AUC Score :
Short-term Tactic1 :
Dominant Strategy :
Time series to forecast n:
ML Model Testing : Inductive Learning (ML)
Hypothesis Testing : Stepwise Regression
Surveillance : Major exchange and OTC
1Short-term revised.
2Time series is updated based on short-term trends.
Key Points
The Dow Jones U.S. Utilities index is expected to experience a period of moderate growth, fueled by increasing demand for essential services and the ongoing transition to renewable energy sources. This growth will likely be somewhat tempered by rising interest rates, which could increase borrowing costs for utilities and potentially impact their profitability. Further, regulatory uncertainties surrounding carbon emissions and infrastructure investments may pose additional challenges, potentially leading to market volatility. Conversely, favorable government policies supporting clean energy initiatives and robust dividend yields could attract investors and support overall stability in the sector.About Dow Jones U.S. Utilities Index
The Dow Jones U.S. Utilities Index is a market capitalization-weighted index designed to track the performance of the utility sector within the United States. It serves as a benchmark for investors seeking exposure to companies that provide essential services such as electricity, natural gas, and water. These companies are typically characterized by relatively stable earnings and dividends, making the index attractive to investors seeking income and a degree of defensive characteristics in their portfolio.
The index's composition is periodically reviewed and rebalanced to reflect changes in the market and industry. The Dow Jones U.S. Utilities Index offers a means to gauge the overall health and performance of the utility sector, enabling investors to compare their portfolio performance with the broader sector trends. It is a widely followed tool used by financial analysts and portfolio managers to assess the investment opportunities within the utilities industry.
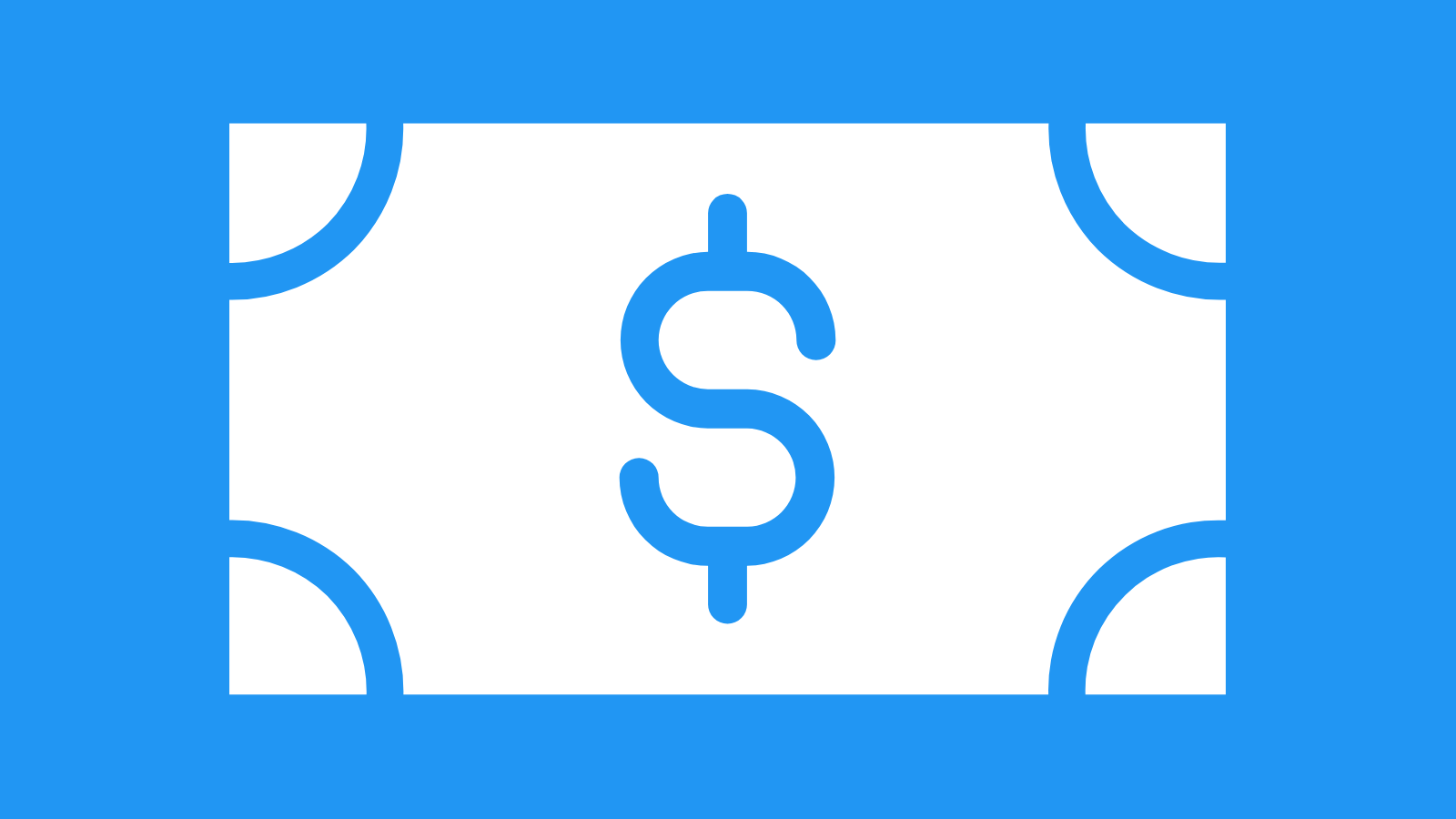
Machine Learning Model for Dow Jones U.S. Utilities Index Forecast
The construction of a robust forecasting model for the Dow Jones U.S. Utilities Index necessitates a comprehensive approach that integrates both financial and macroeconomic variables. Our data science team will employ a hybrid methodology, combining time series analysis with machine learning techniques. Initially, a deep understanding of the index's historical behavior is crucial. We will utilize Autoregressive Integrated Moving Average (ARIMA) models and its variants to capture the inherent temporal dependencies within the index's returns, acknowledging seasonality and trends. Crucially, we will also investigate the impacts of economic indicators like inflation rates, interest rate fluctuations (Federal Reserve decisions), changes in energy prices (natural gas, coal), and regulatory environments on the utilities sector. These macroeconomic factors will serve as external drivers, enhancing the model's predictive capabilities.
