AUC Score :
Short-term Tactic1 :
Dominant Strategy :
Time series to forecast n:
ML Model Testing : Deductive Inference (ML)
Hypothesis Testing : Logistic Regression
Surveillance : Major exchange and OTC
1Short-term revised.
2Time series is updated based on short-term trends.
Key Points
AOBC is expected to experience moderate growth, driven by continued demand for firearms and outdoor products. The company's ability to innovate and expand its product offerings, particularly in the accessories and ammunition segments, will be key to this growth. However, AOBC faces risks including fluctuations in consumer demand, increased competition from established and emerging players, and potential regulatory changes impacting firearms sales. The company's reliance on the firearms market makes it susceptible to political and social pressures, which could significantly impact its financial performance. Furthermore, supply chain disruptions and inflationary pressures may present challenges to profitability. Despite the potential for growth, investors should be aware of the inherent volatility associated with the firearms industry and the impact of external factors on the company's business.About American Outdoor Brands
American Outdoor Brands (AOB) is a publicly traded company operating primarily in the firearms and outdoor products industries. Originally spun off from Smith & Wesson Brands, the company focuses on manufacturing, marketing, and selling a diverse portfolio of shooting sports, hunting, and rugged outdoor lifestyle products. AOB's strategy emphasizes brand development, innovation, and strategic acquisitions to expand its market presence and product offerings. The company aims to provide consumers with high-quality, reliable products that meet their diverse needs in outdoor recreational activities.
AOB's operations encompass various well-known brands, including firearms and related accessories, as well as outdoor equipment and accessories. The company distributes its products through a variety of channels, including retail stores, online platforms, and direct sales to consumers. AOB's financial performance and strategic direction are significantly influenced by consumer demand, industry trends, and evolving regulatory environments related to firearms and outdoor recreation. Continuous innovation, brand building and strategic partnerships are key drivers for the company's long-term growth.
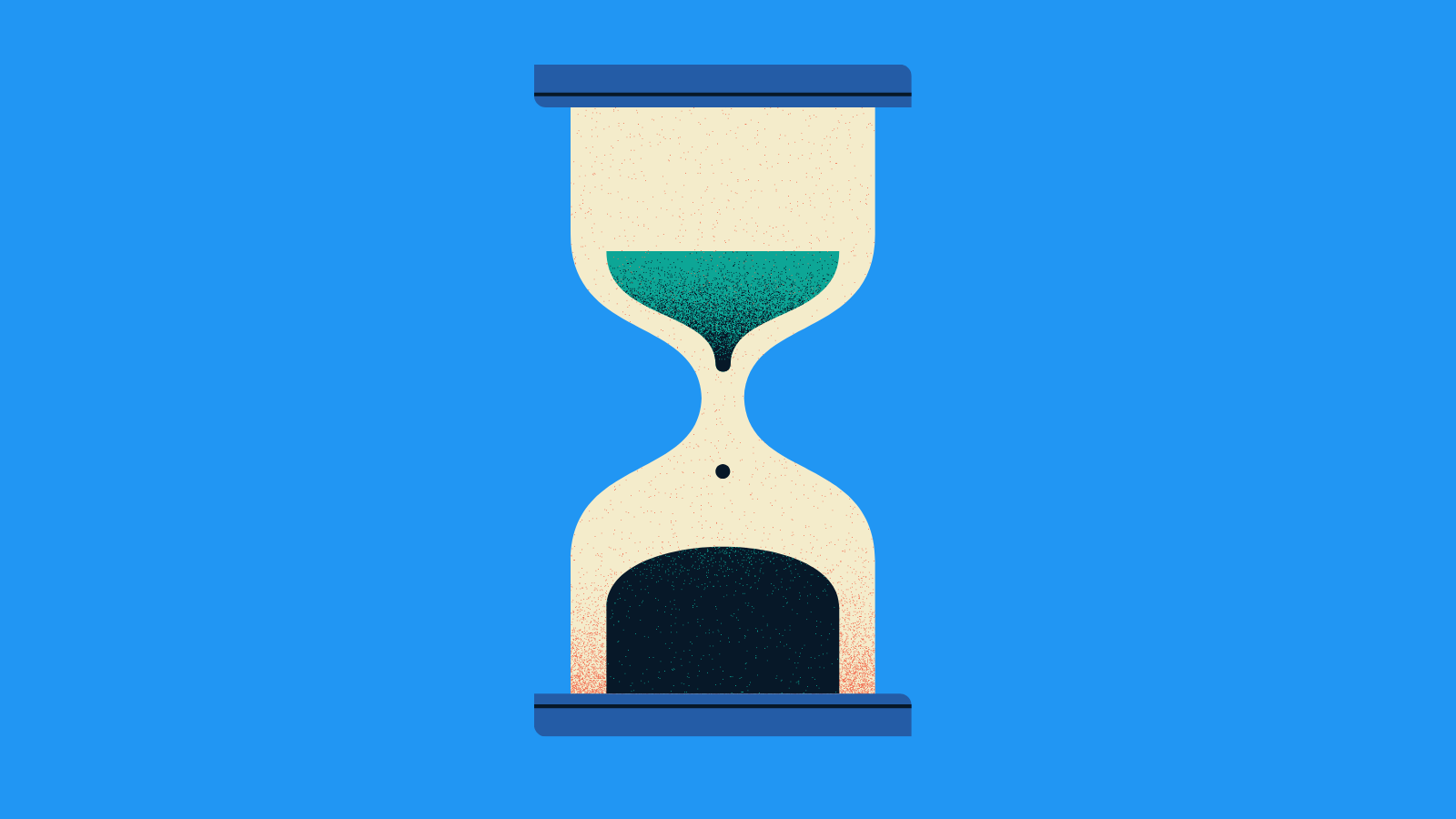
AOUT Stock Forecast: A Machine Learning Model Approach
