AUC Score :
Short-term Tactic1 :
Dominant Strategy :
Time series to forecast n:
ML Model Testing : Modular Neural Network (News Feed Sentiment Analysis)
Hypothesis Testing : Spearman Correlation
Surveillance : Major exchange and OTC
1Short-term revised.
2Time series is updated based on short-term trends.
Key Points
The Dow Jones U.S. Select Insurance Index is anticipated to experience moderate growth, driven by rising interest rates which will bolster investment income for insurance companies, coupled with steady demand for insurance products amid a stable economic environment. However, the index faces several risks. A significant economic downturn or recession could negatively impact demand for certain insurance products, and lead to increased claims, straining profitability. Increased frequency of severe weather events or natural disasters could lead to substantial claims payouts, eroding profits and potentially triggering a decline. Further, regulatory changes, particularly in areas like pricing or capital requirements, could introduce uncertainty and weigh on investor sentiment and intensified competition within the insurance sector, including from Insurtech companies, may compress margins, limiting growth.About Dow Jones U.S. Select Insurance Index
The Dow Jones U.S. Select Insurance Index is a market capitalization-weighted index designed to measure the performance of the U.S. insurance sector. It encompasses companies primarily involved in providing various types of insurance products and services, including property and casualty, life, and health insurance. This index serves as a benchmark for investors seeking exposure to the insurance industry, offering a comprehensive view of the sector's overall health and trends. The index's composition is regularly reviewed to ensure it accurately reflects the evolving landscape of the U.S. insurance market and to maintain its relevance as a reliable investment tool.
The index methodology considers factors such as market capitalization and liquidity to determine the inclusion and weighting of individual companies. This ensures that larger and more actively traded firms have a more significant impact on the index's performance. Investors and analysts frequently utilize the Dow Jones U.S. Select Insurance Index to assess industry-specific risks, evaluate investment strategies, and track the performance of insurance-focused exchange-traded funds (ETFs). The index provides valuable insights into the financial health and growth prospects of the insurance sector within the broader U.S. economy.
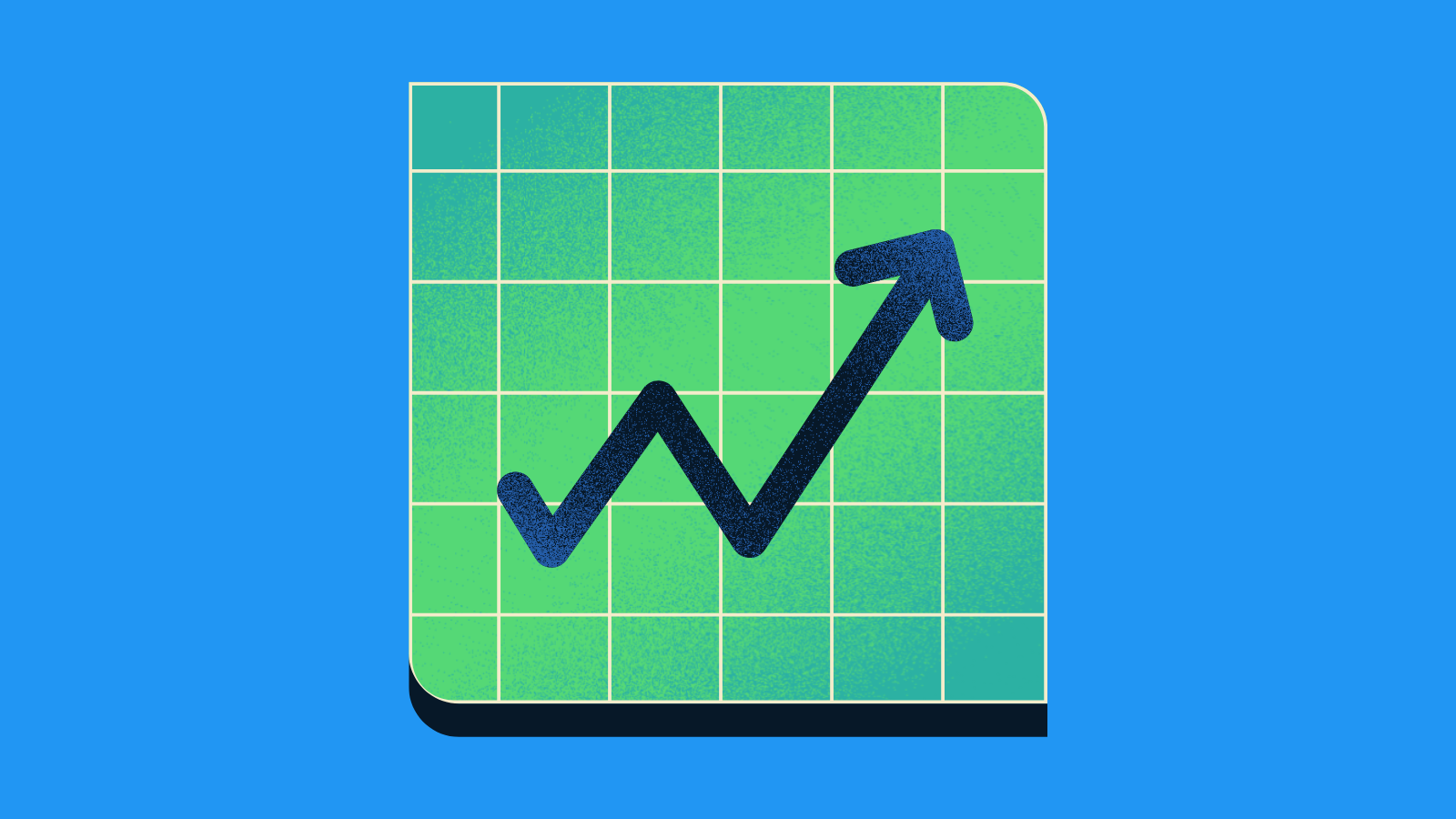
Machine Learning Model for Dow Jones U.S. Select Insurance Index Forecast
Our team of data scientists and economists has developed a robust machine learning model for forecasting the Dow Jones U.S. Select Insurance Index. The core of our model is a hybrid approach, combining the strengths of both time series analysis and machine learning algorithms. We begin by gathering a comprehensive dataset encompassing historical index values, financial ratios specific to the insurance sector (e.g., combined ratio, loss ratio, premiums written), macroeconomic indicators (e.g., interest rates, inflation, GDP growth), and market sentiment data. This data is meticulously preprocessed to handle