AUC Score :
Short-term Tactic1 :
Dominant Strategy :
Time series to forecast n:
ML Model Testing : Modular Neural Network (News Feed Sentiment Analysis)
Hypothesis Testing : Factor
Surveillance : Major exchange and OTC
1Short-term revised.
2Time series is updated based on short-term trends.
Key Points
GRC is projected to experience moderate growth due to its established gold production and ongoing exploration efforts, potentially leading to increased revenue. The company's financial health is expected to remain relatively stable, supported by existing cash flow. However, GRC faces risks including fluctuating gold prices, which could significantly impact profitability. Political and regulatory changes in the regions where it operates pose another potential risk, as do unforeseen operational disruptions at its mines, all of which could negatively affect production output and overall performance. The company is also vulnerable to market sentiment shifts affecting investor confidence and share valuation.About Gold Resource Corporation
Gold Resource Corporation (GORO) is a precious metals producer focused on the exploration and development of gold and silver properties in North America. The company's primary operations are centered in Oaxaca, Mexico, where they operate the Don David Gold Mine. GORO employs a low-cost, high-margin production model by utilizing underground mining methods and focusing on efficient processing techniques. They aim to generate strong cash flows and provide shareholder returns.
GORO emphasizes responsible mining practices, including environmental stewardship and community engagement. Their strategy involves building a portfolio of high-quality gold and silver assets, with a focus on operating in politically stable jurisdictions. The company actively explores opportunities for organic growth through exploration on its existing properties and evaluating strategic acquisitions to expand its production profile and mineral reserves.
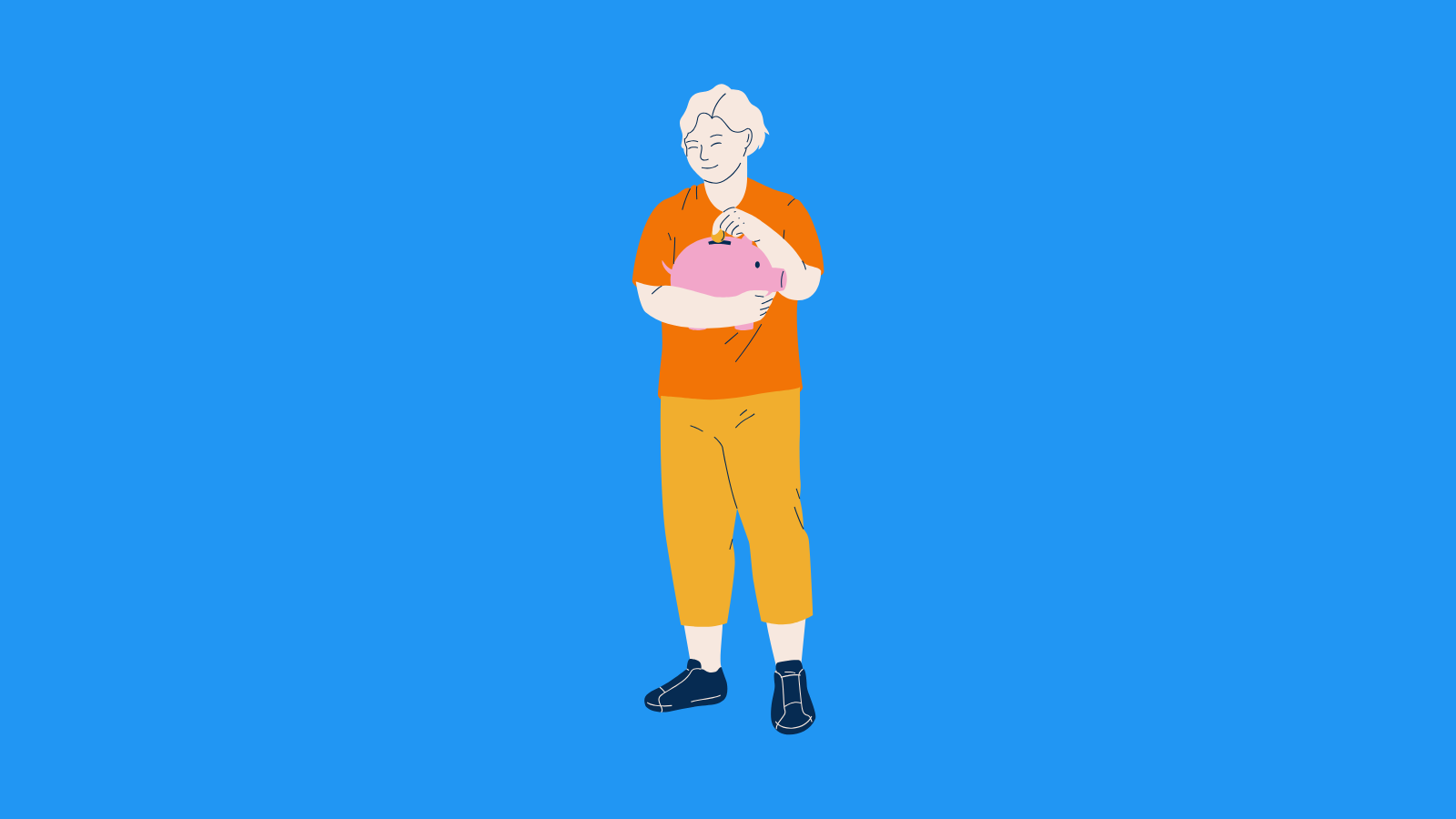
GORO Stock Prediction Model
Our team of data scientists and economists has developed a machine learning model to forecast the future performance of Gold Resource Corporation Common Stock (GORO). This model integrates a diverse range of financial and economic indicators to provide a comprehensive and data-driven prediction. We employed a combination of advanced algorithms, including Recurrent Neural Networks (RNNs) and Support Vector Machines (SVMs), known for their proficiency in handling time-series data and capturing complex relationships. The model's input features include historical trading data (e.g., volume, volatility), macroeconomic variables such as inflation rates and GDP growth, industry-specific factors like gold price fluctuations and mining production, and company-specific data encompassing financial statements, news sentiment analysis, and management guidance. Data preprocessing steps involved cleaning, transformation, and feature engineering to enhance model accuracy and reliability. Cross-validation techniques are employed to ensure robust model generalization, preventing overfitting and enhancing out-of-sample predictive power.
