AUC Score :
Short-term Tactic1 :
Dominant Strategy :
Time series to forecast n:
ML Model Testing : Multi-Task Learning (ML)
Hypothesis Testing : Chi-Square
Surveillance : Major exchange and OTC
1Short-term revised.
2Time series is updated based on short-term trends.
Key Points
TEGNA may experience moderate growth driven by its broadcast television stations and digital media initiatives. The company's ability to adapt to evolving advertising trends and maintain strong local content offerings will be crucial for sustained performance. Strategic acquisitions and partnerships could enhance its market position. However, challenges exist, including fluctuations in advertising revenue due to economic cycles and competition from streaming services. Increased content costs and potential cord-cutting trends pose risks, along with the regulatory environment for media ownership. The company's debt load and the ability to effectively manage its diverse portfolio will influence its financial stability. Overall, the outlook appears cautiously optimistic, contingent on effective execution and navigating industry dynamics.About TEGNA
TEGNA Inc. is a prominent media company operating across the United States. The company owns and operates a diverse portfolio of television stations, primarily focused on local news, entertainment, and sports programming. TEGNA's television stations reach a significant portion of the American population and are key providers of local news and information in their respective markets. Beyond television broadcasting, TEGNA also engages in digital media initiatives, including online platforms and mobile applications, to distribute its content and engage with audiences across various digital channels.
The company emphasizes providing quality journalism and community-focused content. TEGNA has a strong commitment to local news coverage and aims to serve as a trusted source of information for the communities it serves. Additionally, TEGNA is involved in other initiatives, such as the operation of marketing and advertising services to support local businesses. Its primary focus is on creating value for its viewers, advertisers, and stakeholders by leveraging its assets and strategic partnerships.
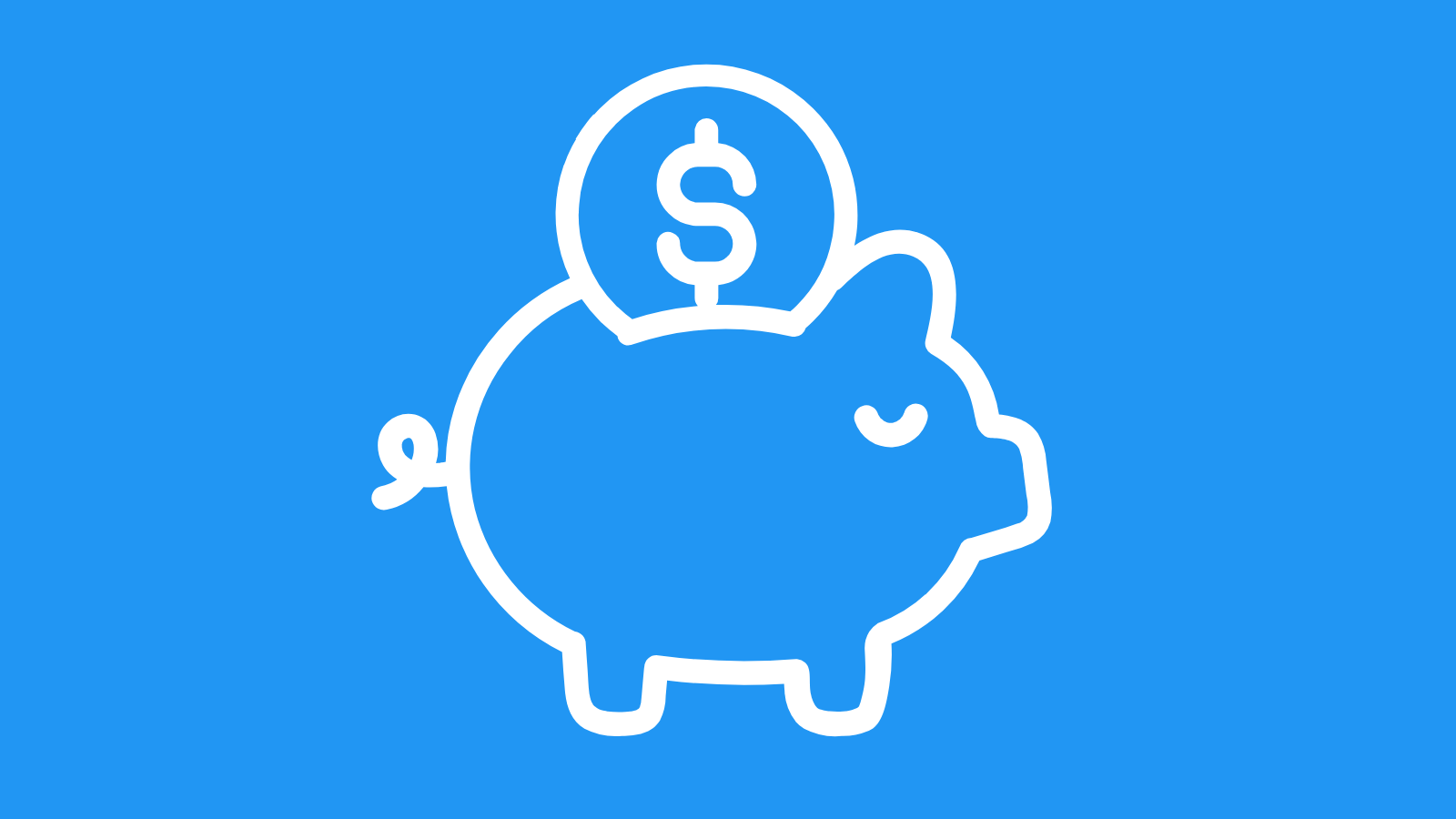
TGNA Stock Forecast Machine Learning Model
Our approach to forecasting TEGNA Inc (TGNA) stock performance involves the development of a sophisticated machine learning model. This model will integrate a diverse set of economic and financial indicators. We'll begin by gathering a comprehensive dataset, including historical stock prices, trading volumes, and relevant financial statements such as revenue, earnings per share, and debt levels. Concurrently, we will incorporate macroeconomic