AUC Score :
Short-term Tactic1 :
Dominant Strategy :
Time series to forecast n:
ML Model Testing : Multi-Instance Learning (ML)
Hypothesis Testing : Polynomial Regression
Surveillance : Major exchange and OTC
1Short-term revised.
2Time series is updated based on short-term trends.
Key Points
The TR/CC CRB ex Energy TR index is anticipated to experience moderate volatility in the near term. A slight upward trend is expected, driven by potential strengthening in industrial metals and agricultural commodities, offset somewhat by uncertainties in precious metals and softer demand. Risks to this outlook include unexpected global economic slowdown, heightened geopolitical tensions impacting supply chains, and significant shifts in currency values. These factors could result in a downward correction, negating the predicted gains, potentially impacting investor confidence in the index. Furthermore, unexpected weather events or crop failures in agricultural markets could substantially destabilize the index.About TR/CC CRB ex Energy TR Index
The TR/CC CRB ex Energy TR index, maintained by Refinitiv, is a commodity futures price index designed to reflect the overall trends in the global commodity markets, excluding the energy sector. The index is a modified version of the Thomson Reuters/CoreCommodity CRB (CRB) Index, widely recognized as a benchmark for commodity market performance. It provides investors with exposure to a diversified basket of commodity futures contracts, encompassing a broad range of sectors such as agriculture, precious metals, industrial metals, and livestock.
This particular index isolates the non-energy commodity components of the CRB, making it a useful tool for analyzing and tracking the performance of these specific commodity sectors. The weighting of the index components is typically based on trading volume and liquidity within the underlying futures markets. This weighting methodology aims to ensure that the index represents a liquid and tradable portfolio that allows investors to gain diversified commodity exposure without the volatility often associated with energy markets. The exclusion of energy provides a clearer view of the price behavior in other commodity sectors.
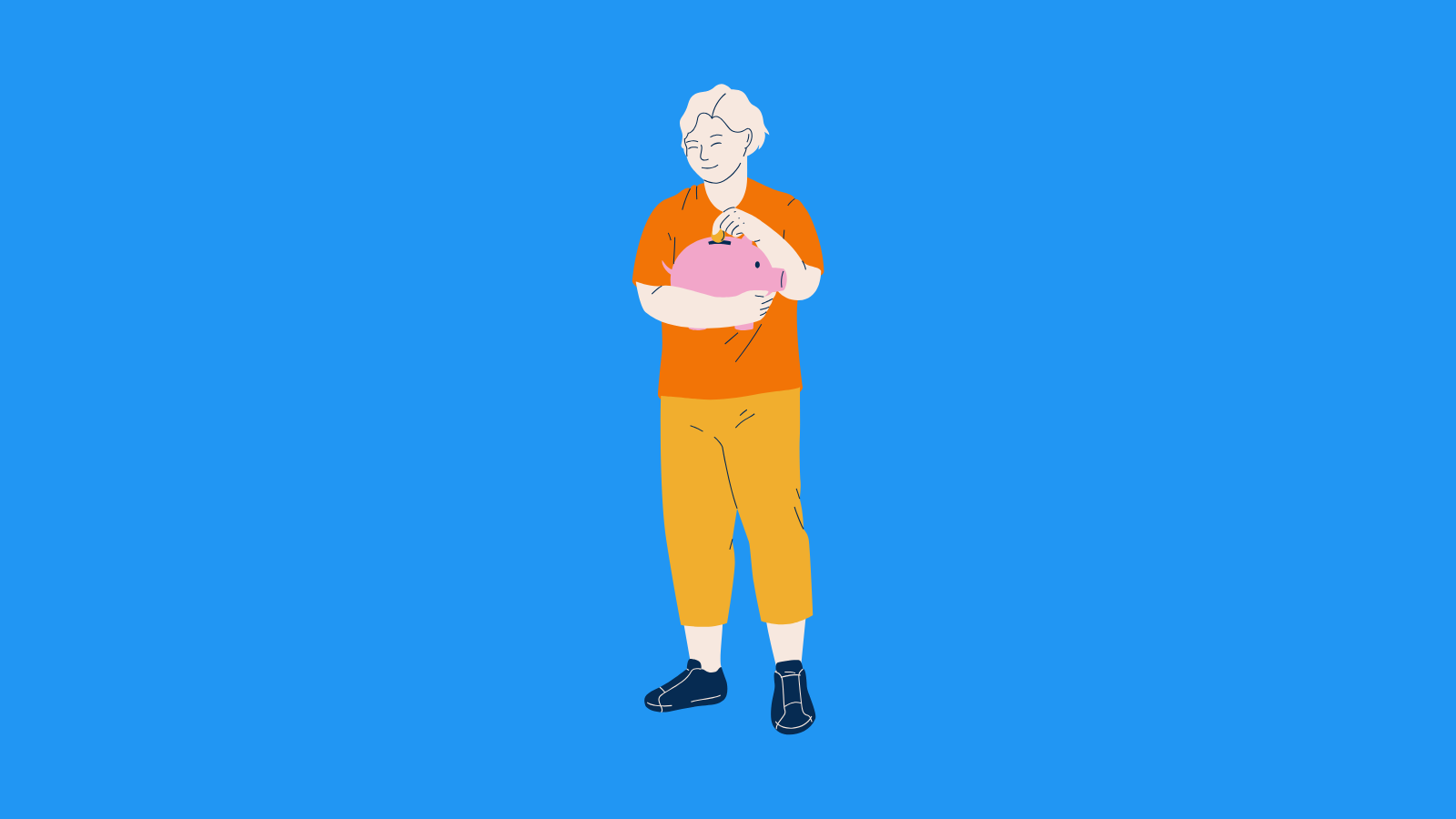
TR/CC CRB ex Energy TR Index Forecast Machine Learning Model
Our team, composed of data scientists and economists, has developed a machine learning model to forecast the TR/CC CRB ex Energy TR index. The model leverages a diverse set of macroeconomic and market-specific features. Macroeconomic indicators such as inflation rates, industrial production indices, and global GDP growth are incorporated to capture the broader economic environment influencing commodity demand. Furthermore, we integrate financial market data, including interest rate differentials, currency exchange rates, and equity market performance, as these factors often correlate with commodity price movements. Crucially, we also include lagged values of the TR/CC CRB ex Energy TR index itself to account for inherent persistence in commodity price trends and potential momentum effects. The data undergo rigorous preprocessing, including cleaning, imputation of missing values, and standardization, to ensure data quality and model stability.
