AUC Score :
Short-term Tactic1 :
Dominant Strategy :
Time series to forecast n:
ML Model Testing : Modular Neural Network (News Feed Sentiment Analysis)
Hypothesis Testing : Wilcoxon Rank-Sum Test
Surveillance : Major exchange and OTC
1Short-term revised.
2Time series is updated based on short-term trends.
Key Points
VTEX's Class A Common Shares are anticipated to experience moderate growth, driven by continued expansion in Latin America and increased adoption of its e-commerce platform by larger enterprise clients. The company's ability to maintain its technological edge and effectively compete with established players in the e-commerce space is key to this growth. Risks include slower-than-expected adoption rates in new markets, heightened competition from both domestic and international rivals, and potential economic downturns in its primary operating regions. A significant risk factor lies in VTEX's dependence on the Latin American market, which exposes it to regional economic instability and currency fluctuations. Moreover, further regulatory scrutiny and data security concerns could impact business.About VTEX Class A
VTEX (NYSE: VTEX) is a global enterprise digital commerce platform that provides a software-as-a-service (SaaS) solution for businesses to build, manage, and run their online stores. The company offers a comprehensive suite of tools, including e-commerce, marketplace, and order management functionalities, aimed at facilitating online sales and improving customer experience. VTEX primarily serves large and medium-sized enterprises across various industries, focusing on clients who require scalable and flexible e-commerce capabilities. Their platform is designed to support multiple storefronts, currencies, and languages, catering to businesses with international ambitions.
Founded in Brazil, VTEX has expanded its operations globally, with a significant presence in Latin America, Europe, and North America. The company differentiates itself through its cloud-based architecture, which allows for easier integration with other systems and faster deployment of new features. VTEX emphasizes a collaborative ecosystem, integrating with various third-party service providers to provide businesses with a complete and adaptable e-commerce solution. Their business model is subscription-based, providing ongoing services and support to their customers.
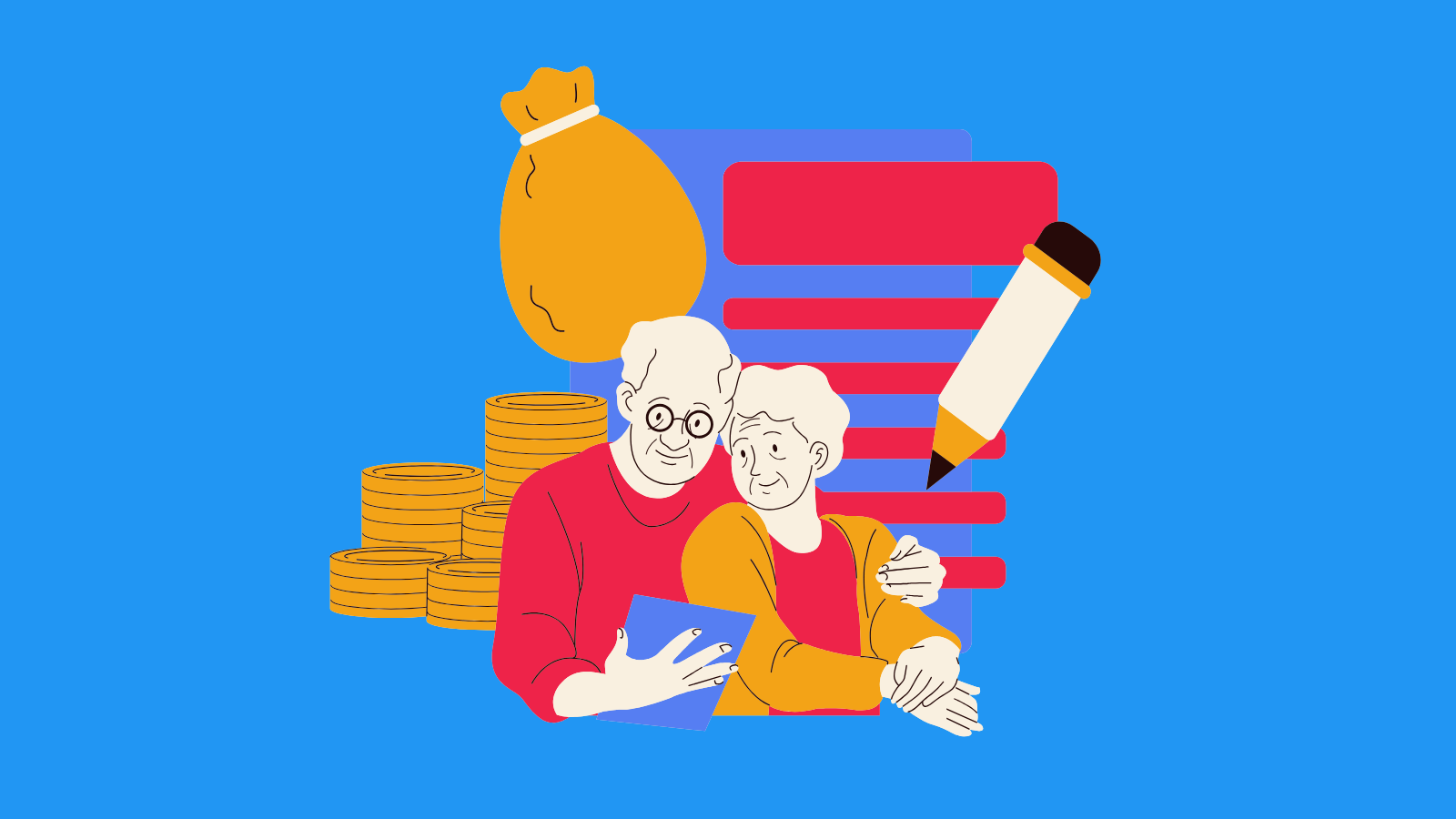
VTEX (VTEX) Class A Common Shares Stock Forecast Model
Our data science and economics team proposes a machine learning model for forecasting VTEX Class A Common Shares. The core of our approach involves a hybrid model combining time-series analysis and machine learning techniques. We will leverage a robust dataset encompassing historical price movements, encompassing volume and volatility, and relevant macroeconomic indicators. This data will be sourced from reputable financial data providers. For time-series analysis, we will implement models such as ARIMA (Autoregressive Integrated