AUC Score :
Short-term Tactic1 :
Dominant Strategy :
Time series to forecast n:
ML Model Testing : Deductive Inference (ML)
Hypothesis Testing : Statistical Hypothesis Testing
Surveillance : Major exchange and OTC
1Short-term revised.
2Time series is updated based on short-term trends.
Key Points
The Dow Jones U.S. Semiconductors index is anticipated to experience a period of moderate growth, driven by continued demand for advanced computing and emerging technologies. Increased adoption of artificial intelligence, cloud computing, and electric vehicles will fuel expansion within the sector, fostering innovation and driving revenue. However, there's a risk of slowing growth due to potential supply chain disruptions, geopolitical tensions impacting global trade, and the cyclical nature of the semiconductor industry, which could lead to periods of price volatility. Furthermore, rapid technological advancements could render some existing technologies obsolete, impacting companies' profitability and market share.About Dow Jones U.S. Semiconductors Index
The Dow Jones U.S. Semiconductors Index is a market capitalization-weighted index designed to track the performance of companies involved in the design, manufacture, and sale of semiconductors and related equipment. It includes a selection of U.S.-listed companies that derive a significant portion of their revenue from the semiconductor industry. The index serves as a benchmark for investors seeking exposure to the semiconductor sector, allowing them to gauge the overall health and performance of this crucial technology area. The index is frequently used as a tool for portfolio construction, performance evaluation, and the creation of financial products such as exchange-traded funds (ETFs).
The semiconductor industry is known for its cyclical nature, driven by technological advancements, global demand, and supply chain dynamics. The Dow Jones U.S. Semiconductors Index reflects these cyclical trends. It is rebalanced periodically to maintain a representative composition of the sector, ensuring that it accurately reflects the current landscape of the semiconductor market. Investors utilize the index to monitor the industry's progress, assess market trends, and make informed investment decisions regarding the dynamic and rapidly evolving semiconductor sector.
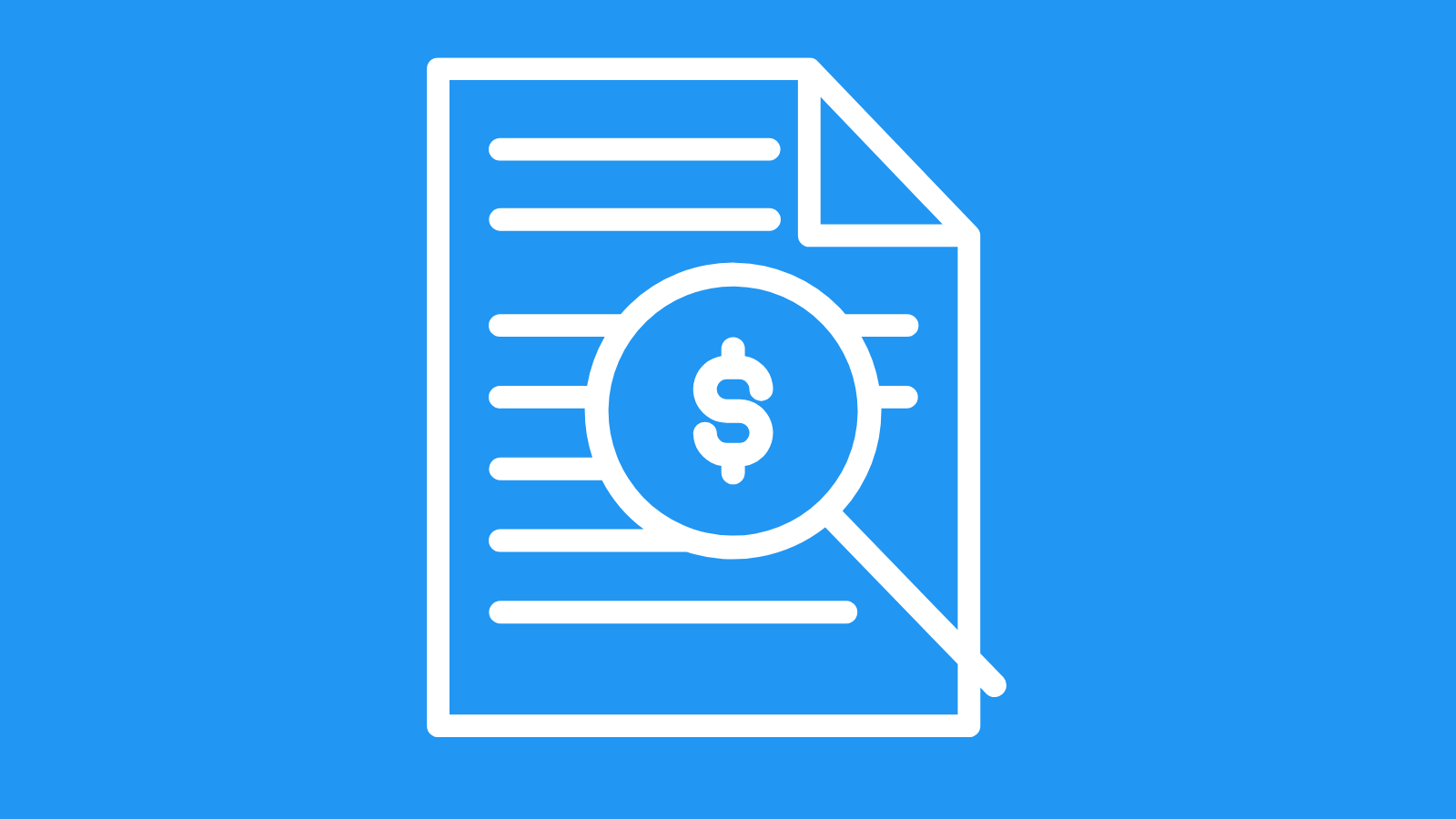
Machine Learning Model for Dow Jones U.S. Semiconductors Index Forecast
Our team of data scientists and economists proposes a comprehensive machine learning model to forecast the Dow Jones U.S. Semiconductors Index. The foundation of this model rests on a robust feature engineering process, leveraging both technical and fundamental indicators. Technical indicators will encompass moving averages (simple, exponential), Relative Strength Index (RSI), Moving Average Convergence Divergence (MACD), and Volume-weighted Average Price (VWAP) to capture market sentiment and trading dynamics. Simultaneously, we will integrate fundamental indicators, including quarterly earnings reports, revenue growth, price-to-earnings ratios (P/E), debt-to-equity ratios, and industry-specific factors like semiconductor sales and inventory levels. Furthermore, we will incorporate macroeconomic variables like interest rates, inflation rates, GDP growth, and unemployment rates, recognizing their significant influence on market performance. Data from various sources, including financial news providers, regulatory filings, and economic databases, will be meticulously curated and preprocessed to ensure data quality and consistency.
