AUC Score :
Short-term Tactic1 :
Dominant Strategy :
Time series to forecast n:
ML Model Testing : Supervised Machine Learning (ML)
Hypothesis Testing : Polynomial Regression
Surveillance : Major exchange and OTC
1Short-term revised.
2Time series is updated based on short-term trends.
Key Points
SQM's future appears moderately promising, buoyed by sustained demand for lithium, a key component in electric vehicle batteries, and the company's strong position within the lithium market. Production capacity expansions are likely to drive revenue growth, especially if lithium prices remain robust. However, this growth is significantly contingent upon geopolitical factors influencing the global supply chain and the company's operational environment in Chile. Risks include potential fluctuations in lithium prices, increased competition within the lithium and fertilizer industries, and regulatory changes affecting mining operations. Additionally, the company faces exposure to currency exchange rate volatility, particularly the Chilean peso.About Sociedad Quimica y Minera S.A.
SQM, Sociedad QuĂmica y Minera de Chile S.A., is a Chilean company and a leading global producer of lithium, iodine, potassium, and specialty plant nutrients. Its operations span across diverse geographical locations, with a significant presence in Chile's Atacama Desert, a crucial source of lithium and potassium. SQM plays a critical role in supplying materials essential for various industries, including electric vehicles (EVs) and renewable energy storage, where lithium is a key component. The company also provides iodine, used in medical applications and industrial processes, and specialized plant nutrients that support global agricultural productivity.
The company's integrated business model allows SQM to control the entire value chain, from resource extraction to product manufacturing and distribution. This approach provides significant control over supply and pricing. SQM's strategic focus on lithium production has positioned it at the forefront of the transition towards a sustainable and electrified future. Further, SQM continuously invests in technological innovations to improve production efficiency, reduce environmental impact, and diversify its product portfolio to meet growing global demands.
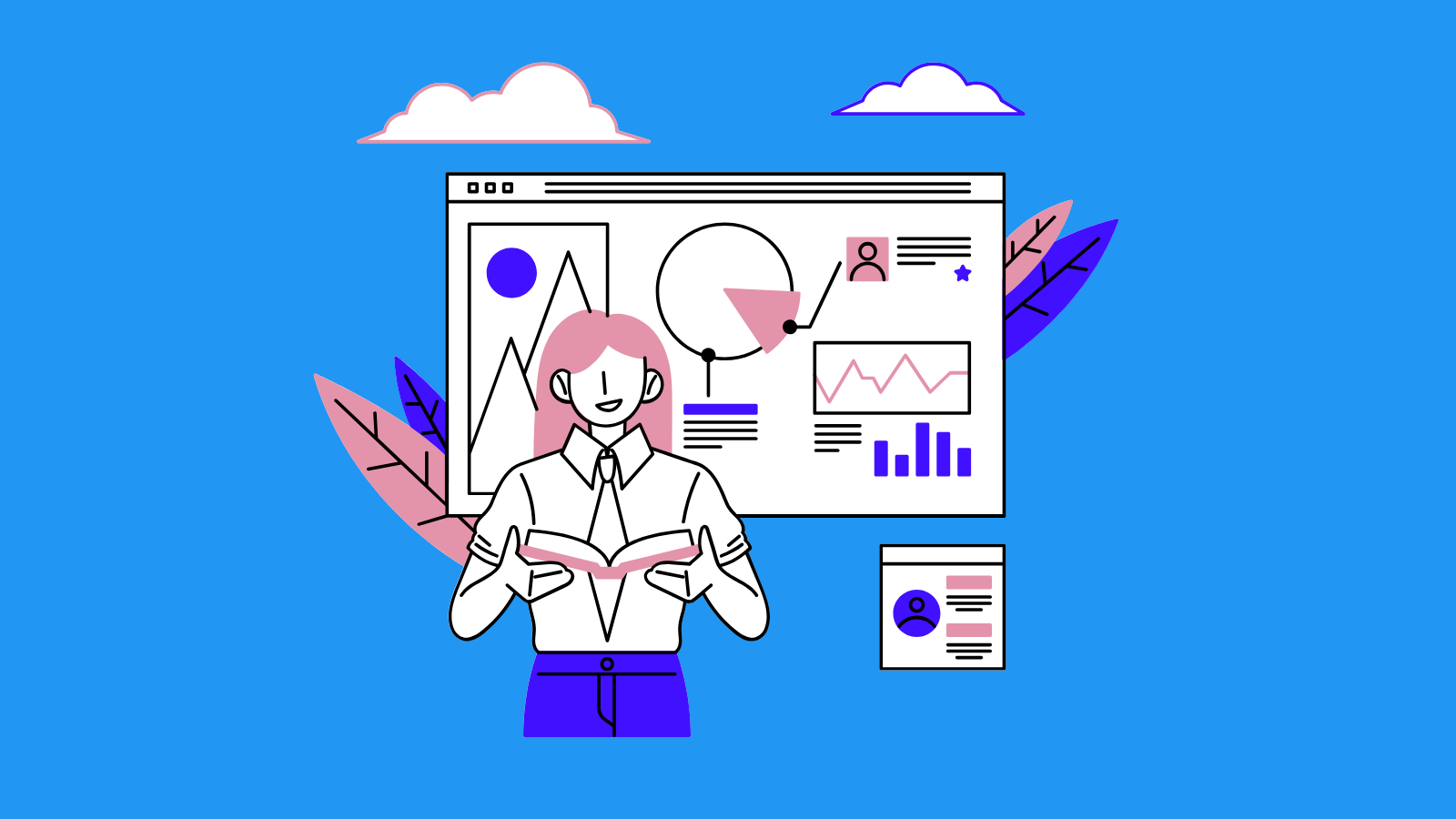
SQM Stock Forecast Model
Our team of data scientists and economists has developed a machine learning model to forecast the performance of Sociedad Quimica y Minera S.A. (SQM) common stock. The model employs a combination of advanced techniques, including Recurrent Neural Networks (RNNs), particularly LSTMs (Long Short-Term Memory) to capture time-series dependencies in the data. We integrate macroeconomic indicators such as global GDP growth, inflation rates, and commodity price