AUC Score :
Short-term Tactic1 :
Dominant Strategy :
Time series to forecast n:
ML Model Testing : Modular Neural Network (CNN Layer)
Hypothesis Testing : Chi-Square
Surveillance : Major exchange and OTC
1Short-term revised.
2Time series is updated based on short-term trends.
Key Points
FTAI Aviation faces a mixed outlook. The company is anticipated to benefit from sustained demand within the aviation leasing market, particularly for engine and component leasing, potentially leading to revenue growth. However, FTAI's performance is closely tied to the health of the airline industry, making it vulnerable to economic downturns or unforeseen events such as geopolitical instability or supply chain disruptions that can directly impact airline profitability and aircraft utilization rates, potentially reducing lease income. Furthermore, significant debt levels pose a risk, potentially increasing financial instability during periods of market volatility or rising interest rates. Competitive pressures from established leasing companies and the impact of technological advancements on engine lifecycles also create considerable challenges.About FTAI Aviation
FTAI Aviation Ltd. provides aviation services, specializing in the ownership and leasing of aircraft engines. The company focuses on the commercial aviation sector, offering engine leasing solutions to airlines globally. They also engage in engine sales and provide related maintenance, repair, and overhaul (MRO) services. FTAI's business model centers around acquiring, managing, and remarketing aircraft engines, generating revenue through lease payments and engine-related services. FTAI has a diversified portfolio of engines, serving a wide range of aircraft types and operators.
The company's strategy is to capitalize on the growing demand for aircraft engine leasing and MRO services. FTAI Aviation aims to optimize its engine portfolio's utilization and profitability through strategic acquisitions, efficient asset management, and strong customer relationships. The company seeks to adapt to the evolving needs of the aviation industry, including shifts towards more fuel-efficient engines and stricter environmental regulations, to deliver value to its stakeholders. FTAI Aviation is committed to providing quality engine solutions and related support services.
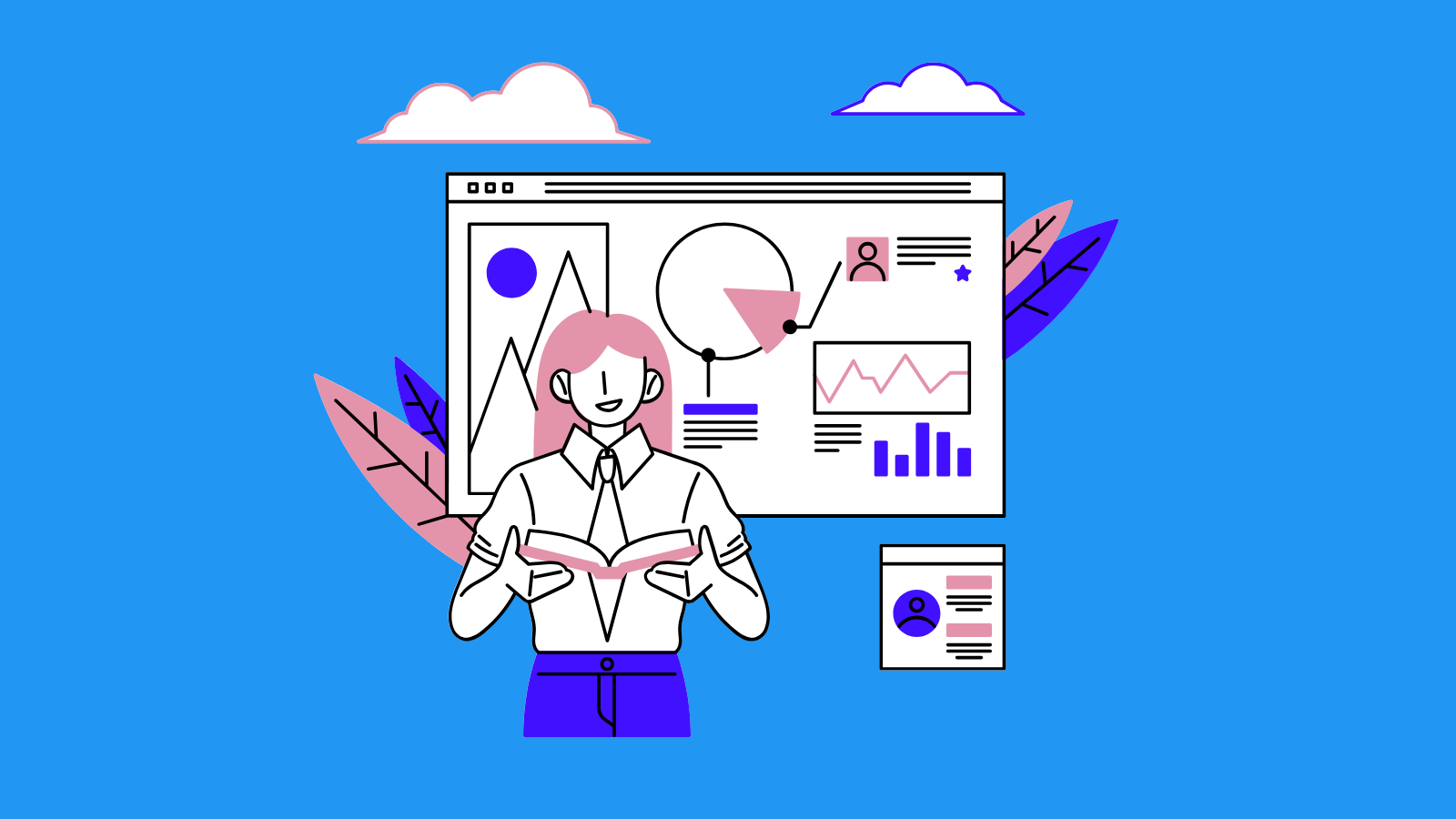
FTAI Aviation Ltd. (FTAI) Stock Forecast Model
