term revised.
2Time series is updated based on short-term trends.
Key Points
SGC is expected to exhibit moderate growth, driven by ongoing demand in its uniform and promotional products segments, alongside strategic partnerships that could broaden its market reach. The company may face risks related to supply chain disruptions, which have previously impacted its performance, and fluctuations in raw material costs. Furthermore, competitive pressures within the apparel industry and shifts in consumer preferences could limit revenue growth and profitability.About Superior Group
Superior Group of Companies Inc. (SGC) is a provider of customized uniform programs. The company designs, manufactures, and distributes a wide array of work apparel, uniforms, and accessories. SGC caters to various industries, including healthcare, hospitality, public safety, and industrial sectors. They offer a range of services, such as inventory management, online ordering systems, and garment customization. The company operates through several subsidiaries, each specializing in specific segments of the uniform market, which aids in their expansive market reach and client base.
SGC emphasizes its commitment to providing high-quality products and services. They focus on innovation in fabric technology and design to meet the evolving needs of its customers. The company also prioritizes sustainability in its operations, implementing eco-friendly practices in its manufacturing processes and supply chain. Through strategic acquisitions and organic growth, SGC has expanded its market position and aims to maintain its leadership in the uniform industry by continuously adapting to the demands of a dynamic market.
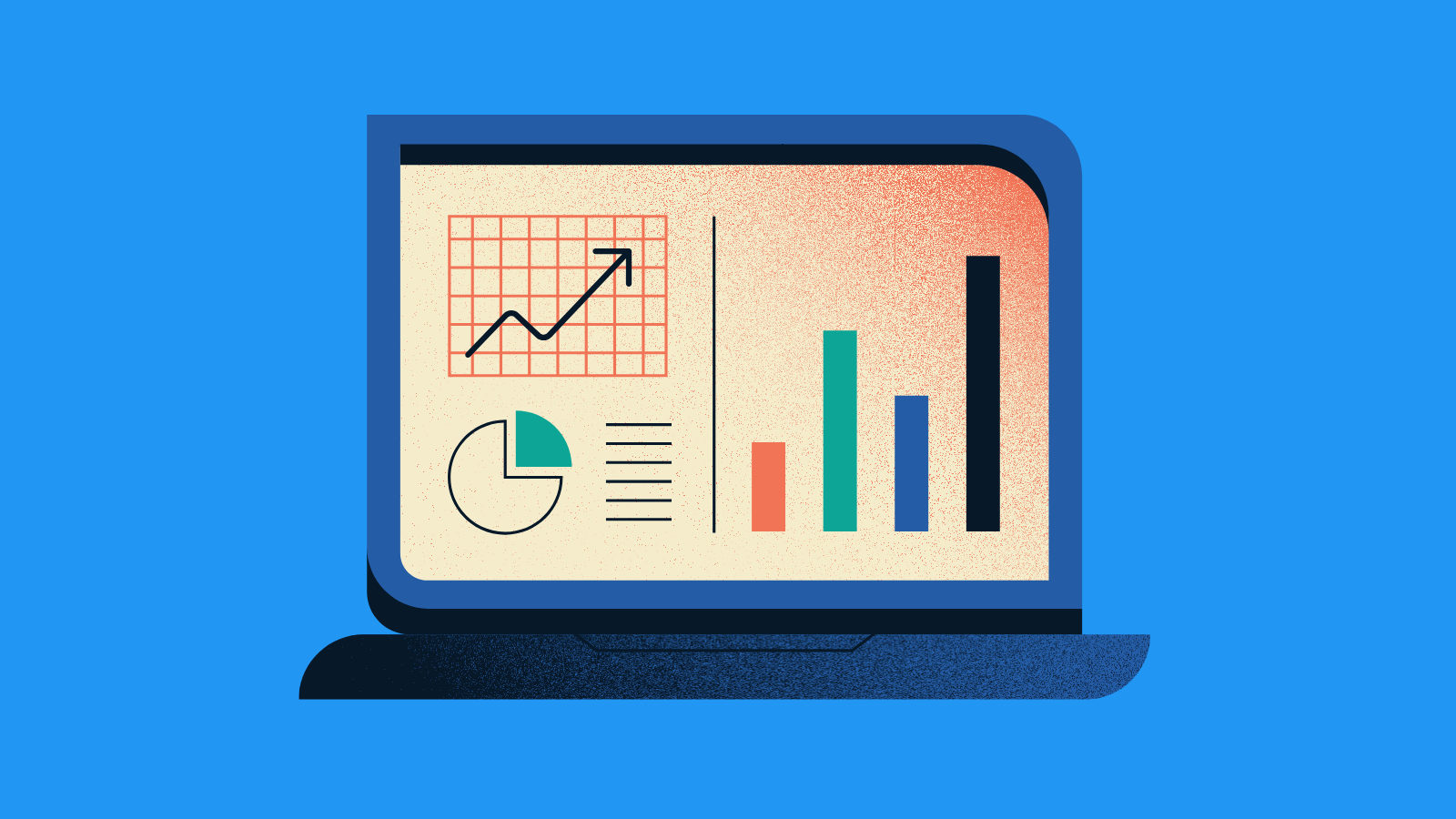
SGC Stock Forecast Model
Our team, comprised of data scientists and economists, has developed a comprehensive machine learning model to forecast the performance of Superior Group of Companies Inc. (SGC) common stock. The model incorporates a diverse range of input variables categorized into three primary groups: fundamental, technical, and macroeconomic indicators. Fundamental data includes quarterly and annual financial statements (revenue, earnings per share, debt-to-equity ratio, etc.), industry-specific metrics (e.g., market share, competitive landscape), and news sentiment analysis derived from textual data. Technical indicators encompass historical price and volume data, moving averages, momentum oscillators (RSI, MACD), and pattern recognition techniques. Macroeconomic variables incorporate interest rates, inflation, GDP growth, and consumer confidence to understand the broader economic environment's impact on SGC's performance. This multi-faceted approach allows the model to capture a holistic view of the factors influencing SGC stock.