To enhance predictive accuracy beyond the capabilities of simple time series methods, we will incorporate machine learning algorithms, such as Recurrent Neural Networks (RNNs), specifically LSTMs (Long Short-Term Memory), designed to handle sequential data effectively. Furthermore, we consider the use of Ensemble methods (Random Forest, Gradient Boosting) to leverage the strengths of multiple models. These algorithms will be trained on historical index data, along with macroeconomic factors and fundamental financial ratios of prominent utility companies within the index. Feature engineering will be a critical aspect, involving lag variables, rolling statistics, and the creation of composite indicators to extract relevant signals. To mitigate the risk of overfitting, cross-validation techniques will be employed, ensuring the model generalizes well to unseen data.
The final model will provide forecasts for the Dow Jones U.S. Utilities Index. Regular model performance evaluation is of paramount importance. Key metrics will include Mean Absolute Error (MAE), Root Mean Squared Error (RMSE), and directional accuracy. The model's performance will be assessed against a baseline model (e.g., a simple random walk) and established benchmarks. The model will be continuously monitored and re-trained with new data to maintain predictive validity. Furthermore, we will conduct sensitivity analysis to determine the relative importance of different input variables, offering insights into the drivers of index performance and aiding in risk management within the utilities sector.
ML Model Testing
n:Time series to forecast
p:Price signals of Dow Jones U.S. Utilities index
j:Nash equilibria (Neural Network)
k:Dominated move of Dow Jones U.S. Utilities index holders
a:Best response for Dow Jones U.S. Utilities target price
For further technical information as per how our model work we invite you to visit the article below:
How do KappaSignal algorithms actually work?
Dow Jones U.S. Utilities Index Forecast Strategic Interaction Table
Strategic Interaction Table Legend:
X axis: *Likelihood% (The higher the percentage value, the more likely the event will occur.)
Y axis: *Potential Impact% (The higher the percentage value, the more likely the price will deviate.)
Z axis (Grey to Black): *Technical Analysis%
Dow Jones U.S. Utilities Index: Financial Outlook and Forecast
The Dow Jones U.S. Utilities Index, representing a sector critical to the American economy, faces a mixed outlook characterized by both opportunities and challenges. The industry, comprised of companies providing essential services like electricity, natural gas, and water, tends to be considered a relatively defensive sector due to the consistent demand for its products regardless of economic cycles. This inherent stability makes it a potential haven for investors seeking lower volatility and consistent dividend yields. However, the sector is simultaneously undergoing a significant transformation driven by factors like the ongoing shift towards renewable energy sources, evolving regulatory landscapes, and the need for substantial infrastructure investments. These forces will significantly shape the financial performance of utilities in the coming years.