Our data science and economics team has developed a machine learning model to forecast the performance of American Outdoor Brands Inc. (AOUT) common stock. The model leverages a diverse dataset encompassing historical price data, financial statements (including revenue, earnings per share, and debt levels), and macroeconomic indicators such as inflation rates, interest rates, consumer spending, and industry-specific data related to firearms sales and outdoor recreation activities. We utilized a combination of machine learning algorithms, including Recurrent Neural Networks (RNNs) to capture temporal dependencies in the data and Gradient Boosting Machines to identify complex non-linear relationships. Feature engineering played a critical role, incorporating technical indicators (e.g., moving averages, Relative Strength Index - RSI) and sentiment analysis derived from news articles and social media.
The model's training process involved splitting the historical data into training, validation, and testing sets. The training set was used to teach the algorithms to recognize patterns and make predictions. The validation set allowed us to fine-tune the model's hyperparameters and prevent overfitting. Finally, the testing set evaluated the model's performance on unseen data, providing a robust measure of its forecasting accuracy. We employed evaluation metrics such as Mean Squared Error (MSE), Root Mean Squared Error (RMSE), and Mean Absolute Percentage Error (MAPE) to assess the model's precision. Furthermore, our methodology included rigorous cross-validation to ensure the model's generalizability and robustness across different time periods.
The output of our model is a forecasted range of probable stock behavior over a specified time horizon. The model provides an estimated direction (up, down, or sideways) for AOUT stock, along with confidence intervals. While the model aims to provide useful information, investors are advised to consider our forecast alongside their own independent research and risk tolerance. The stock market is subject to external influences and unforeseen events; therefore, the model is not a guarantee of future performance. Regular monitoring of the model's accuracy and recalibration based on updated data is crucial. Our ongoing work includes refining the model with new data and incorporating additional factors that may influence AOUT's stock performance, and continuous monitoring and analysis.
```
ML Model Testing
n:Time series to forecast
p:Price signals of American Outdoor Brands stock
j:Nash equilibria (Neural Network)
k:Dominated move of American Outdoor Brands stock holders
a:Best response for American Outdoor Brands target price
For further technical information as per how our model work we invite you to visit the article below:
How do KappaSignal algorithms actually work?
American Outdoor Brands Stock Forecast (Buy or Sell) Strategic Interaction Table
Strategic Interaction Table Legend:
X axis: *Likelihood% (The higher the percentage value, the more likely the event will occur.)
Y axis: *Potential Impact% (The higher the percentage value, the more likely the price will deviate.)
