AUC Score :
Short-term Tactic1 :
Dominant Strategy :
Time series to forecast n:
ML Model Testing : Modular Neural Network (Market Volatility Analysis)
Hypothesis Testing : Linear Regression
Surveillance : Major exchange and OTC
1Short-term revised.
2Time series is updated based on short-term trends.
Key Points
Innodata's future appears mixed. The company may experience moderate revenue growth driven by expansion in AI data solutions, particularly in the e-commerce and technology sectors. However, competition within the AI services market is intensifying, which could limit Innodata's pricing power and profitability. Further, economic downturns or decreased technology spending could negatively impact client demand, thus creating slower revenue. Over-reliance on a small number of key clients presents a concentration risk, while integration risks associated with potential acquisitions and attracting and retaining top technical talent also pose threats to its overall success.About Innodata
Innodata (INOD) is a global data engineering company that focuses on providing advanced data solutions and services. They specialize in the creation, enrichment, and management of large datasets, catering to a diverse clientele. The company employs artificial intelligence and machine learning technologies to extract, structure, and refine data from various sources, including text, images, and audio. Their services support a wide range of industries, such as technology, finance, and healthcare. Innodata's operations span across multiple countries with a significant presence in the Philippines and North America.
The firm's services encompass data annotation, content creation, and data analytics support. Innodata helps businesses improve their AI models and decision-making by delivering high-quality, actionable data. The company aims to enhance the productivity and efficiency of its clients through its data-centric approach. They are constantly developing new offerings to meet evolving market needs in the rapidly changing data landscape. Innodata is committed to maintaining data security and privacy throughout its operations, complying with industry standards.
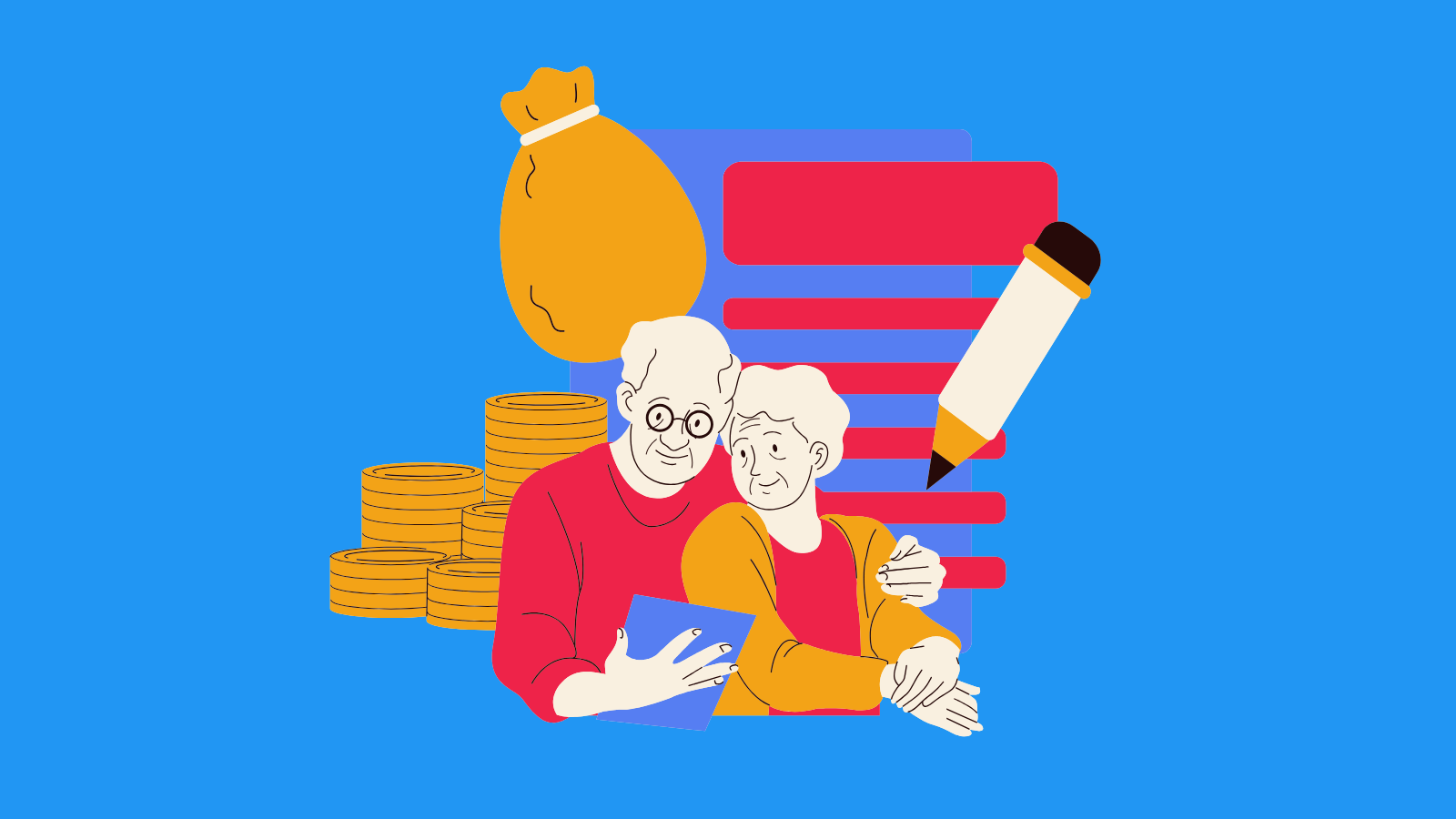
INOD Stock Forecast Model
As a team of data scientists and economists, we propose a comprehensive machine learning model to forecast the future performance of Innodata Inc. Common Stock (INOD). Our approach integrates diverse datasets, including historical stock prices, trading volume, and technical indicators, with macroeconomic variables such as interest rates, inflation, and industry-specific performance metrics. These