indices (specifically lithium, potassium, and iodine prices, which are crucial to SQM's operations) into our feature set. Technical indicators derived from historical SQM trading data, such as moving averages, Relative Strength Index (RSI), and volume data are incorporated to identify potential trends and volatility. The model is trained on a comprehensive dataset covering the past 10 years, ensuring robust statistical power and enabling a dynamic learning process.
The model's architecture involves multiple layers, including an input layer receiving a standardized set of features, followed by LSTM layers to process sequential data. These layers are designed to understand patterns in the data. We then employ dense layers with an activation function suitable for regression tasks and a final output layer which predicts the future direction of the stock. During training, we employ a backpropagation algorithm and optimize the model parameters using an adaptive optimizer, ensuring convergence towards an optimal solution. The model's performance is continuously monitored by using validation datasets to track overfitting and to assess its generalization capability. The model's predictions are then thoroughly evaluated using metrics like Mean Absolute Error (MAE) and Root Mean Squared Error (RMSE) to assess prediction accuracy, and the Sharpe ratio is calculated to gauge the risk-adjusted performance of the model's forecast strategy.
The output of our model is designed to provide insights into the potential direction of SQM stock, not a price. The model forecasts include probabilities of stock price performance, such as "increasing," "decreasing," or "stable". The model can also forecast a range of potential returns. The model is refreshed and retrained on a regular basis to incorporate new information and to ensure that it remains aligned with current market conditions. We have also included a robustness check by sensitivity analysis, testing the impact of changes in key input variables on model outputs. This will enable us to give SQM management a tool for strategic decision-making, including identifying favorable periods for investment and for risk management purposes.
ML Model Testing
n:Time series to forecast
p:Price signals of Sociedad Quimica y Minera S.A. stock
j:Nash equilibria (Neural Network)
k:Dominated move of Sociedad Quimica y Minera S.A. stock holders
a:Best response for Sociedad Quimica y Minera S.A. target price
For further technical information as per how our model work we invite you to visit the article below:
How do KappaSignal algorithms actually work?
Sociedad Quimica y Minera S.A. Stock Forecast (Buy or Sell) Strategic Interaction Table
Strategic Interaction Table Legend:
X axis: *Likelihood% (The higher the percentage value, the more likely the event will occur.)