We explored various machine learning algorithms, including Random Forest, Gradient Boosting, and Recurrent Neural Networks (RNNs), specifically Long Short-Term Memory (LSTM) networks. Model selection was based on rigorous evaluation, utilizing time series cross-validation to assess predictive accuracy and robustness across different historical periods. Performance metrics, such as Mean Absolute Error (MAE), Root Mean Squared Error (RMSE), and the Directional Accuracy, were employed to gauge the model's predictive power. Hyperparameter tuning was conducted using techniques like grid search and cross-validation to optimize model performance. The optimal model configuration, incorporating a carefully selected set of features and the best-performing algorithm, was chosen for forecasting the TR/CC CRB ex Energy TR index. Model interpretability is prioritized by analyzing feature importance and understanding the influence of different input variables on the forecast.
The final model provides forecasts for the TR/CC CRB ex Energy TR index, offering insights into potential future price movements. These forecasts are subject to inherent uncertainties associated with commodity markets, and we emphasize the need for caution when interpreting the results. The model's predictions are continuously monitored and validated against actual market data to refine its performance and incorporate any changes in market dynamics. Furthermore, we plan to periodically update the model with new data and refine its parameters based on emerging insights. We also integrate model risk management as part of the development strategy to understand the potential risks of model predictions.
ML Model Testing
n:Time series to forecast
p:Price signals of TR/CC CRB ex Energy TR index
j:Nash equilibria (Neural Network)
k:Dominated move of TR/CC CRB ex Energy TR index holders
a:Best response for TR/CC CRB ex Energy TR target price
For further technical information as per how our model work we invite you to visit the article below:
How do KappaSignal algorithms actually work?
TR/CC CRB ex Energy TR Index Forecast Strategic Interaction Table
Strategic Interaction Table Legend:
X axis: *Likelihood% (The higher the percentage value, the more likely the event will occur.)
Y axis: *Potential Impact% (The higher the percentage value, the more likely the price will deviate.)
Z axis (Grey to Black): *Technical Analysis%
TR/CC CRB ex Energy TR Index: Financial Outlook and Forecast
The Thomson Reuters/CoreCommodity CRB ex Energy Total Return (TR) Index, a leading benchmark for commodity markets, presents a mixed outlook characterized by underlying strengths and significant vulnerabilities. The index, excluding energy components, offers a perspective on the performance of a diversified basket of non-energy commodities, providing insights into global economic activity, inflation expectations, and supply-demand dynamics across various sectors. Its financial outlook is intricately tied to these factors, influencing its trajectory and potential for value appreciation. Analysis suggests that industrial metals, agricultural products, and precious metals will have substantial weight in the index's performance. The index's value is also impacted by the strength of the U.S. dollar, which often has an inverse relationship with commodity prices. Moreover, the global economic landscape, encompassing manufacturing output, construction activities, and infrastructure development, critically affects demand and, consequently, the index's performance. Geopolitical events, such as conflicts or trade disputes, are critical factors and can cause sudden shifts in supply chains and price volatility.