external factors are critical for capturing market sentiment and economic trends influencing INOD's growth. The model will leverage a combination of advanced machine learning techniques, including Recurrent Neural Networks (RNNs), specifically Long Short-Term Memory (LSTM) networks, renowned for their ability to process sequential data and capture temporal dependencies. This will be supplemented by ensemble methods like Gradient Boosting to optimize predictive accuracy and robustness.
The model's architecture involves several key stages. Firstly, rigorous data preprocessing and cleaning are paramount. This includes handling missing values, outlier detection, and feature engineering to enhance signal extraction. We will use time series analysis to build the data. This will be followed by the data split, 80% will be use for training the model, and 20% for the testing the model. The model's performance will be evaluated using metrics such as Mean Squared Error (MSE), Root Mean Squared Error (RMSE), and Mean Absolute Percentage Error (MAPE) to ensure accuracy and reliability. Cross-validation techniques will be employed to prevent overfitting and assess generalizability. Finally, sensitivity analysis and what-if scenarios will be run to assess the model's robustness under different market conditions and economic environments.
The output of our model will be a set of forecasts indicating the expected direction of INOD's stock performance over specified time horizons. The model will provide probability distributions to describe the range of potential outcomes. Furthermore, we will continuously monitor and refine the model by incorporating new data and adjusting parameters to maintain predictive accuracy. The model will be updated regularly. This dynamic approach, combined with expert economic insights, will provide Innodata with an actionable tool for understanding INOD's stock behavior, enabling more informed investment decisions, and improving strategic planning.
ML Model Testing
n:Time series to forecast
p:Price signals of Innodata stock
j:Nash equilibria (Neural Network)
k:Dominated move of Innodata stock holders
a:Best response for Innodata target price
For further technical information as per how our model work we invite you to visit the article below:
How do KappaSignal algorithms actually work?
Innodata Stock Forecast (Buy or Sell) Strategic Interaction Table
Strategic Interaction Table Legend:
X axis: *Likelihood% (The higher the percentage value, the more likely the event will occur.)
Y axis: *Potential Impact% (The higher the percentage value, the more likely the price will deviate.)