Our multidisciplinary team of data scientists and economists has developed a machine learning model designed to forecast the performance of FTAI Aviation Ltd. (FTAI) common stock. This model incorporates a diverse range of predictive variables, drawing from both fundamental and technical analysis. Fundamental factors include the company's financial statements (revenue, earnings, debt levels), industry-specific data (aircraft leasing market trends, cargo demand), and macroeconomic indicators (interest rates, inflation, GDP growth). We also consider qualitative factors such as management quality, competitive landscape, and regulatory environment. The model leverages these inputs to generate probabilistic forecasts, assessing the likelihood of future price movements and identifying potential risks and opportunities. The core algorithm utilizes a combination of time series analysis and supervised learning techniques.
The technical analysis component integrates historical price data, trading volume, and a variety of technical indicators (moving averages, RSI, MACD) to capture market sentiment and trading patterns. This approach allows us to incorporate short-term fluctuations and identify potential support and resistance levels. Feature engineering is a crucial aspect of our methodology; we transform raw data into meaningful features that enhance the model's predictive power. We employ techniques like feature scaling, dimensionality reduction (Principal Component Analysis), and lagged variables to optimize the model's performance and reduce overfitting. The model is trained on historical data, with regular re-training cycles to adapt to evolving market dynamics. We will continually validate and refine the model using out-of-sample testing to ensure robust performance and minimize forecast errors.
Our model provides a comprehensive risk assessment by quantifying the volatility and potential downside risk associated with FTAI stock. We use backtesting, scenario analysis, and sensitivity analysis to evaluate the model's performance across different market conditions. This will help in understanding the model's strengths and weaknesses. Key outputs of the model include predicted price directions, confidence intervals, and risk assessments. The forecasts are intended to aid in informed decision-making for investors, allowing them to assess potential returns and manage risks related to their FTAI stock investments. Our team is committed to continuous monitoring, updates, and improvements to ensure the model remains accurate and relevant.
ML Model Testing
n:Time series to forecast
p:Price signals of FTAI Aviation stock
j:Nash equilibria (Neural Network)
k:Dominated move of FTAI Aviation stock holders
a:Best response for FTAI Aviation target price
For further technical information as per how our model work we invite you to visit the article below:
How do KappaSignal algorithms actually work?
FTAI Aviation Stock Forecast (Buy or Sell) Strategic Interaction Table
Strategic Interaction Table Legend:
X axis: *Likelihood% (The higher the percentage value, the more likely the event will occur.)
Y axis: *Potential Impact% (The higher the percentage value, the more likely the price will deviate.)
