AUC Score :
Short-term Tactic1 :
Dominant Strategy :
Time series to forecast n:
ML Model Testing : Multi-Task Learning (ML)
Hypothesis Testing : Wilcoxon Sign-Rank Test
Surveillance : Major exchange and OTC
1Short-term revised.
2Time series is updated based on short-term trends.
Key Points
The Dow Jones U.S. Semiconductors Index is anticipated to experience moderate growth, driven by increasing demand for artificial intelligence applications and continued advancements in chip technology. This sector is likely to benefit from government support, especially in areas related to domestic chip manufacturing. However, this positive outlook carries risks; geopolitical tensions, particularly those involving trade and access to critical materials, pose a significant threat. Additionally, potential oversupply due to aggressive expansion by several manufacturers and unexpected changes in consumer spending on electronics could negatively impact this sector's performance. Furthermore, rapid technological obsolescence presents a constant challenge, as new, more efficient chips could quickly render existing ones less competitive.About Dow Jones U.S. Semiconductors Index
The Dow Jones U.S. Semiconductors Index is a market capitalization-weighted index designed to track the performance of companies involved in the semiconductor industry within the United States. This index serves as a benchmark for investors seeking exposure to the sector, offering a comprehensive view of the industry's overall health and growth trajectory. It comprises a selection of publicly traded companies that manufacture, design, and sell semiconductors and related equipment, reflecting the dynamic nature of the technology sector. The index's construction ensures representation of the largest and most influential players, making it a widely followed indicator of industry trends and investment opportunities.
The Dow Jones U.S. Semiconductors Index is rebalanced periodically to maintain its accuracy and reflect changes in the market. This process involves adjusting the weightings of constituent companies based on their market capitalization and other relevant factors. The index provides a transparent and readily accessible tool for tracking the performance of semiconductor companies, enabling investors to assess risk, evaluate portfolio diversification, and compare investment strategies within the technology sector. The index's methodology and constituent composition are clearly defined, making it a reliable tool for market analysis and investment decision-making.
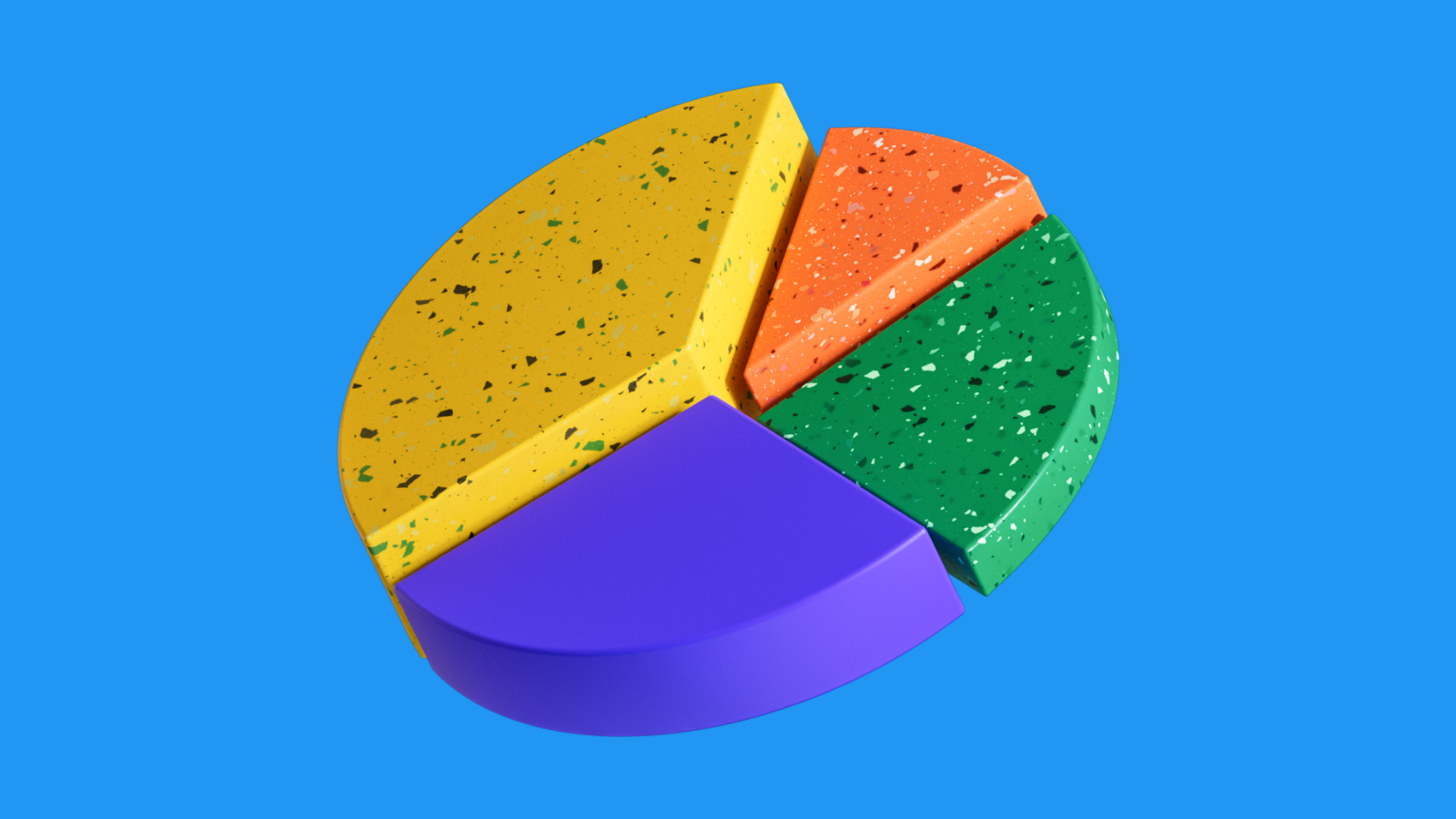
Machine Learning Model for Dow Jones U.S. Semiconductors Index Forecast