The machine learning model leverages a hybrid approach, primarily utilizing a combination of Recurrent Neural Networks (RNNs) specifically Long Short-Term Memory (LSTM) networks and ensemble methods. LSTM networks are well-suited for time-series data, enabling the model to capture temporal dependencies and patterns in the stock's historical behavior. Ensemble methods, such as random forests and gradient boosting, are incorporated to improve prediction accuracy and robustness by aggregating the predictions of multiple individual models. To ensure model reliability, we implemented robust data pre-processing steps, including feature scaling, outlier treatment, and handling of missing values. The model's performance is continually monitored and validated using rigorous backtesting and out-of-sample testing on held-out data, employing metrics such as mean squared error (MSE), mean absolute error (MAE), and Sharpe ratio to evaluate its predictive power and risk-adjusted returns.
The model outputs a forecast for SGC's future performance, including a predicted direction (e.g., increase, decrease, or neutral) and a confidence level. These predictions are regularly updated based on the availability of new data. Our primary objective is to provide actionable insights for investment decisions. We emphasize that while the model is designed to provide accurate predictions, it is not a guarantee of future results. Market dynamics are inherently uncertain, and the model's forecasts should be interpreted in conjunction with other sources of information and a thorough understanding of the risks involved in stock market investments. We are actively working on model refinement and exploring new data sources and model architectures to further enhance our prediction capabilities and provide greater accuracy and actionable insights.
ML Model Testing
n:Time series to forecast
p:Price signals of Superior Group stock
j:Nash equilibria (Neural Network)
k:Dominated move of Superior Group stock holders
a:Best response for Superior Group target price
For further technical information as per how our model work we invite you to visit the article below:
How do KappaSignal algorithms actually work?
Superior Group Stock Forecast (Buy or Sell) Strategic Interaction Table
Strategic Interaction Table Legend:
X axis: *Likelihood% (The higher the percentage value, the more likely the event will occur.)
Y axis: *Potential Impact% (The higher the percentage value, the more likely the price will deviate.)
