AUC Score :
Short-term Tactic1 :
Dominant Strategy :
Time series to forecast n:
ML Model Testing : Ensemble Learning (ML)
Hypothesis Testing : Wilcoxon Sign-Rank Test
Surveillance : Major exchange and OTC
1Short-term revised.
2Time series is updated based on short-term trends.
Key Points
Ardent's stock is projected to experience moderate growth, fueled by increasing demand for healthcare services and strategic acquisitions. However, this growth faces risks including fluctuations in healthcare reimbursement rates, potential impacts from changes in healthcare regulations, and the possibility of increased operating costs. Competition from other healthcare providers also poses a challenge. Furthermore, any failure to effectively integrate acquired entities or manage debt levels could negatively affect financial performance and stock valuation, introducing volatility and uncertainty.About Ardent Health Partners
Ardent Health, a leading healthcare provider, operates a network of hospitals and clinics across the United States. The company focuses on delivering high-quality, patient-centered care, encompassing a broad range of medical services. Their business model involves owning and managing acute care hospitals, ambulatory surgery centers, and physician practices, creating an integrated healthcare delivery system. Ardent Health emphasizes operational efficiency and strategic growth, constantly seeking opportunities to expand its footprint and enhance its service offerings within the healthcare sector. They aim to improve patient outcomes while navigating the evolving landscape of healthcare regulations and technological advancements.
Ardent Health's strategy includes acquiring and developing healthcare facilities in various markets, enhancing their capabilities through strategic partnerships. The company strives to create a positive work environment for its medical professionals. They provide essential medical services, focusing on areas such as cardiology, oncology, orthopedics, and women's health, and catering to diverse patient populations. Ardent Health aims to be a key player in the healthcare industry by prioritizing patient satisfaction, operational excellence, and adapting to the healthcare industry trends.
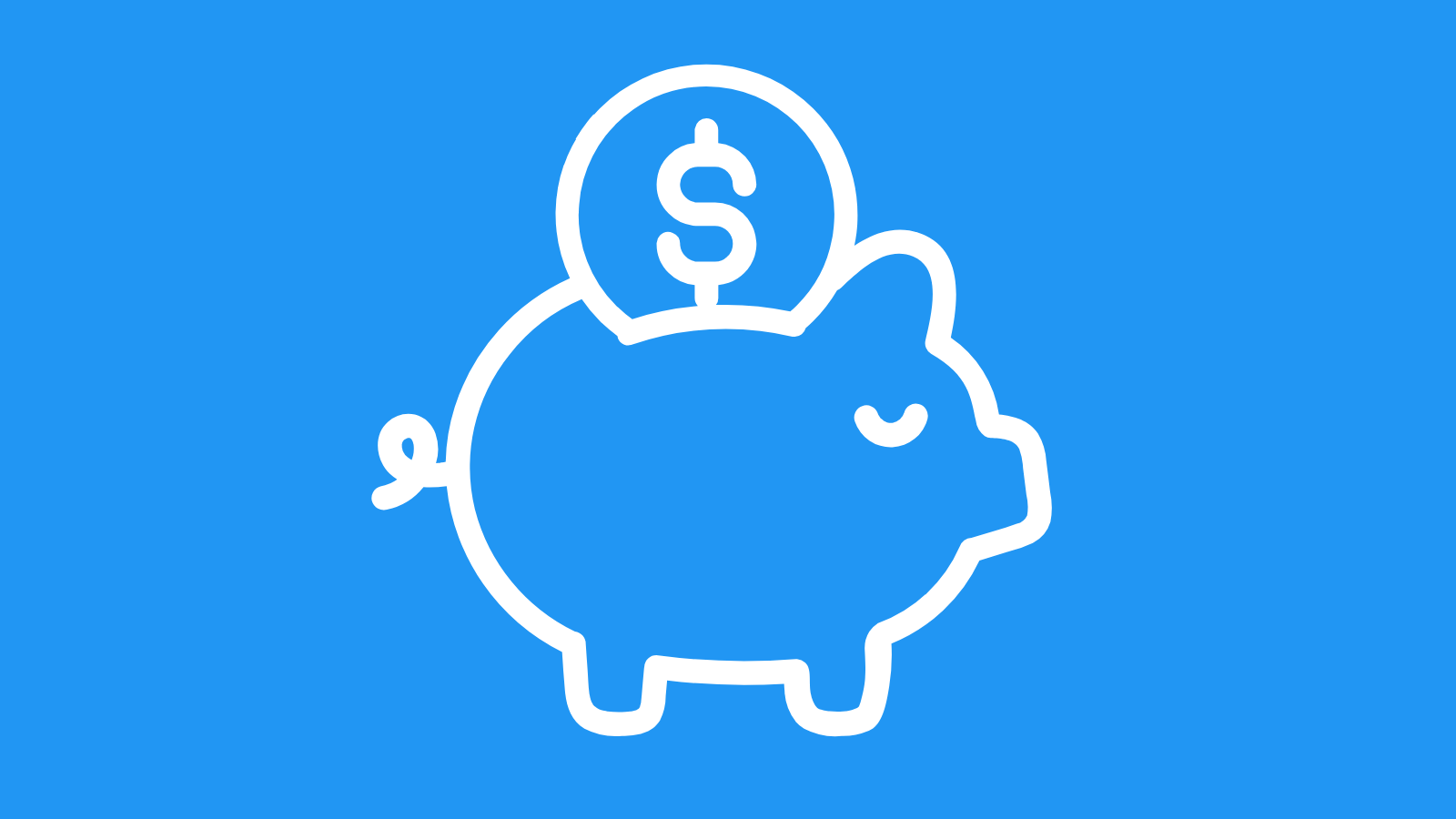
Machine Learning Model for ARDT Stock Forecast
Our interdisciplinary team, composed of data scientists and economists, has developed a machine learning model to forecast the performance of Ardent Health Partners Inc. (ARDT) common stock. The model leverages a diverse dataset, encompassing both internal and external factors. This includes historical financial statements from ARDT (revenue, expenses, profitability margins), market capitalization, and trading volume, as well as macroeconomic indicators (GDP growth, inflation rates, interest rates) and industry-specific data (healthcare