AUC Score :
Short-term Tactic1 :
Dominant Strategy :
Time series to forecast n:
ML Model Testing : Modular Neural Network (Market News Sentiment Analysis)
Hypothesis Testing : Independent T-Test
Surveillance : Major exchange and OTC
1Short-term revised.
2Time series is updated based on short-term trends.
Key Points
This exclusive content is only available to premium users.About APTV
This exclusive content is only available to premium users.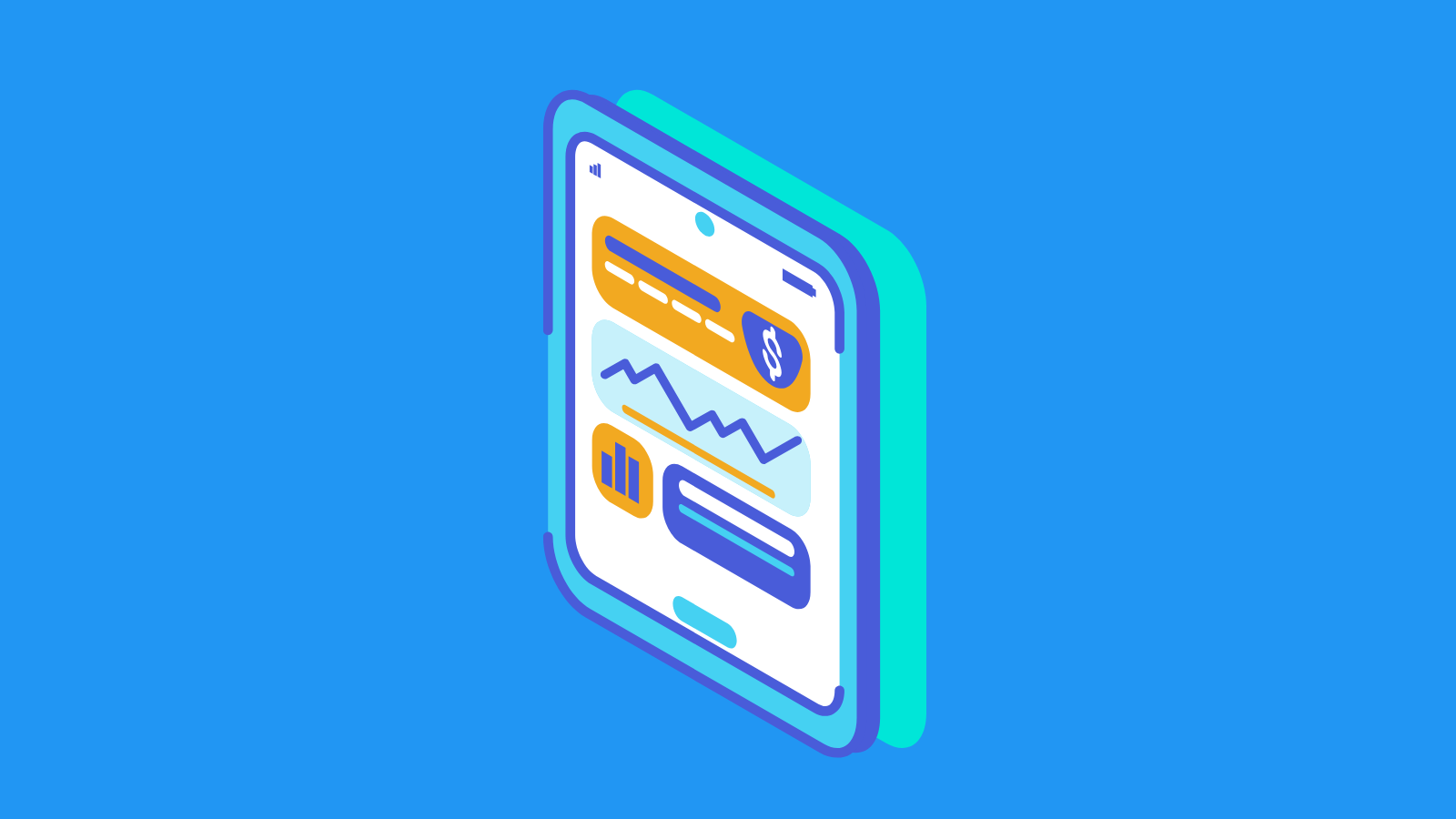
ML Model Testing
n:Time series to forecast
p:Price signals of APTV stock
j:Nash equilibria (Neural Network)
k:Dominated move of APTV stock holders
a:Best response for APTV target price
For further technical information as per how our model work we invite you to visit the article below:
How do KappaSignal algorithms actually work?
APTV Stock Forecast (Buy or Sell) Strategic Interaction Table
Strategic Interaction Table Legend:
X axis: *Likelihood% (The higher the percentage value, the more likely the event will occur.)
Y axis: *Potential Impact% (The higher the percentage value, the more likely the price will deviate.)
Z axis (Grey to Black): *Technical Analysis%
Rating | Short-Term | Long-Term Senior |
---|---|---|
Outlook | B2 | Ba3 |
Income Statement | Baa2 | B2 |
Balance Sheet | B1 | B3 |
Leverage Ratios | C | Baa2 |
Cash Flow | B1 | B3 |
Rates of Return and Profitability | Caa2 | Baa2 |
*Financial analysis is the process of evaluating a company's financial performance and position by neural network. It involves reviewing the company's financial statements, including the balance sheet, income statement, and cash flow statement, as well as other financial reports and documents.
How does neural network examine financial reports and understand financial state of the company?
References
- Swaminathan A, Joachims T. 2015. Batch learning from logged bandit feedback through counterfactual risk minimization. J. Mach. Learn. Res. 16:1731–55
- Belsley, D. A. (1988), "Modelling and forecast reliability," International Journal of Forecasting, 4, 427–447.
- T. Morimura, M. Sugiyama, M. Kashima, H. Hachiya, and T. Tanaka. Nonparametric return distribution ap- proximation for reinforcement learning. In Proceedings of the 27th International Conference on Machine Learning, pages 799–806, 2010
- H. Khalil and J. Grizzle. Nonlinear systems, volume 3. Prentice hall Upper Saddle River, 2002.
- K. Boda, J. Filar, Y. Lin, and L. Spanjers. Stochastic target hitting time and the problem of early retirement. Automatic Control, IEEE Transactions on, 49(3):409–419, 2004
- Arjovsky M, Bottou L. 2017. Towards principled methods for training generative adversarial networks. arXiv:1701.04862 [stat.ML]
- Dudik M, Erhan D, Langford J, Li L. 2014. Doubly robust policy evaluation and optimization. Stat. Sci. 29:485–511