AUC Score :
Short-term Tactic1 :
Dominant Strategy :
Time series to forecast n:
ML Model Testing : Modular Neural Network (CNN Layer)
Hypothesis Testing : Stepwise Regression
Surveillance : Major exchange and OTC
1Short-term revised.
2Time series is updated based on short-term trends.
Key Points
WBY's growth trajectory is expected to be characterized by moderate expansion, driven by its brand recognition and omnichannel presence, with potential for increased market share in the eyewear industry. This projection assumes successful execution of its retail expansion strategy and ongoing innovation in product offerings and customer experience. Risks include heightened competition from both established players and emerging direct-to-consumer brands, which could pressure margins. Economic downturns and shifts in consumer spending habits pose further challenges, potentially affecting sales volume. In addition, the company is vulnerable to supply chain disruptions and increasing operational costs.About Warby Parker
Warby Parker (WRBY), founded in 2010, is a direct-to-consumer brand focused on eyewear. The company designs, markets, and retails prescription glasses, sunglasses, and contact lenses primarily through its website and physical retail stores. Warby Parker emphasizes a customer-centric approach, offering a home try-on program, virtual try-on tools, and a commitment to social responsibility, including its "Buy a Pair, Give a Pair" program, where it donates glasses to individuals in need for every pair sold.
WRBY differentiates itself through its vertically integrated model, controlling design, manufacturing, and distribution, which allows for greater control over product quality and pricing. The company has expanded beyond eyeglasses to include eye exams and other vision-related products. Warby Parker targets a digitally-savvy customer base, utilizing data and technology to personalize the shopping experience and drive brand engagement. The company aims to disrupt the traditional eyewear industry by providing stylish, accessible, and affordable eyewear options.
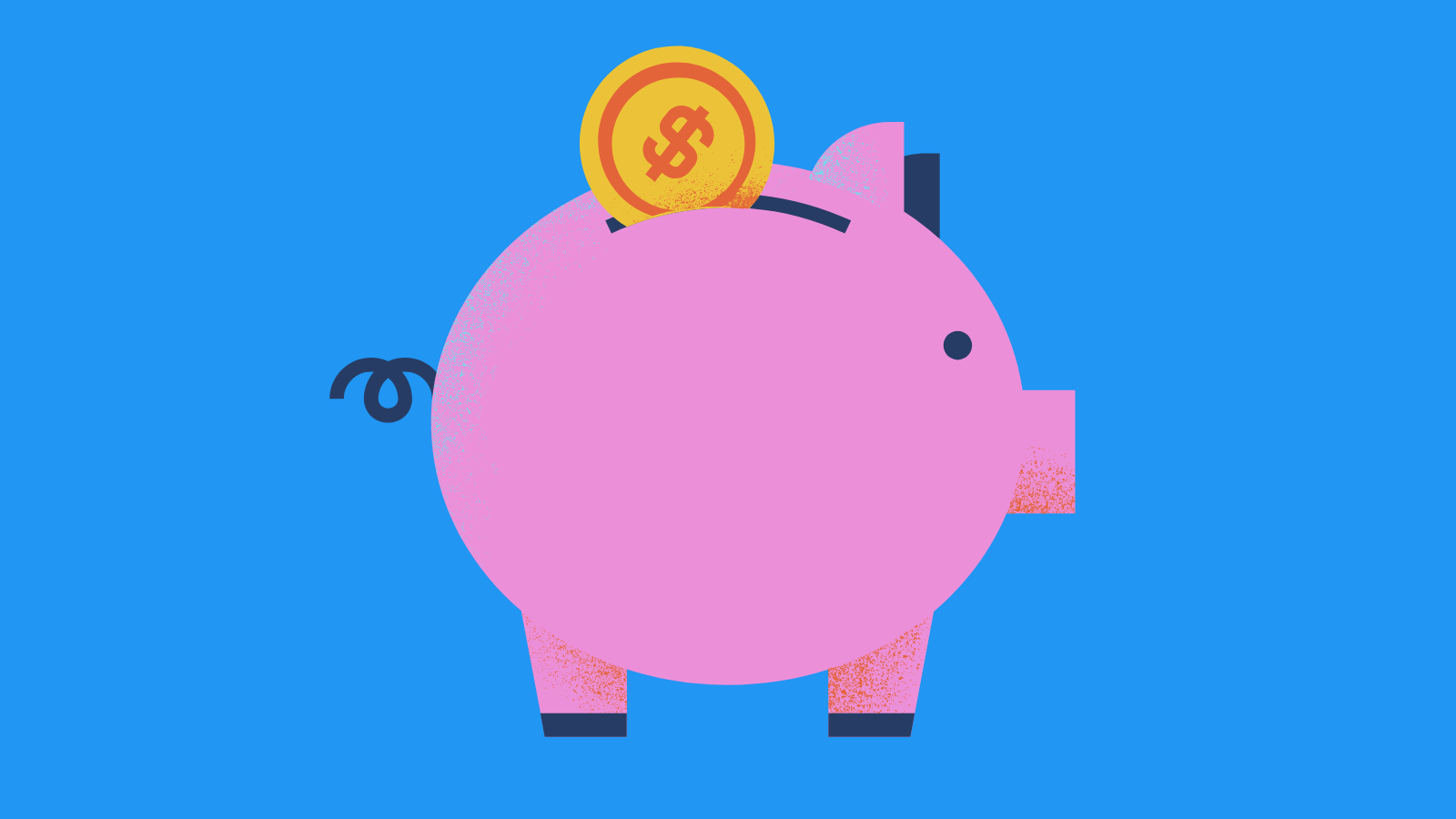
WRBY Stock Forecast Model
Our team of data scientists and economists has developed a machine learning model to forecast Warby Parker Inc. Class A Common Stock (WRBY). The model leverages a diverse set of features encompassing financial performance, market sentiment, macroeconomic indicators, and competitive landscape analysis. Financial data includes key metrics like revenue growth, gross margin, operating expenses, and net income, sourced from the company's filings and financial news aggregators. Market sentiment is gauged through natural language processing (NLP) of news articles, social media mentions, and analyst reports, identifying positive, negative, or neutral sentiment scores. Macroeconomic indicators such as consumer confidence, inflation rates, and interest rates are incorporated to reflect the broader economic environment's potential impact on consumer spending and business performance. Finally, the model considers the competitive landscape, assessing the performance of rival companies in the eyewear industry and the broader e-commerce market.