Z axis (Grey to Black): *Technical Analysis%
Innodata Inc. (INOD) Financial Outlook and Forecast
The financial outlook for INOD appears to be cautiously optimistic, underpinned by its position as a provider of data engineering services and its strategic focus on artificial intelligence (AI) solutions. The company's diversification across various sectors, including technology, healthcare, and finance, provides some resilience against economic downturns in any particular industry. Key drivers for future growth include the increasing demand for high-quality training data for AI models, which INOD is well-positioned to provide. The company's emphasis on AI-driven solutions, particularly in areas such as data annotation and model training, aligns with a rapidly expanding market. Further supporting this outlook is INOD's continued investment in proprietary technologies and its ability to adapt to evolving client needs within the AI landscape. The expansion of existing partnerships and the potential for new collaborations with leading AI firms are significant opportunities that could positively influence financial performance. The growth in digital content and the need for organized, accurate data are key demand-side factors that support INOD's core business operations.
INOD's financial forecast reflects a trajectory of steady, though not explosive, growth. Revenue projections are likely to be positive, driven by the demand for AI training data and the expansion of existing client contracts. Profitability is expected to improve over time, primarily due to greater efficiencies in operational cost. Increased automation and streamlined workflows, driven by proprietary technology investments, are projected to contribute to improved gross margins. Furthermore, a strategic focus on higher-margin services, such as model training and AI consulting, is set to support enhanced profitability. Management is expected to emphasize prudent financial management, including debt reduction and strategic capital allocation to ensure long-term financial stability and maintain a sustainable growth rate. The company is focusing on scaling its operations in key markets to meet the demand, which is expected to create more robust cash flow generation.
Crucial to INOD's financial outlook is the company's ability to secure and retain large contracts, particularly those within the AI and data science sectors. Success hinges on maintaining a strong competitive edge, staying ahead of technological advancements, and delivering services of superior quality. The company's capacity to innovate and adapt to the changing needs of its clients, along with its investment in top-tier engineering talent, is essential. Maintaining customer satisfaction is critical to ensure contract renewals and generate positive referrals. Also, the global data and AI markets' evolution is considered a key factor, with growth being dependent on the adoption rate of AI solutions across various industries and the capacity to develop and deploy AI algorithms for multiple purposes.
In summary, the prediction for INOD is positive, with potential for moderate growth. The demand for AI-related services provides strong tailwinds for future financial performance. However, there are notable risks associated with this outlook. The competition within the data and AI service sector is significant, requiring INOD to maintain a competitive edge. Economic downturns within sectors that heavily rely on these services could hurt financial performance. Technological disruption, such as an emergence of new AI training techniques, is also a possible risk. The company is also exposed to the risk of client concentration. Any negative financial outcomes from these areas could significantly impede INOD's future growth potential. However, INOD has a strong foundation, and the demand for its services should drive steady growth.
Rating | Short-Term | Long-Term Senior |
---|---|---|
Outlook | B2 | B1 |
Income Statement | B1 | C |
Balance Sheet | C | Baa2 |
Leverage Ratios | Baa2 | Baa2 |
Cash Flow | Caa2 | C |
Rates of Return and Profitability | B3 | B1 |
*Financial analysis is the process of evaluating a company's financial performance and position by neural network. It involves reviewing the company's financial statements, including the balance sheet, income statement, and cash flow statement, as well as other financial reports and documents.
How does neural network examine financial reports and understand financial state of the company?
References
- L. Prashanth and M. Ghavamzadeh. Actor-critic algorithms for risk-sensitive MDPs. In Proceedings of Advances in Neural Information Processing Systems 26, pages 252–260, 2013.
- Athey S, Wager S. 2017. Efficient policy learning. arXiv:1702.02896 [math.ST]
- A. Y. Ng, D. Harada, and S. J. Russell. Policy invariance under reward transformations: Theory and application to reward shaping. In Proceedings of the Sixteenth International Conference on Machine Learning (ICML 1999), Bled, Slovenia, June 27 - 30, 1999, pages 278–287, 1999.
- Ashley, R. (1983), "On the usefulness of macroeconomic forecasts as inputs to forecasting models," Journal of Forecasting, 2, 211–223.
- M. J. Hausknecht and P. Stone. Deep recurrent Q-learning for partially observable MDPs. CoRR, abs/1507.06527, 2015
- Hartigan JA, Wong MA. 1979. Algorithm as 136: a k-means clustering algorithm. J. R. Stat. Soc. Ser. C 28:100–8
- M. Sobel. The variance of discounted Markov decision processes. Applied Probability, pages 794–802, 1982