Moving Average) and its variants to capture the patterns and seasonality inherent in the stock's past performance. Simultaneously, we'll incorporate external factors, including industry-specific news, broader market trends (S&P 500, NASDAQ Composite), and VTEX's financial reports (earnings, revenue growth, customer acquisition metrics). These factors will be used in machine-learning models like Gradient Boosting Machines (GBM) and Recurrent Neural Networks (RNN) to capture non-linear relationships that the time series models may miss.
The model's architecture will be built with modularity in mind. The time-series and machine-learning components will work in conjunction. We'll initially preprocess and clean the data to handle missing values and outliers. Feature engineering will be crucial, and we'll construct technical indicators (Moving Averages, RSI, MACD) from price data, and derive sentiment scores from news articles. To improve performance, our model will have a feature importance analysis step to identify the most relevant drivers of VTEX share performance. We will employ a cross-validation strategy to validate the model, ensuring that the model is reliable. Model outputs will be generated using the time series and machine learning models, incorporating an ensemble approach. This will then generate a forecast that considers both historical patterns and the impact of various factors. The final forecast will then show a probability of a bullish, bearish, or neutral outlook for the stock.
Model performance will be evaluated using standard metrics such as Mean Absolute Error (MAE), Mean Squared Error (MSE), and directional accuracy. Regular model retraining and fine-tuning, employing new data and adjusting parameters based on market dynamics, are essential. We will continually analyze the market, VTEX performance, and adjust the inputs of the model accordingly. We will also set up automatic alerts and monitoring systems to track model performance. Our team will ensure the model remains competitive and reflects the most recent data inputs. Model results will be visualized for easier interpretation and provide actionable insights for stakeholders, enabling data-driven decisions regarding investment strategies. The model will also provide risk assessments. This approach will provide forecasts and insights into the risk factors.
```
ML Model Testing
n:Time series to forecast
p:Price signals of VTEX Class A stock
j:Nash equilibria (Neural Network)
k:Dominated move of VTEX Class A stock holders
a:Best response for VTEX Class A target price
For further technical information as per how our model work we invite you to visit the article below:
How do KappaSignal algorithms actually work?
VTEX Class A Stock Forecast (Buy or Sell) Strategic Interaction Table
Strategic Interaction Table Legend:
X axis: *Likelihood% (The higher the percentage value, the more likely the event will occur.)
Y axis: *Potential Impact% (The higher the percentage value, the more likely the price will deviate.)