expenditure, competitor performance, regulatory changes). The model is designed with a multi-faceted approach, allowing for different machine learning techniques. Algorithms such as recurrent neural networks (specifically LSTM for time-series data), gradient boosting methods (like XGBoost and LightGBM), and potentially a hybrid approach combining several model types, will be used to capture different characteristics. We will refine the architecture and hyperparameters, in this way, maximizing the model's predictive accuracy.
The modeling process involves several key stages. First, data will be rigorously cleaned and preprocessed to handle missing values, outliers, and ensure data consistency. Feature engineering will be a core activity, creating new variables that capture relationships and transformations of the input data. For example, we'll build momentum indicators based on ARDT's trading volume and moving averages of the key metrics. Then, the dataset is split into training, validation, and testing sets. The model will be trained on the training data, and validated on the validation data to tune hyperparameters. Finally, the model's performance will be evaluated on the held-out testing set. Key performance indicators will be the mean absolute error (MAE), the root mean squared error (RMSE), and the R-squared score. Model evaluation will incorporate backtesting and sensitivity analysis.
To address the dynamic nature of financial markets, the model will be periodically retrained with the most recent data. We will implement a model monitoring system to constantly assess the model's performance and identify any potential degradation in accuracy. A feedback loop will allow us to incorporate new features, refine algorithms, and adapt to changing market dynamics. Further, we will integrate a risk management component to incorporate the model's outputs into investment strategies. We will analyze the forecasts, their associated confidence intervals, and the potential impacts of market changes, using the model to help make informed and data-driven trading decisions. This will provide us with the best possible forecast and will minimize our risks.
ML Model Testing
n:Time series to forecast
p:Price signals of Ardent Health Partners stock
j:Nash equilibria (Neural Network)
k:Dominated move of Ardent Health Partners stock holders
a:Best response for Ardent Health Partners target price
For further technical information as per how our model work we invite you to visit the article below:
How do KappaSignal algorithms actually work?
Ardent Health Partners Stock Forecast (Buy or Sell) Strategic Interaction Table
Strategic Interaction Table Legend:
X axis: *Likelihood% (The higher the percentage value, the more likely the event will occur.)
Y axis: *Potential Impact% (The higher the percentage value, the more likely the price will deviate.)