For the core predictive model, we will employ a hybrid approach, combining the strengths of different machine learning algorithms. Initially, we will explore Recurrent Neural Networks (RNNs), particularly Long Short-Term Memory (LSTM) networks, which are well-suited for time-series data and capable of capturing long-term dependencies within the index's historical performance. Additionally, we plan to experiment with Gradient Boosting Machines (GBMs), such as XGBoost or LightGBM, known for their accuracy and robustness. An ensemble model, integrating the predictions of both RNNs and GBMs, will then be created to leverage the benefits of each algorithm. Hyperparameter tuning will be conducted using cross-validation techniques to optimize model performance and prevent overfitting. Rigorous evaluation metrics, including Mean Squared Error (MSE), Root Mean Squared Error (RMSE), and Mean Absolute Error (MAE), will be used to assess forecast accuracy. Furthermore, we will evaluate the model's ability to generate trading signals, gauging its potential for profitable investment strategies.
Model deployment will involve a real-time data pipeline to ingest new data and generate forecasts regularly. We will establish an automated system for data ingestion, preprocessing, and feature engineering to ensure timely predictions. The model's outputs, including predicted index values and potential trading signals, will be made available through a user-friendly interface, possibly integrated with existing financial platforms. Regular model retraining will be a crucial part of the process, ensuring that the model adapts to changing market conditions and maintains its predictive accuracy. We will monitor model performance, analyze forecast errors, and adjust model parameters or incorporate new data to improve performance over time. Regular model performance reports and backtesting results will be generated to track performance and inform further model refinements. We anticipate that this model will provide valuable insights into the Dow Jones U.S. Semiconductors Index, aiding investors, financial analysts, and portfolio managers in making informed investment decisions.
```
ML Model Testing
n:Time series to forecast
p:Price signals of Dow Jones U.S. Semiconductors index
j:Nash equilibria (Neural Network)
k:Dominated move of Dow Jones U.S. Semiconductors index holders
a:Best response for Dow Jones U.S. Semiconductors target price
For further technical information as per how our model work we invite you to visit the article below:
How do KappaSignal algorithms actually work?
Dow Jones U.S. Semiconductors Index Forecast Strategic Interaction Table
Strategic Interaction Table Legend:
X axis: *Likelihood% (The higher the percentage value, the more likely the event will occur.)
Y axis: *Potential Impact% (The higher the percentage value, the more likely the price will deviate.)