Z axis (Grey to Black): *Technical Analysis%
VTEX Class A Common Shares: Financial Outlook and Forecast
VTEX's financial outlook is currently influenced by a combination of factors specific to the e-commerce platform provider. The company has demonstrated consistent revenue growth, particularly in Latin America, which represents a significant portion of its business. This growth is driven by the increasing adoption of e-commerce across the region and VTEX's ability to attract and retain enterprise clients. Additionally, VTEX's emphasis on its composable commerce platform and its continued investment in product development positions it favorably to capitalize on evolving market demands. However, the company faces challenges that could impact its financial performance, including competition from established and emerging players in the e-commerce software market. This competition, combined with fluctuations in the global economy, could influence pricing pressures and potentially impact VTEX's expansion plans.
Future growth is expected to be fueled by several key trends. The global expansion of e-commerce presents a substantial opportunity, and VTEX's presence in multiple markets allows it to capture a portion of this growth. Furthermore, VTEX's focus on enterprise clients is significant. These clients typically generate larger revenue streams and longer customer lifecycles. Success in this segment depends on VTEX's capability to offer solutions that meet the complex requirements of large retailers and brands. Furthermore, the expansion of its product offerings, like payment and fulfillment solutions, is expected to strengthen its position within the e-commerce ecosystem and drive growth. The company's ability to integrate with other software providers and platforms is critical for its success in attracting and retaining clients. Maintaining competitiveness will also depend on continued innovation in its product offerings.
The company's financial forecast is predicated on its ability to achieve several critical objectives. These include the continued expansion of its customer base, especially within the enterprise segment. VTEX's capacity to maintain competitive pricing while upholding its commitment to profitability is also vital for achieving its financial goals. Furthermore, the efficient management of operating expenses, particularly in areas like sales and marketing, and research and development, is crucial for improving profitability. Revenue growth depends on the success of its composable commerce platform, which allows clients to adapt to specific needs. VTEX's capacity to adapt to change and capitalize on emerging technologies like Artificial Intelligence and to manage currency fluctuations, especially in Latin America, is important to drive sustainable growth.
Based on the current market dynamics and VTEX's strategic initiatives, a positive outlook is predicted. The company's focus on enterprise clients, geographical diversification, and its innovative product roadmap, support the prospects of future growth. The most significant risks associated with this outlook include intensified competition from e-commerce giants and the impact of economic downturns on consumer spending, especially in developing markets. Currency fluctuations in Latin America and the broader global economy also pose risks to VTEX's financial performance. Furthermore, VTEX's capacity to swiftly integrate new technologies and adapt to market trends will determine the success of its position in the market.
Rating | Short-Term | Long-Term Senior |
---|---|---|
Outlook | Ba3 | Ba1 |
Income Statement | Baa2 | Baa2 |
Balance Sheet | Ba3 | Caa2 |
Leverage Ratios | B2 | Baa2 |
Cash Flow | Baa2 | B1 |
Rates of Return and Profitability | Caa2 | Ba1 |
*Financial analysis is the process of evaluating a company's financial performance and position by neural network. It involves reviewing the company's financial statements, including the balance sheet, income statement, and cash flow statement, as well as other financial reports and documents.
How does neural network examine financial reports and understand financial state of the company?
References
- Chen X. 2007. Large sample sieve estimation of semi-nonparametric models. In Handbook of Econometrics, Vol. 6B, ed. JJ Heckman, EE Learner, pp. 5549–632. Amsterdam: Elsevier
- Cortes C, Vapnik V. 1995. Support-vector networks. Mach. Learn. 20:273–97
- Allen, P. G. (1994), "Economic forecasting in agriculture," International Journal of Forecasting, 10, 81–135.
- Hastie T, Tibshirani R, Tibshirani RJ. 2017. Extended comparisons of best subset selection, forward stepwise selection, and the lasso. arXiv:1707.08692 [stat.ME]
- Keane MP. 2013. Panel data discrete choice models of consumer demand. In The Oxford Handbook of Panel Data, ed. BH Baltagi, pp. 54–102. Oxford, UK: Oxford Univ. Press
- V. Borkar. An actor-critic algorithm for constrained Markov decision processes. Systems & Control Letters, 54(3):207–213, 2005.
- Jacobs B, Donkers B, Fok D. 2014. Product Recommendations Based on Latent Purchase Motivations. Rotterdam, Neth.: ERIM