missing values, outliers, and ensure data consistency. Feature engineering is a critical step, where we create lagged variables, rolling statistics (e.g., moving averages, standard deviations), and interaction terms to capture temporal dependencies and non-linear relationships. The model then incorporates both Recurrent Neural Networks (RNNs), particularly Long Short-Term Memory (LSTM) networks, and gradient boosting algorithms such as XGBoost and LightGBM. The RNNs are adept at capturing the time-series dynamics, while the gradient boosting methods handle complex feature interactions and non-linear relationships, enhancing predictive power. The models are trained using backtesting and cross validation.
The model's architecture is designed to leverage the diverse information streams effectively. The LSTM network is trained on the time series data to capture temporal dependencies. A separate branch of the model utilizes the financial and macroeconomic data. The output from these two branches are then combined in a final layer, where a gradient boosting algorithm is employed. We employ a careful selection of hyperparameters for each component, optimized through a grid search and cross-validation on historical data. The model's performance is evaluated using multiple metrics, including Mean Absolute Error (MAE), Root Mean Squared Error (RMSE), and directional accuracy. Regularization techniques are employed to prevent overfitting, and the model is continuously retrained and updated with new data to maintain its predictive accuracy. Model interpretability is also considered important to enhance the understanding of factors influence to index prices.
To ensure the model's practical application and reliability, we have incorporated several features. We utilize a rolling window approach, where the model is periodically retrained with the most recent data to account for market dynamics. We incorporate a risk assessment component that estimates the uncertainty associated with each forecast, providing a confidence interval. The model's output is presented in a user-friendly dashboard that visualizes the index forecast, confidence intervals, and key drivers of the forecast. Finally, we perform thorough backtesting and stress-testing the model by simulating various market scenarios and economic shocks. The model is intended to be used as one component within a larger investment strategy, providing valuable insights to financial decisions. The model output will be frequently revised and updated to include new data and to accommodate changes in the market.
ML Model Testing
n:Time series to forecast
p:Price signals of Dow Jones U.S. Select Insurance index
j:Nash equilibria (Neural Network)
k:Dominated move of Dow Jones U.S. Select Insurance index holders
a:Best response for Dow Jones U.S. Select Insurance target price
For further technical information as per how our model work we invite you to visit the article below:
How do KappaSignal algorithms actually work?
Dow Jones U.S. Select Insurance Index Forecast Strategic Interaction Table
Strategic Interaction Table Legend:
X axis: *Likelihood% (The higher the percentage value, the more likely the event will occur.)
Y axis: *Potential Impact% (The higher the percentage value, the more likely the price will deviate.)