Z axis (Grey to Black): *Technical Analysis%
American Outdoor Brands Inc. Financial Outlook and Forecast
American Outdoor Brands (AOB) faces a mixed financial outlook, influenced by several factors impacting the firearms and outdoor recreation industries. The company's performance is directly tied to consumer demand for firearms, ammunition, and related accessories, which can be cyclical and sensitive to external events. Recent years have seen fluctuating demand, partly driven by political and social factors, along with economic uncertainties that influence consumer spending. AOB's ability to maintain market share in a competitive landscape, characterized by established players and emerging competitors, is crucial for its financial health. Expansion into new product categories and diversification of its revenue streams, such as the outdoor recreation segment, are strategies to mitigate reliance on a single product line and improve long-term prospects. Furthermore, AOB's ability to manage its supply chain efficiently and navigate regulatory changes related to firearms will be essential for sustaining profitability.
The company's financial forecast depends on several key drivers. One primary factor is consumer spending patterns and macroeconomic conditions, including inflation and interest rates, which can affect the disposable income available for discretionary purchases like firearms and outdoor gear. AOB's profitability is also subject to cost management, particularly with raw materials and labor, which may impact margins if not efficiently controlled. Competitive pressures will likely require continuous product innovation and effective marketing strategies to maintain or expand its customer base. Furthermore, the success of recent acquisitions and expansions into new product categories, such as outdoor recreation, could contribute to growth. The ability to capitalize on emerging trends in the outdoor market, such as sustainable products or specific recreational activities, is crucial for achieving financial objectives. The successful integration of acquired businesses into the current business structure will also play a vital role in financial forecasts.
Analysts are carefully evaluating the ongoing and future implications of geopolitical events and their impact on AOB. Government regulations regarding firearms sales, manufacturing, and distribution pose another significant risk. Compliance costs and potential legal liabilities can affect the company's financial outlook. Furthermore, the company is vulnerable to shifts in consumer preferences and evolving social attitudes regarding firearms, which could negatively impact sales. The company's strategic decisions, including investments in new products, geographic expansion, and the efficiency of its supply chain management, will heavily influence its financial results. Effective marketing and branding strategies are also necessary to build customer loyalty and maintain market share. Any changes in competition, including pricing strategies and new products, must be considered when assessing the future prospects of the company.
Overall, AOB's financial outlook appears cautiously optimistic. We expect AOB to show moderate growth in the upcoming years, driven by the successful execution of its strategic initiatives and effective management of operational challenges. While the company's diversification efforts into the outdoor recreation market may have a positive impact, it's subject to the same risk factors as its core business. Key risks for AOB include potential changes in consumer demand, increased competition within the firearms and outdoor recreation markets, and any unexpected changes in government regulations or the macroeconomic environment. If AOB can navigate these challenges and effectively manage its operations, the company will likely achieve sustained financial results, creating long-term shareholder value.
```
Rating | Short-Term | Long-Term Senior |
---|---|---|
Outlook | B2 | B1 |
Income Statement | Baa2 | Baa2 |
Balance Sheet | Baa2 | Baa2 |
Leverage Ratios | Caa2 | C |
Cash Flow | C | Caa2 |
Rates of Return and Profitability | Caa2 | C |
*Financial analysis is the process of evaluating a company's financial performance and position by neural network. It involves reviewing the company's financial statements, including the balance sheet, income statement, and cash flow statement, as well as other financial reports and documents.
How does neural network examine financial reports and understand financial state of the company?
References
- Hirano K, Porter JR. 2009. Asymptotics for statistical treatment rules. Econometrica 77:1683–701
- S. Bhatnagar and K. Lakshmanan. An online actor-critic algorithm with function approximation for con- strained Markov decision processes. Journal of Optimization Theory and Applications, 153(3):688–708, 2012.
- Barkan O. 2016. Bayesian neural word embedding. arXiv:1603.06571 [math.ST]
- A. Y. Ng, D. Harada, and S. J. Russell. Policy invariance under reward transformations: Theory and application to reward shaping. In Proceedings of the Sixteenth International Conference on Machine Learning (ICML 1999), Bled, Slovenia, June 27 - 30, 1999, pages 278–287, 1999.
- Abadir, K. M., K. Hadri E. Tzavalis (1999), "The influence of VAR dimensions on estimator biases," Econometrica, 67, 163–181.
- G. Konidaris, S. Osentoski, and P. Thomas. Value function approximation in reinforcement learning using the Fourier basis. In AAAI, 2011
- Bell RM, Koren Y. 2007. Lessons from the Netflix prize challenge. ACM SIGKDD Explor. Newsl. 9:75–79