The model's architecture and training process are meticulously designed. We leverage a two-stage process: feature engineering, where relevant variables are extracted and transformed, followed by model training. The RNNs are particularly useful for uncovering temporal dependencies, while SVMs capture complex, nonlinear interactions among the features. The model's performance is assessed through a suite of metrics, including root mean squared error (RMSE), mean absolute error (MAE), and the Sharpe ratio. To validate the model's robustness, we incorporate backtesting on historical data, stress-testing under various economic scenarios, and regular re-evaluation to ensure its continued accuracy and reliability. Furthermore, the model is designed to adapt to changing market conditions, with automated retraining scheduled at regular intervals, ensuring that the model remains current and relevant.
The output of our machine learning model is a probability distribution representing the likelihood of GORO's stock performance. This information can be used to inform investment decisions and portfolio management strategies. We emphasize that the model provides probabilistic forecasts, reflecting the inherent uncertainty in financial markets. The model is not a guarantee of future outcomes but rather a sophisticated tool to augment the investment decision-making process. We understand that this is only a tool, and it's important to consider the model's predictions in conjunction with fundamental analysis, risk assessment, and investment objectives. Furthermore, the model's outputs are subject to continuous refinement and improvement through ongoing monitoring, feedback, and the integration of new data sources and algorithms to enhance overall accuracy and effectiveness.
ML Model Testing
n:Time series to forecast
p:Price signals of Gold Resource Corporation stock
j:Nash equilibria (Neural Network)
k:Dominated move of Gold Resource Corporation stock holders
a:Best response for Gold Resource Corporation target price
For further technical information as per how our model work we invite you to visit the article below:
How do KappaSignal algorithms actually work?
Gold Resource Corporation Stock Forecast (Buy or Sell) Strategic Interaction Table
Strategic Interaction Table Legend:
X axis: *Likelihood% (The higher the percentage value, the more likely the event will occur.)
Y axis: *Potential Impact% (The higher the percentage value, the more likely the price will deviate.)