Z axis (Grey to Black): *Technical Analysis%
Dow Jones U.S. Semiconductors Index: Financial Outlook and Forecast
The Dow Jones U.S. Semiconductors Index, representing a significant segment of the technology sector, faces a complex and evolving financial outlook. Several key factors are currently shaping its trajectory. Firstly, the global demand for semiconductors remains substantial, fueled by the ongoing digitalization across various industries, including artificial intelligence, cloud computing, automotive, and consumer electronics. This pervasive need underpins a relatively strong base for the index. Secondly, supply chain dynamics continue to be a crucial consideration. While some bottlenecks have eased since the peak of the pandemic, geopolitical tensions and regional manufacturing concentrations create vulnerabilities. The index's performance is therefore sensitive to disruptions, such as trade restrictions or natural disasters, that could impact production and distribution. Thirdly, the index is inherently cyclical, meaning its performance is tied to broader economic cycles, which include consumer spending habits, capital expenditure, and overall business confidence. Therefore, any downturn in these economic indicators has the potential to negatively affect the performance of the index.
Specific industry trends significantly impact the outlook. The expansion of 5G technology and the Internet of Things (IoT) are driving demand for advanced semiconductors used in communications infrastructure and connected devices. The automotive industry's shift towards electric vehicles (EVs) is also a powerful growth driver, requiring increasingly sophisticated and powerful chips for propulsion systems, infotainment, and autonomous driving features. Investment in data centers and cloud infrastructure is another positive catalyst, demanding higher-performance processors and memory chips. However, the sector is characterized by high capital expenditures, long product development cycles, and rapid technological advancements. Companies must continuously invest in research and development to stay competitive. Competition is fierce among major players, and market share dynamics can shift quickly. Pricing pressure is also common, particularly in more mature product categories.
Government policies and macroeconomic trends also contribute to the outlook. Initiatives such as the U.S. CHIPS and Science Act and similar programs in other countries are designed to boost domestic semiconductor manufacturing and innovation, which could enhance long-term growth prospects for companies in the index. Inflation and rising interest rates, however, present risks by increasing the cost of capital, which could impact companies' investments in capital expenditures, R&D, and affect the price sensitivity of consumers purchasing electronic devices. Currency fluctuations are also a factor, given the global nature of the industry. Additionally, geopolitical risks related to trade and intellectual property protection can influence the competitive landscape and, thus, the financial performance of companies within the index. The valuation of semiconductor stocks is often subject to significant volatility. Investor sentiment, including expectations about future earnings, can influence stock prices. The sector is also exposed to regulatory scrutiny, particularly related to antitrust concerns and data privacy regulations.
Considering these multifaceted factors, the outlook for the Dow Jones U.S. Semiconductors Index is cautiously optimistic. We predict moderate growth in the mid-term (1-3 years), driven by sustained demand for advanced computing and communication technologies. This will be supported by an easing of supply chain bottlenecks and government initiatives. However, the inherent cyclicality of the sector and global economic uncertainty present significant risks. Inflation, economic slowdowns, and geopolitical events could dampen demand and profitability. Technological disruptions, such as the emergence of new chip architectures, are another risk factor. Furthermore, any sudden shift in trade policies or intellectual property disputes could negatively impact companies' financial results. Investors should monitor these factors closely and understand that while long-term prospects remain bright, the index's performance will likely be subject to volatility and short-term fluctuations.
Rating | Short-Term | Long-Term Senior |
---|---|---|
Outlook | Baa2 | B2 |
Income Statement | Baa2 | C |
Balance Sheet | Baa2 | B2 |
Leverage Ratios | B2 | Ba3 |
Cash Flow | B2 | C |
Rates of Return and Profitability | Baa2 | Ba3 |
*An aggregate rating for an index summarizes the overall sentiment towards the companies it includes. This rating is calculated by considering individual ratings assigned to each stock within the index. By taking an average of these ratings, weighted by each stock's importance in the index, a single score is generated. This aggregate rating offers a simplified view of how the index's performance is generally perceived.
How does neural network examine financial reports and understand financial state of the company?
References
- Meinshausen N. 2007. Relaxed lasso. Comput. Stat. Data Anal. 52:374–93
- S. Bhatnagar, R. Sutton, M. Ghavamzadeh, and M. Lee. Natural actor-critic algorithms. Automatica, 45(11): 2471–2482, 2009
- Alpaydin E. 2009. Introduction to Machine Learning. Cambridge, MA: MIT Press
- M. L. Littman. Markov games as a framework for multi-agent reinforcement learning. In Ma- chine Learning, Proceedings of the Eleventh International Conference, Rutgers University, New Brunswick, NJ, USA, July 10-13, 1994, pages 157–163, 1994
- M. Ono, M. Pavone, Y. Kuwata, and J. Balaram. Chance-constrained dynamic programming with application to risk-aware robotic space exploration. Autonomous Robots, 39(4):555–571, 2015
- V. Borkar. A sensitivity formula for the risk-sensitive cost and the actor-critic algorithm. Systems & Control Letters, 44:339–346, 2001
- Kallus N. 2017. Balanced policy evaluation and learning. arXiv:1705.07384 [stat.ML]