The model utilizes a hybrid approach, combining the strengths of multiple machine learning algorithms. A time series component, such as a recurrent neural network (RNN) or a Long Short-Term Memory (LSTM) network, analyzes historical stock data to identify trends and patterns. Simultaneously, a gradient boosting model, such as XGBoost or LightGBM, processes the feature set derived from financial statements, sentiment analysis, macroeconomic data, and competitive analysis to capture non-linear relationships. The outputs of these two components are then integrated through an ensemble method, effectively combining the predictive power of both. The model is trained on historical data and regularly re-trained with fresh data to maintain its predictive accuracy. The model's performance is continuously evaluated using metrics such as Mean Absolute Error (MAE), Root Mean Squared Error (RMSE), and R-squared, considering both in-sample and out-of-sample periods, and using rigorous backtesting methods to evaluate its predictive performance.
The output of the model is a probabilistic forecast of the WRBY stock's future performance, presented in the form of expected direction, confidence intervals, and probabilities. The model provides a dynamic forecast, constantly updating based on new data, ensuring timely and relevant predictions. Moreover, the model allows for sensitivity analysis, simulating different scenarios by adjusting input variables to assess their potential impact on the forecast. The results are presented in an easily understandable format, including detailed reports and interactive visualizations, allowing for informed decision-making. The model's performance is regularly monitored, with periodic reviews and refinements based on feedback and new data. The team is committed to maintaining and continuously enhancing the accuracy of the WRBY stock forecast model.
```
ML Model Testing
n:Time series to forecast
p:Price signals of Warby Parker stock
j:Nash equilibria (Neural Network)
k:Dominated move of Warby Parker stock holders
a:Best response for Warby Parker target price
For further technical information as per how our model work we invite you to visit the article below:
How do KappaSignal algorithms actually work?
Warby Parker Stock Forecast (Buy or Sell) Strategic Interaction Table
Strategic Interaction Table Legend:
X axis: *Likelihood% (The higher the percentage value, the more likely the event will occur.)
Y axis: *Potential Impact% (The higher the percentage value, the more likely the price will deviate.)