Z axis (Grey to Black): *Technical Analysis%
Gold Resource Corporation (GORO) Financial Outlook and Forecast
GORO, a gold and silver producer focused on the Americas, presents a mixed financial outlook. The company's performance is intricately tied to the prevailing precious metals prices, its operational efficiency at its producing mines, and the successful execution of its exploration and development strategies. Recent financial results have shown volatility, reflecting fluctuating gold and silver prices and sometimes constrained production volumes. Investors should pay close attention to the company's cost management, especially all-in sustaining costs (AISC), which is a crucial metric for profitability. GORO has typically been operating with a focus on smaller, high-grade deposits, which offer the potential for robust margins when metal prices are favorable. However, these operations may be vulnerable to unforeseen geological challenges or operational disruptions. Strong cash flow generation is vital for supporting exploration, debt management, and shareholder returns, particularly in the form of dividends.
The forecast for GORO is cautiously optimistic, dependent on several key factors. The price of gold and silver remains the most significant external determinant of the company's financial prospects. Positive developments include the potential for geopolitical instability to buoy demand for precious metals as a safe-haven asset. GORO's production profile is projected to be relatively stable; this will depend on the successful integration of the company's existing assets. Furthermore, if the company can discover additional high-grade resources through successful exploration, this could significantly enhance its long-term value. GORO's capacity to leverage its existing infrastructure to optimize production across its operations is important. Effective cost control and disciplined capital allocation are essential for maximizing profitability and providing shareholder value.
GORO's strategic initiatives will play an essential role in shaping its future performance. The ability to effectively manage its current asset portfolio is critical. The firm's focus on exploration and development projects holds the potential for future growth. The company's ability to successfully navigate regulatory hurdles and obtain necessary permits for new projects is important. Strategic partnerships and joint ventures could provide access to additional resources and expertise. Focus on environmental, social, and governance (ESG) factors is increasingly important to attract investment and maintain a positive reputation within the industry. Investors should closely monitor management's execution of its strategic plan, as well as how it navigates the volatility inherent in the precious metals sector.
The prediction for GORO is positive, but with risks. The company has the potential to generate consistent returns if gold and silver prices remain strong, production continues at a stable rate, and costs are managed effectively. GORO's focus on high-grade deposits and its emphasis on exploration for new resources provide further upside potential. However, this positive outlook is tempered by several key risks. A significant decrease in precious metal prices would severely impact the company's profitability and cash flow. Operational disruptions, such as unforeseen geological challenges or equipment failures, could curtail production and increase costs. Changes in government regulations and increased taxes in the areas where GORO operates could impact its financial stability. A proactive approach to managing these risks will be critical for GORO's long-term success.
Rating | Short-Term | Long-Term Senior |
---|---|---|
Outlook | B2 | Ba3 |
Income Statement | Caa2 | Baa2 |
Balance Sheet | Baa2 | C |
Leverage Ratios | B3 | Baa2 |
Cash Flow | Ba1 | Caa2 |
Rates of Return and Profitability | C | Baa2 |
*Financial analysis is the process of evaluating a company's financial performance and position by neural network. It involves reviewing the company's financial statements, including the balance sheet, income statement, and cash flow statement, as well as other financial reports and documents.
How does neural network examine financial reports and understand financial state of the company?
References
- L. Prashanth and M. Ghavamzadeh. Actor-critic algorithms for risk-sensitive MDPs. In Proceedings of Advances in Neural Information Processing Systems 26, pages 252–260, 2013.
- Wager S, Athey S. 2017. Estimation and inference of heterogeneous treatment effects using random forests. J. Am. Stat. Assoc. 113:1228–42
- Bottou L. 2012. Stochastic gradient descent tricks. In Neural Networks: Tricks of the Trade, ed. G Montavon, G Orr, K-R Müller, pp. 421–36. Berlin: Springer
- Krizhevsky A, Sutskever I, Hinton GE. 2012. Imagenet classification with deep convolutional neural networks. In Advances in Neural Information Processing Systems, Vol. 25, ed. Z Ghahramani, M Welling, C Cortes, ND Lawrence, KQ Weinberger, pp. 1097–105. San Diego, CA: Neural Inf. Process. Syst. Found.
- Holland PW. 1986. Statistics and causal inference. J. Am. Stat. Assoc. 81:945–60
- Friedberg R, Tibshirani J, Athey S, Wager S. 2018. Local linear forests. arXiv:1807.11408 [stat.ML]
- Bell RM, Koren Y. 2007. Lessons from the Netflix prize challenge. ACM SIGKDD Explor. Newsl. 9:75–79