The forecast for the TR/CC CRB ex Energy TR Index will largely depend on macroeconomic indicators and sectoral trends. Stronger-than-expected global growth, particularly in emerging markets, would likely fuel demand for industrial metals and agricultural commodities, supporting the index's positive development. Conversely, an economic slowdown or recession could dampen demand, leading to price corrections. Factors such as supply chain disruptions, geopolitical tensions, and climate-related events can severely affect the agricultural sector, potentially causing price spikes. The performance of precious metals will be highly correlated to investor sentiment and risk appetite, with safe-haven demand driving prices higher during economic uncertainty. Supply-side dynamics, including production levels, inventories, and emerging technologies, will play a critical role in shaping commodity prices. Technological advancements in areas such as agricultural yield enhancement and mineral extraction may also impact commodity pricing and overall market volatility.
Several indicators will be crucial in assessing the index's financial trajectory. Monitoring manufacturing purchasing managers' indexes (PMIs), which provide insights into industrial production levels, is paramount. Changes in global construction activities, reflected in housing starts and infrastructure spending, are also vital, particularly for industrial metals. Furthermore, agricultural production reports and weather patterns, impacting yields, are important for the agricultural sector. Inflation data and central bank policies, including interest rate decisions, heavily affect investor sentiment and commodity values. Analyzing supply-demand balances across various commodities, including inventories and production forecasts, is essential. Monitoring the U.S. dollar's exchange rate and global trade dynamics, including any shifts in trade policies, will have important roles in the index's performance. Considering the aforementioned indicators will provide a comprehensive understanding of the prevailing market conditions, guiding investors' strategic decisions.
Overall, the outlook for the TR/CC CRB ex Energy TR Index appears cautiously optimistic. The prediction is positive, assuming sustained, yet moderate, global economic growth, limited supply-chain disruptions, and relatively stable geopolitical environment. Demand from emerging markets, coupled with infrastructure spending, is expected to support commodity prices, leading to modest index growth. However, there are substantial risks to this forecast. Any escalation in geopolitical tensions, significant economic slowdowns, or major disruptions in key commodity-producing regions could trigger substantial price corrections. Moreover, the rapid evolution of alternative energy sources and technological advancements may affect demand for certain commodities. Climate change related events leading to production shortfalls across various agricultural commodities could also negatively impact the index. The index's sensitivity to various macroeconomic and geopolitical events necessitates continuous monitoring and diversification to mitigate potential investment risks.
Rating | Short-Term | Long-Term Senior |
---|---|---|
Outlook | Ba1 | Ba3 |
Income Statement | Baa2 | Ba3 |
Balance Sheet | B3 | Baa2 |
Leverage Ratios | Baa2 | C |
Cash Flow | Baa2 | Baa2 |
Rates of Return and Profitability | B2 | Caa2 |
*An aggregate rating for an index summarizes the overall sentiment towards the companies it includes. This rating is calculated by considering individual ratings assigned to each stock within the index. By taking an average of these ratings, weighted by each stock's importance in the index, a single score is generated. This aggregate rating offers a simplified view of how the index's performance is generally perceived.
How does neural network examine financial reports and understand financial state of the company?
References
- Challen, D. W. A. J. Hagger (1983), Macroeconomic Systems: Construction, Validation and Applications. New York: St. Martin's Press.
- L. Panait and S. Luke. Cooperative multi-agent learning: The state of the art. Autonomous Agents and Multi-Agent Systems, 11(3):387–434, 2005.
- Hoerl AE, Kennard RW. 1970. Ridge regression: biased estimation for nonorthogonal problems. Technometrics 12:55–67
- Miller A. 2002. Subset Selection in Regression. New York: CRC Press
- Bewley, R. M. Yang (1998), "On the size and power of system tests for cointegration," Review of Economics and Statistics, 80, 675–679.
- Li L, Chu W, Langford J, Moon T, Wang X. 2012. An unbiased offline evaluation of contextual bandit algo- rithms with generalized linear models. In Proceedings of 4th ACM International Conference on Web Search and Data Mining, pp. 297–306. New York: ACM
- Chernozhukov V, Demirer M, Duflo E, Fernandez-Val I. 2018b. Generic machine learning inference on heteroge- nous treatment effects in randomized experiments. NBER Work. Pap. 24678