The objective is to develop a robust machine learning model to forecast the Dow Jones U.S. Semiconductors Index. Our approach will integrate several key steps. First, a comprehensive dataset will be compiled, encompassing various economic indicators, industry-specific metrics, and market sentiment data. Economic indicators will include GDP growth, inflation rates, interest rates (e.g., Federal Funds Rate), and unemployment figures, providing a broad understanding of the macroeconomic environment. Industry-specific data will involve semiconductor sales figures, manufacturing data (e.g., Purchasing Managers' Index), and technological advancements like new product releases and research & development spending. We will also incorporate market sentiment data through volatility indexes (VIX), news sentiment analysis (using NLP techniques), and social media trends, which often influence short-term market movements. This diverse dataset will ensure the model captures the multifaceted factors impacting the index.
Next, we will employ a time-series analysis framework with multiple machine learning algorithms. Initially, we will use Autoregressive Integrated Moving Average (ARIMA) and Exponential Smoothing models as baseline benchmarks. Subsequently, we will explore more advanced techniques, including Recurrent Neural Networks (RNNs), specifically Long Short-Term Memory (LSTM) networks, known for their efficacy in time-series forecasting due to their ability to learn temporal dependencies. Additionally, ensemble methods such as Random Forests and Gradient Boosting Machines will be implemented to improve predictive accuracy. The models will be trained using historical data, and we will meticulously validate the performance using techniques such as cross-validation, time-series splits, and backtesting. Key performance metrics will include Mean Absolute Error (MAE), Root Mean Squared Error (RMSE), and R-squared to evaluate forecast accuracy. Furthermore, feature importance analysis will be used to understand which variables have the most influence on index movement.
Finally, our model will incorporate risk management and interpretability. Forecasts will be accompanied by confidence intervals to communicate the uncertainty of the predictions. To improve interpretability, we will utilize techniques to explain the model's decisions, especially with complex models like LSTM. This includes examining feature importance and SHAP values. This approach helps users understand the underlying drivers of the forecast and enhances trust in the model's predictions. We will also create a monitoring system to track model performance over time and retrain the model using the latest data to adapt to evolving market conditions. This strategy guarantees the long-term relevance and effectiveness of our forecasts.
ML Model Testing
n:Time series to forecast
p:Price signals of Dow Jones U.S. Semiconductors index
j:Nash equilibria (Neural Network)
k:Dominated move of Dow Jones U.S. Semiconductors index holders
a:Best response for Dow Jones U.S. Semiconductors target price
For further technical information as per how our model work we invite you to visit the article below:
How do KappaSignal algorithms actually work?
Dow Jones U.S. Semiconductors Index Forecast Strategic Interaction Table
Strategic Interaction Table Legend:
X axis: *Likelihood% (The higher the percentage value, the more likely the event will occur.)
Y axis: *Potential Impact% (The higher the percentage value, the more likely the price will deviate.)