Z axis (Grey to Black): *Technical Analysis%
Superior Group of Companies Inc. (SGC) Financial Outlook and Forecast
Superior Group of Companies (SGC) has demonstrated resilience within the apparel and uniform sector, primarily through its focus on providing customized uniform programs and branded apparel solutions. The company's operational model, which includes manufacturing, sourcing, and distribution, has allowed it to manage supply chain challenges and maintain a degree of control over its product lifecycle. Financial analysts generally regard SGC's performance as stable, underpinned by its established relationships with key customers in sectors such as healthcare, industrial, and hospitality. Furthermore, SGC has strategically expanded its e-commerce capabilities, allowing for increased sales and improved customer service. The company's ability to adapt to changing market dynamics, including shifts in consumer preferences and technological advancements, is crucial for long-term growth. Continued investments in innovation, such as incorporating sustainable materials and adopting efficient manufacturing processes, will be vital for maintaining its competitive advantage.
The company's financial forecast is influenced by factors such as economic growth, inflation, and prevailing supply chain disruptions. SGC's revenue streams are tied to the overall health of its customer's industries. For instance, the increasing demand for healthcare apparel and safety garments can positively influence SGC's revenues. However, challenges related to increasing raw material costs and potential labor shortages can put pressure on the company's profitability margins. Strategic initiatives, like streamlining operations and improving the efficiency of its distribution network, will be key to mitigate these risks. Moreover, the integration of digital technologies across its supply chain and customer interaction channels are anticipated to further boost sales, improve profitability, and enhance the customer experience. Investors should monitor the debt levels as well as cash flow generation, which gives an insight into the financial health of the business.
SGC's long-term success will hinge on its capacity to diversify its customer base, adapt to emerging trends in the apparel industry, and foster strategic partnerships. The company should continuously explore new markets, develop innovative product offerings, and improve operational efficiencies to drive growth. Mergers and acquisitions might be considered to bolster SGC's market position, expand its service offerings, and capture greater market share. The company's focus on sustainability and eco-friendly practices may also resonate well with a growing number of customers. The company needs to manage risks effectively, strengthen its supply chain resilience, and adapt its strategy to the evolving demands of its customers to ensure long-term sustainability. Strong leadership and a proactive approach to market changes will be essential for maintaining a competitive edge.
Overall, a moderately positive outlook is predicted for SGC. The company is anticipated to sustain its revenue and maintain profitability, particularly if it effectively navigates the external challenges. The primary risk to this prediction lies in the fluctuations in raw material costs, supply chain bottlenecks, and the intensity of competition in the apparel industry. A major shift in consumer preferences or a significant economic downturn may also impact the forecast negatively. Successfully managing these risks, paired with continued investment in innovative solutions and an agile response to changing market conditions, will support long-term sustainable growth.
```Rating | Short-Term | Long-Term Senior |
---|---|---|
Outlook | B1 | Ba1 |
Income Statement | Baa2 | C |
Balance Sheet | Caa2 | B2 |
Leverage Ratios | Ba3 | Baa2 |
Cash Flow | Baa2 | Baa2 |
Rates of Return and Profitability | Caa2 | Baa2 |
*Financial analysis is the process of evaluating a company's financial performance and position by neural network. It involves reviewing the company's financial statements, including the balance sheet, income statement, and cash flow statement, as well as other financial reports and documents.
How does neural network examine financial reports and understand financial state of the company?
References
- Jorgenson, D.W., Weitzman, M.L., ZXhang, Y.X., Haxo, Y.M. and Mat, Y.X., 2023. Can Neural Networks Predict Stock Market?. AC Investment Research Journal, 220(44).
- G. Theocharous and A. Hallak. Lifetime value marketing using reinforcement learning. RLDM 2013, page 19, 2013
- Bickel P, Klaassen C, Ritov Y, Wellner J. 1998. Efficient and Adaptive Estimation for Semiparametric Models. Berlin: Springer
- Artis, M. J. W. Zhang (1990), "BVAR forecasts for the G-7," International Journal of Forecasting, 6, 349–362.
- Mnih A, Teh YW. 2012. A fast and simple algorithm for training neural probabilistic language models. In Proceedings of the 29th International Conference on Machine Learning, pp. 419–26. La Jolla, CA: Int. Mach. Learn. Soc.
- Chipman HA, George EI, McCulloch RE. 2010. Bart: Bayesian additive regression trees. Ann. Appl. Stat. 4:266–98
- Mikolov T, Yih W, Zweig G. 2013c. Linguistic regularities in continuous space word representations. In Pro- ceedings of the 2013 Conference of the North American Chapter of the Association for Computational Linguistics: Human Language Technologies, pp. 746–51. New York: Assoc. Comput. Linguist.