The financial forecast for the utilities sector is heavily influenced by governmental policies and the evolution of energy markets. The increasing emphasis on environmental sustainability, driven by both public and private sector initiatives, is fueling substantial investment in renewable energy infrastructure, including solar, wind, and battery storage. This transition requires significant capital expenditure, potentially leading to increased debt levels and the need to raise rates to fund projects. Furthermore, utilities are grappling with regulatory complexities, varying from state to state, and the pace of approvals for new infrastructure projects can be slow. The growth of distributed generation, like rooftop solar, also poses a challenge to traditional business models, demanding adaptation and innovation from utility companies. Companies successful in managing capital allocation, adapting to the changing energy mix, and navigating regulatory complexities will likely experience more robust financial results.
The growth potential for the Dow Jones U.S. Utilities Index is reliant on several factors. Population growth within service territories will directly impact demand. Economic expansion, leading to increased industrial and commercial activity, will also drive electricity consumption. Further, utilities companies are also investing heavily in smart grid technologies to improve efficiency, enhance reliability, and enable better demand-side management. This investment can boost profitability. Moreover, many utilities offer attractive dividend yields, appealing to income-seeking investors. However, the industry faces inherent risks. Interest rate fluctuations represent a significant risk factor, because utilities' capital-intensive nature makes them especially vulnerable to rising borrowing costs. Unexpected changes in regulation, such as revisions to rate structures or environmental compliance standards, can also negatively impact profitability.
Overall, the outlook for the Dow Jones U.S. Utilities Index is cautiously optimistic. The shift toward cleaner energy, combined with the underlying demand for essential services, supports long-term growth. We predict that the index will see modest but consistent growth in the coming years, driven by infrastructure investments, demographic trends, and the stable nature of demand. However, this prediction comes with several risks. Increased interest rates, delays in project approvals, and unpredictable regulatory changes could significantly affect financial performance. Additionally, the success of this sector depends on the effective management of the transition towards renewables, and the companies' ability to navigate the complexities of a changing energy landscape. Investors should thus carefully consider the sector's inherent characteristics, potential challenges, and the ability of individual utilities to adapt and innovate.
Rating | Short-Term | Long-Term Senior |
---|---|---|
Outlook | B1 | B2 |
Income Statement | Baa2 | B1 |
Balance Sheet | C | C |
Leverage Ratios | Caa2 | B1 |
Cash Flow | Ba2 | C |
Rates of Return and Profitability | Ba3 | Baa2 |
*An aggregate rating for an index summarizes the overall sentiment towards the companies it includes. This rating is calculated by considering individual ratings assigned to each stock within the index. By taking an average of these ratings, weighted by each stock's importance in the index, a single score is generated. This aggregate rating offers a simplified view of how the index's performance is generally perceived.
How does neural network examine financial reports and understand financial state of the company?
References
- Harris ZS. 1954. Distributional structure. Word 10:146–62
- Athey S, Bayati M, Doudchenko N, Imbens G, Khosravi K. 2017a. Matrix completion methods for causal panel data models. arXiv:1710.10251 [math.ST]
- Jorgenson, D.W., Weitzman, M.L., ZXhang, Y.X., Haxo, Y.M. and Mat, Y.X., 2023. Can Neural Networks Predict Stock Market?. AC Investment Research Journal, 220(44).
- Athey S. 2017. Beyond prediction: using big data for policy problems. Science 355:483–85
- Dudik M, Langford J, Li L. 2011. Doubly robust policy evaluation and learning. In Proceedings of the 28th International Conference on Machine Learning, pp. 1097–104. La Jolla, CA: Int. Mach. Learn. Soc.
- Chow, G. C. (1960), "Tests of equality between sets of coefficients in two linear regressions," Econometrica, 28, 591–605.
- D. Bertsekas. Min common/max crossing duality: A geometric view of conjugacy in convex optimization. Lab. for Information and Decision Systems, MIT, Tech. Rep. Report LIDS-P-2796, 2009