Z axis (Grey to Black): *Technical Analysis%
Dow Jones U.S. Semiconductors Index: Outlook and Forecast
The financial outlook for the Dow Jones U.S. Semiconductors Index is currently robust, largely driven by sustained global demand for semiconductor chips across various sectors. The ongoing digital transformation, encompassing cloud computing, artificial intelligence, the Internet of Things (IoT), and the expansion of 5G networks, fuels consistent demand for advanced semiconductor technologies. This strong demand, coupled with advancements in chip manufacturing, such as the move towards smaller node technologies and innovative packaging solutions, is supporting revenue growth and profit margins across the industry. Furthermore, government initiatives, particularly in the U.S., aimed at bolstering domestic semiconductor manufacturing capacity through subsidies and tax incentives, are contributing to increased investment and long-term growth prospects. This proactive approach is designed to reduce reliance on foreign suppliers and enhance national competitiveness in this strategically important sector. The industry also benefits from cyclical upturns in specific segments like automotive and consumer electronics, which further contribute to revenue and profitability growth.
Looking ahead, the forecast for the semiconductor index remains generally positive, albeit with potential for some moderation in the rate of growth compared to the recent, exceptionally high-growth periods. Several factors support this optimistic outlook, including the continued expansion of data centers and the increasing reliance on advanced computing for various applications. The automotive sector, with the ongoing transition to electric vehicles (EVs) and the integration of advanced driver-assistance systems (ADAS), presents another significant growth driver, demanding higher volumes of chips for vehicle control and infotainment systems. Moreover, the growing adoption of AI across various industries, from healthcare to finance, is fueling demand for high-performance chips required for complex computations. The industry is continuously innovating, with companies investing heavily in research and development to create more powerful, efficient, and specialized chips to cater to the evolving needs of the market. Geographic diversification and increased investment in emerging markets will also play a role in driving demand.
However, it is important to consider potential headwinds that could impact the semiconductor index's performance. Geopolitical tensions and trade disputes, particularly those related to access to advanced chip technologies and manufacturing equipment, pose a significant risk. Furthermore, the cyclical nature of the semiconductor industry means that periods of high demand can be followed by periods of oversupply, potentially leading to price pressures and margin compression. Macroeconomic factors, such as fluctuations in interest rates and inflation, can also influence consumer spending and business investment, impacting demand for electronic devices and, consequently, semiconductors. The high capital expenditure required to build and operate semiconductor manufacturing facilities, and the long lead times associated with these projects, also necessitate careful financial management and strategic planning by companies within the index. Moreover, competition between major semiconductor manufacturers is likely to remain intense, which may require continuous innovation and efficiency improvements.
Overall, the Dow Jones U.S. Semiconductors Index is predicted to experience continued, albeit potentially moderating, growth over the forecast horizon. This positive outlook is supported by strong underlying demand drivers and ongoing technological advancements. However, there are several risks to this forecast. Potential geopolitical disruptions, including trade restrictions and supply chain vulnerabilities, present a significant downside risk. The cyclical nature of the industry and macroeconomic uncertainties, especially related to inflation and interest rates, are further risks. In addition, increased competition in the chip manufacturing landscape may lead to margin pressures. Prudent investors should carefully consider these risks when evaluating investments in the semiconductor sector, as the market is characterized by periods of volatility.
Rating | Short-Term | Long-Term Senior |
---|---|---|
Outlook | B1 | B2 |
Income Statement | Caa2 | B3 |
Balance Sheet | Ba3 | Caa2 |
Leverage Ratios | Ba3 | Baa2 |
Cash Flow | Baa2 | B2 |
Rates of Return and Profitability | C | Caa2 |
*An aggregate rating for an index summarizes the overall sentiment towards the companies it includes. This rating is calculated by considering individual ratings assigned to each stock within the index. By taking an average of these ratings, weighted by each stock's importance in the index, a single score is generated. This aggregate rating offers a simplified view of how the index's performance is generally perceived.
How does neural network examine financial reports and understand financial state of the company?
References
- Efron B, Hastie T, Johnstone I, Tibshirani R. 2004. Least angle regression. Ann. Stat. 32:407–99
- R. Sutton and A. Barto. Reinforcement Learning. The MIT Press, 1998
- Nie X, Wager S. 2019. Quasi-oracle estimation of heterogeneous treatment effects. arXiv:1712.04912 [stat.ML]
- J. N. Foerster, Y. M. Assael, N. de Freitas, and S. Whiteson. Learning to communicate with deep multi-agent reinforcement learning. In Advances in Neural Information Processing Systems 29: Annual Conference on Neural Information Processing Systems 2016, December 5-10, 2016, Barcelona, Spain, pages 2137–2145, 2016.
- Friedman JH. 2002. Stochastic gradient boosting. Comput. Stat. Data Anal. 38:367–78
- Abadie A, Cattaneo MD. 2018. Econometric methods for program evaluation. Annu. Rev. Econ. 10:465–503
- Jorgenson, D.W., Weitzman, M.L., ZXhang, Y.X., Haxo, Y.M. and Mat, Y.X., 2023. S&P 500: Is the Bull Market Ready to Run Out of Steam?. AC Investment Research Journal, 220(44).