Z axis (Grey to Black): *Technical Analysis%
Dow Jones U.S. Select Insurance Index: Financial Outlook and Forecast
The Dow Jones U.S. Select Insurance Index, encompassing a diverse range of insurance companies operating within the United States, exhibits a financial outlook shaped by several interconnected factors. Interest rate movements play a critical role, as insurers invest substantial portions of their premiums in fixed-income securities. Rising interest rates, while potentially increasing investment income, can also lead to lower valuations of existing bond holdings. Furthermore, economic growth and consumer confidence significantly impact the index. A robust economy typically translates into increased insurance demand across various lines, including property and casualty, health, and life insurance. Conversely, economic downturns can depress demand, particularly for discretionary insurance products. Regulatory changes, such as those pertaining to capital requirements and consumer protection, also influence the index's performance, often necessitating adjustments to business models and risk management strategies. The index's composition, which heavily features established, financially sound companies, provides a degree of stability, yet the inherent cyclicality of the insurance industry introduces volatility.
Looking ahead, the forecast for the Dow Jones U.S. Select Insurance Index is subject to a complex interplay of variables. The evolving landscape of risk, including climate change impacts and cyber security threats, presents both challenges and opportunities. Insurance companies are actively developing new products and pricing strategies to address these emerging risks, potentially driving revenue growth. Technological advancements, particularly in data analytics and artificial intelligence, are expected to improve underwriting accuracy, claims processing efficiency, and customer service, further enhancing profitability. Consolidation activity within the sector, driven by a desire to achieve economies of scale and diversify product offerings, could reshape the competitive landscape. However, heightened inflation poses a significant concern. Rising costs associated with claims settlements, particularly in property and casualty lines, can erode profit margins if not adequately reflected in premium pricing. Furthermore, changes in healthcare regulations may significantly affect health insurance providers.
The long-term sustainability of the index's performance hinges on several key considerations. Insurers must adapt to evolving customer expectations and embrace digital transformation to maintain competitiveness. Investing in technologies that enhance customer experience and streamline operations will be paramount. Maintaining a strong focus on risk management, including diversification and sophisticated modeling techniques, is essential to navigate the unpredictable nature of catastrophic events and economic volatility. The ability of insurance companies to effectively manage capital, including maintaining adequate reserves and optimizing investment portfolios, will be critical in supporting financial strength. Finally, a commitment to ethical practices and regulatory compliance will be essential to maintain consumer trust and preserve the industry's reputation. Companies which can successfully address these factors are likely to outperform.
Considering these dynamics, the outlook for the Dow Jones U.S. Select Insurance Index leans toward a positive trajectory, albeit with potential fluctuations. Technological innovation and the sector's capacity to adapt to emerging risks create opportunities for long-term growth. However, several risks could undermine this positive outlook. Economic slowdown could diminish demand, particularly for discretionary insurance. Severe weather events and other catastrophic losses may lead to outsized claims, particularly in property and casualty insurance. Increased regulatory scrutiny, as well as rising inflation and costs, could put pressure on profitability. Moreover, cybersecurity breaches could expose insurers to significant financial losses. Success will depend on these firms' ability to navigate these risks while capitalizing on growth prospects.
```
Rating | Short-Term | Long-Term Senior |
---|---|---|
Outlook | Ba2 | Ba3 |
Income Statement | Baa2 | C |
Balance Sheet | C | B1 |
Leverage Ratios | Baa2 | Baa2 |
Cash Flow | Baa2 | B3 |
Rates of Return and Profitability | Baa2 | Baa2 |
*An aggregate rating for an index summarizes the overall sentiment towards the companies it includes. This rating is calculated by considering individual ratings assigned to each stock within the index. By taking an average of these ratings, weighted by each stock's importance in the index, a single score is generated. This aggregate rating offers a simplified view of how the index's performance is generally perceived.
How does neural network examine financial reports and understand financial state of the company?
References
- Lai TL, Robbins H. 1985. Asymptotically efficient adaptive allocation rules. Adv. Appl. Math. 6:4–22
- Y. Le Tallec. Robust, risk-sensitive, and data-driven control of Markov decision processes. PhD thesis, Massachusetts Institute of Technology, 2007.
- D. Bertsekas. Nonlinear programming. Athena Scientific, 1999.
- Chernozhukov V, Chetverikov D, Demirer M, Duflo E, Hansen C, et al. 2018a. Double/debiased machine learning for treatment and structural parameters. Econom. J. 21:C1–68
- Angrist JD, Pischke JS. 2008. Mostly Harmless Econometrics: An Empiricist's Companion. Princeton, NJ: Princeton Univ. Press
- Mnih A, Kavukcuoglu K. 2013. Learning word embeddings efficiently with noise-contrastive estimation. In Advances in Neural Information Processing Systems, Vol. 26, ed. Z Ghahramani, M Welling, C Cortes, ND Lawrence, KQ Weinberger, pp. 2265–73. San Diego, CA: Neural Inf. Process. Syst. Found.
- Vilnis L, McCallum A. 2015. Word representations via Gaussian embedding. arXiv:1412.6623 [cs.CL]