Y axis: *Potential Impact% (The higher the percentage value, the more likely the price will deviate.)
Z axis (Grey to Black): *Technical Analysis%
SQM Financial Outlook and Forecast
SQM, a leading global producer of lithium, iodine, and specialty plant nutrients, is poised for continued growth, driven by the escalating demand for lithium in the electric vehicle (EV) and energy storage sectors. The company's robust production capacity, geographically diversified operations, and strategic partnerships position it favorably to capitalize on this trend. Recent financial results demonstrate strong profitability, underpinned by favorable lithium pricing and increasing sales volumes. The company has also demonstrated its commitment to sustainable mining practices, which is increasingly important to investors and customers. Furthermore, SQM's focus on cost optimization and operational efficiency is expected to further bolster its financial performance.
The outlook for SQM is heavily reliant on the trajectory of the global EV market and the wider adoption of energy storage systems. Demand for lithium is projected to surge in the coming years, potentially outstripping supply, which could maintain or even increase lithium prices. SQM's expansion projects, including the Salar de Atacama in Chile and its international ventures, are strategically designed to meet this rising demand. The company's diversified product portfolio, encompassing iodine and plant nutrients, provides a degree of insulation against fluctuations in any single market. Moreover, SQM's established relationships with key customers, including major EV manufacturers and battery producers, ensures a stable revenue stream and enhances its ability to adapt to evolving market dynamics.
Key factors influencing SQM's financial performance include lithium pricing volatility, production costs, and geopolitical risks. Any changes in lithium prices will directly impact the company's revenue and profitability. Production costs, influenced by factors such as energy expenses, labor, and regulatory compliance, represent a critical aspect of operational efficiency. Geopolitical risks, particularly those related to Chile's political climate and resource regulations, could introduce uncertainties to the company's operations. Furthermore, competition within the lithium market could intensify, which may affect SQM's market share and pricing power. The company's ability to effectively manage these risks is essential for sustaining its growth trajectory.
SQM is anticipated to maintain a positive financial outlook. This is based on the robust demand for lithium and the company's strong operational positioning within the market. The positive forecast is accompanied by the risks of a slowdown in EV adoption rates, the volatility of lithium prices, and geopolitical uncertainties. To mitigate these risks, SQM must proactively manage its operational costs, monitor global market dynamics, and maintain strategic relationships with key customers and suppliers. Successful execution of its expansion plans and continued focus on innovation will be vital to sustained growth and profitability.
```
Rating | Short-Term | Long-Term Senior |
---|---|---|
Outlook | B1 | Ba1 |
Income Statement | Baa2 | Baa2 |
Balance Sheet | Caa2 | Baa2 |
Leverage Ratios | Caa2 | Caa2 |
Cash Flow | Baa2 | B1 |
Rates of Return and Profitability | Caa2 | Baa2 |
*Financial analysis is the process of evaluating a company's financial performance and position by neural network. It involves reviewing the company's financial statements, including the balance sheet, income statement, and cash flow statement, as well as other financial reports and documents.
How does neural network examine financial reports and understand financial state of the company?
References
- Chernozhukov V, Escanciano JC, Ichimura H, Newey WK. 2016b. Locally robust semiparametric estimation. arXiv:1608.00033 [math.ST]
- S. Bhatnagar. An actor-critic algorithm with function approximation for discounted cost constrained Markov decision processes. Systems & Control Letters, 59(12):760–766, 2010
- Jorgenson, D.W., Weitzman, M.L., ZXhang, Y.X., Haxo, Y.M. and Mat, Y.X., 2023. S&P 500: Is the Bull Market Ready to Run Out of Steam?. AC Investment Research Journal, 220(44).
- Abadie A, Cattaneo MD. 2018. Econometric methods for program evaluation. Annu. Rev. Econ. 10:465–503
- Friedman JH. 2002. Stochastic gradient boosting. Comput. Stat. Data Anal. 38:367–78
- A. Shapiro, W. Tekaya, J. da Costa, and M. Soares. Risk neutral and risk averse stochastic dual dynamic programming method. European journal of operational research, 224(2):375–391, 2013
- S. Bhatnagar, H. Prasad, and L. Prashanth. Stochastic recursive algorithms for optimization, volume 434. Springer, 2013