Z axis (Grey to Black): *Technical Analysis%
Ardent Health Partners' Financial Outlook and Forecast
The financial outlook for Ardent Health Partners (AHP) appears promising, driven by a strategic focus on expanding its network of hospitals and outpatient facilities, particularly in high-growth markets. The company's strategy involves acquisitions, partnerships, and organic growth initiatives to increase its market share and diversify its revenue streams. This approach is further bolstered by the ongoing trend towards outpatient care, which aligns with AHP's investments in ambulatory services and surgical centers. Furthermore, AHP's management team has demonstrated a commitment to operational efficiency, aiming to streamline processes and control costs. This includes leveraging technology and data analytics to optimize patient care and improve financial performance. Such initiatives suggest a focus on building a resilient and adaptable business model capable of weathering economic fluctuations and healthcare industry shifts.
AHP's forecast reflects its expansion plans and operational improvements. Revenue growth is anticipated to be driven by increased patient volumes, especially in areas with growing populations and underserved healthcare needs. The company's investments in technology and infrastructure are expected to translate into enhanced operational efficiency, which in turn should improve its profitability margins. Strategic partnerships and acquisitions will likely play a key role in increasing its footprint and revenue diversification. In addition, the trend towards value-based care and managed care contracts suggests that AHP is strategically positioned to adapt to evolving reimbursement models. As the healthcare landscape continues to evolve, the company is expected to remain agile and flexible, adapting to changes in healthcare delivery and regulation.
Key factors influencing AHP's financial performance include its ability to successfully integrate acquired businesses and maintain high-quality patient care. The company's ability to navigate regulatory changes within the healthcare industry, including those related to reimbursement and compliance, is also crucial. Furthermore, economic factors, such as inflation and labor market conditions, will undoubtedly impact AHP's costs. Rising labor costs, in particular, pose a significant challenge for all healthcare providers. AHP's ability to manage these costs effectively will be a major factor in its profitability. The company's operational excellence and efficiency measures, coupled with its strategic expansion, will be crucial in improving its overall performance.
In conclusion, the forecast for AHP is positive, with expectations for continued revenue growth and improved profitability. This optimistic outlook is supported by its expansion strategy, investments in outpatient care, and commitment to operational excellence. However, this prediction does carry risks. One key risk involves integration challenges following acquisitions, which could lead to unforeseen costs or operational disruptions. Economic downturns, particularly those affecting healthcare demand and insurance coverage, pose another risk. Changes in government regulations, especially those related to reimbursement rates and healthcare access, may negatively affect financial results. The company's long-term success hinges on its ability to mitigate these risks while capitalizing on the opportunities presented by a changing healthcare landscape.
Rating | Short-Term | Long-Term Senior |
---|---|---|
Outlook | B2 | B1 |
Income Statement | C | Ba3 |
Balance Sheet | B1 | Caa2 |
Leverage Ratios | Baa2 | Ba3 |
Cash Flow | B2 | Baa2 |
Rates of Return and Profitability | B2 | B3 |
*Financial analysis is the process of evaluating a company's financial performance and position by neural network. It involves reviewing the company's financial statements, including the balance sheet, income statement, and cash flow statement, as well as other financial reports and documents.
How does neural network examine financial reports and understand financial state of the company?
References
- Breiman L. 2001b. Statistical modeling: the two cultures (with comments and a rejoinder by the author). Stat. Sci. 16:199–231
- N. B ̈auerle and A. Mundt. Dynamic mean-risk optimization in a binomial model. Mathematical Methods of Operations Research, 70(2):219–239, 2009.
- Varian HR. 2014. Big data: new tricks for econometrics. J. Econ. Perspect. 28:3–28
- J. Z. Leibo, V. Zambaldi, M. Lanctot, J. Marecki, and T. Graepel. Multi-agent Reinforcement Learning in Sequential Social Dilemmas. In Proceedings of the 16th International Conference on Autonomous Agents and Multiagent Systems (AAMAS 2017), Sao Paulo, Brazil, 2017
- Jorgenson, D.W., Weitzman, M.L., ZXhang, Y.X., Haxo, Y.M. and Mat, Y.X., 2023. Can Neural Networks Predict Stock Market?. AC Investment Research Journal, 220(44).
- Burkov A. 2019. The Hundred-Page Machine Learning Book. Quebec City, Can.: Andriy Burkov
- Z. Wang, T. Schaul, M. Hessel, H. van Hasselt, M. Lanctot, and N. de Freitas. Dueling network architectures for deep reinforcement learning. In Proceedings of the International Conference on Machine Learning (ICML), pages 1995–2003, 2016.