Z axis (Grey to Black): *Technical Analysis%
FTAI Aviation Ltd. Common Stock Financial Outlook and Forecast
The financial outlook for FTAI Aviation (FTAI) presents a mixed picture, influenced by the dynamic conditions within the aviation industry. The company, which specializes in leasing, engine services, and infrastructure, is poised to benefit from several key trends. The ongoing recovery in air travel, particularly as global restrictions ease, is driving increased demand for aircraft and engine leases. FTAI's focus on providing engine services positions it well to capitalize on the growing need for maintenance, repair, and overhaul (MRO) services as airlines resume and expand operations. Furthermore, the company's infrastructure segment, involving investments in aviation-related assets, can offer diversification and potential for long-term growth. However, this segment's performance might be subject to the cyclical nature of certain infrastructure projects and changes in regulatory environments. Careful examination of FTAI's revenue streams reveals that their revenue composition, with an increasing proportion coming from these services, may contribute to more stable revenue over time.
Analysing FTAI's financials, important factors need to be closely evaluated to form an accurate prediction. Considerations include the company's debt levels and its ability to manage financial leverage, as high debt could pose a risk, particularly if interest rates increase. The profitability of its various business segments, including lease rates and engine service margins, must be carefully reviewed. Furthermore, the strength of the company's backlog and pipeline of future business offers a crucial indicator of future revenue generation. A healthy backlog, indicating sustained customer demand, can provide reassurance about long-term growth prospects. Operational efficiency, including cost management and the ability to adapt to changes in the aviation industry, also affect FTAI's performance. By critically assessing these factors, analysts can formulate a prediction that reflects the evolving industry landscape and FTAI's strategic positioning.
The assessment of FTAI's competitiveness should be examined considering its peers within the aviation services and leasing sectors. Understanding FTAI's market share and its ability to compete with larger, more established players is crucial. Key differentiating factors, such as its specialised focus on engine services and its diverse infrastructure assets, will set it apart from the competition. Technological innovation and adaptation to evolving industry standards, including the growing emphasis on sustainable aviation practices, are imperative. FTAI's ability to develop cutting-edge products, services, and adopt efficient operational methods will significantly impact its future prospects. Moreover, an understanding of the company's management team and their past strategic decisions will influence the long-term growth of the company. Proper decisions and strong leadership will be the cornerstone of success.
The financial outlook for FTAI is cautiously optimistic. The positive trend in air travel recovery and the expanding demand for its engine services suggest growth. The infrastructure segment holds potential, albeit with inherent risks. However, the company's success hinges on managing its debt, maintaining profitability, and effectively executing its strategic vision. The main risk is the aviation industry's volatility, with factors such as fluctuating fuel prices, economic downturns, and geopolitical uncertainties possibly affecting FTAI's profitability. Increased competition from bigger players and emerging technological disruptions also pose challenges. Considering these factors, a moderate long-term growth is likely.
Rating | Short-Term | Long-Term Senior |
---|---|---|
Outlook | B1 | B1 |
Income Statement | Baa2 | C |
Balance Sheet | Ba3 | C |
Leverage Ratios | C | Ba1 |
Cash Flow | Ba3 | B1 |
Rates of Return and Profitability | B3 | Baa2 |
*Financial analysis is the process of evaluating a company's financial performance and position by neural network. It involves reviewing the company's financial statements, including the balance sheet, income statement, and cash flow statement, as well as other financial reports and documents.
How does neural network examine financial reports and understand financial state of the company?
References
- Athey S, Imbens GW. 2017a. The econometrics of randomized experiments. In Handbook of Economic Field Experiments, Vol. 1, ed. E Duflo, A Banerjee, pp. 73–140. Amsterdam: Elsevier
- Swaminathan A, Joachims T. 2015. Batch learning from logged bandit feedback through counterfactual risk minimization. J. Mach. Learn. Res. 16:1731–55
- A. Tamar, Y. Glassner, and S. Mannor. Policy gradients beyond expectations: Conditional value-at-risk. In AAAI, 2015
- Breiman L, Friedman J, Stone CJ, Olshen RA. 1984. Classification and Regression Trees. Boca Raton, FL: CRC Press
- Wu X, Kumar V, Quinlan JR, Ghosh J, Yang Q, et al. 2008. Top 10 algorithms in data mining. Knowl. Inform. Syst. 14:1–37
- Li L, Chen S, Kleban J, Gupta A. 2014. Counterfactual estimation and optimization of click metrics for search engines: a case study. In Proceedings of the 24th International Conference on the World Wide Web, pp. 929–34. New York: ACM
- Arora S, Li Y, Liang Y, Ma T. 2016. RAND-WALK: a latent variable model approach to word embeddings. Trans. Assoc. Comput. Linguist. 4:385–99