Z axis (Grey to Black): *Technical Analysis%
Warby Parker Inc. Financial Outlook and Forecast
WP's financial outlook reflects a company navigating the evolving landscape of the eyewear market. The company has demonstrated consistent revenue growth, driven by its direct-to-consumer model and expanding retail presence. However, the path to profitability has been more challenging, with WP incurring significant operating expenses related to customer acquisition, marketing, and expansion initiatives. Recent financial reports indicate a focus on improving operational efficiency and achieving profitability. This includes strategic investments in technology and supply chain optimization to reduce costs and enhance margins. Management's guidance suggests continued revenue growth, albeit at a potentially slower pace, alongside a commitment to achieving adjusted EBITDA profitability. The company's ability to manage its cost structure effectively and sustain its growth trajectory will be crucial to its financial performance in the coming periods.
The forecast for WP's financial performance hinges on several key factors. Firstly, the company's ability to successfully execute its retail expansion strategy is paramount. Opening new stores in strategic locations and driving comparable store sales growth are essential for revenue generation. Secondly, WP's digital presence remains a significant component of its business model. Continued investment in e-commerce platforms, website optimization, and targeted digital marketing campaigns will be necessary to maintain customer engagement and attract new customers. Moreover, WP's success will be determined by its ability to innovate in product offerings, expand into adjacent markets, and differentiate itself from competitors. The company must continue to provide stylish, high-quality eyewear at competitive prices, while also offering a seamless and convenient customer experience. Finally, market dynamics, including consumer spending patterns and potential economic downturns, will also impact the company's financial performance.
Analysis of WP's competitive environment provides further context for its financial outlook. The eyewear market is increasingly competitive, with established players, such as Luxottica, and other direct-to-consumer brands vying for market share. WP's competitive advantages lie in its brand recognition, strong customer loyalty, and innovative approach to the eyewear shopping experience. The company's direct-to-consumer model allows it to offer competitive pricing and provide a high level of customer service. However, WP faces the challenges of differentiating itself in a crowded market, retaining customer loyalty in an age of increased online competition, and navigating the complexities of supply chain management and inventory control. The success of WP will depend on its ability to effectively manage these competitive pressures, invest in brand building, and continue to enhance its customer value proposition.
Based on the current trajectory and market analysis, WP is expected to achieve improved financial performance in the next one to two years. The company is likely to see continued revenue growth, driven by retail expansion and e-commerce strength. The shift to profitability, though, may remain challenging. This forecast assumes that WP effectively manages its operational costs and drives improved margins. Risks include potential fluctuations in consumer spending, supply chain disruptions, and intensified competition. Moreover, macroeconomic factors, such as inflation and interest rate hikes, could negatively impact consumer spending and impede the company's ability to generate profits. Despite these risks, WP's strong brand and loyal customer base will enable it to manage its operating costs and achieve financial goals.
Rating | Short-Term | Long-Term Senior |
---|---|---|
Outlook | Ba3 | Ba3 |
Income Statement | Baa2 | B2 |
Balance Sheet | Baa2 | Baa2 |
Leverage Ratios | C | C |
Cash Flow | Ba3 | Ba3 |
Rates of Return and Profitability | B3 | Baa2 |
*Financial analysis is the process of evaluating a company's financial performance and position by neural network. It involves reviewing the company's financial statements, including the balance sheet, income statement, and cash flow statement, as well as other financial reports and documents.
How does neural network examine financial reports and understand financial state of the company?
References
- Dimakopoulou M, Athey S, Imbens G. 2017. Estimation considerations in contextual bandits. arXiv:1711.07077 [stat.ML]
- Tibshirani R, Hastie T. 1987. Local likelihood estimation. J. Am. Stat. Assoc. 82:559–67
- Hastie T, Tibshirani R, Wainwright M. 2015. Statistical Learning with Sparsity: The Lasso and Generalizations. New York: CRC Press
- Semenova V, Goldman M, Chernozhukov V, Taddy M. 2018. Orthogonal ML for demand estimation: high dimensional causal inference in dynamic panels. arXiv:1712.09988 [stat.ML]
- Mikolov T, Sutskever I, Chen K, Corrado GS, Dean J. 2013b. Distributed representations of words and phrases and their compositionality. In Advances in Neural Information Processing Systems, Vol. 26, ed. Z Ghahramani, M Welling, C Cortes, ND Lawrence, KQ Weinberger, pp. 3111–19. San Diego, CA: Neural Inf. Process. Syst. Found.
- Candès E, Tao T. 2007. The Dantzig selector: statistical estimation when p is much larger than n. Ann. Stat. 35:2313–51
- Arora S, Li Y, Liang Y, Ma T. 2016. RAND-WALK: a latent variable model approach to word embeddings. Trans. Assoc. Comput. Linguist. 4:385–99