factors, including inflation rates, interest rates, GDP growth, and industry-specific indicators, such as advertising revenue trends. The selection of these indicators will be informed by rigorous statistical analysis, correlation studies, and expert economic judgment to ensure that the model captures the key drivers of TGNA's stock price fluctuations. The data will be cleaned, preprocessed, and transformed to address missing values, outliers, and scale differences to ensure the data is ready for the models.
The core of our model will be a combination of several machine learning algorithms to enhance prediction accuracy. We will explore the use of various models, including but not limited to: Recurrent Neural Networks (RNNs) such as Long Short-Term Memory (LSTM) networks, which are well-suited for time series data; Gradient Boosting algorithms like XGBoost, which excels at handling complex relationships; and possibly Support Vector Machines (SVMs) to create a robust and efficient model. The choice of algorithms will be based on their ability to capture complex patterns in the data. The ensemble of models will be trained, validated, and tested using a robust methodology including train, validation, and test datasets, to evaluate and compare the performance of various algorithms. We'll also implement cross-validation techniques to assess model stability and reduce the risk of overfitting.
Model performance will be rigorously evaluated using various metrics. We will measure the model's predictive power using Mean Absolute Error (MAE), Root Mean Squared Error (RMSE), and the R-squared value. Furthermore, we'll incorporate techniques for risk assessment to assess the uncertainty of our forecasts. This includes backtesting the model's performance against historical data and examining its sensitivity to different market conditions. The model's output will not only provide a point forecast for TGNA's stock performance but will also provide confidence intervals to estimate the range of potential outcomes. Continuous monitoring, evaluation, and model refinement will be implemented to ensure the accuracy and reliability of our forecasts, and the model will be adapted based on the analysis results.
ML Model Testing
n:Time series to forecast
p:Price signals of TEGNA stock
j:Nash equilibria (Neural Network)
k:Dominated move of TEGNA stock holders
a:Best response for TEGNA target price
For further technical information as per how our model work we invite you to visit the article below:
How do KappaSignal algorithms actually work?
TEGNA Stock Forecast (Buy or Sell) Strategic Interaction Table
Strategic Interaction Table Legend:
X axis: *Likelihood% (The higher the percentage value, the more likely the event will occur.)
Y axis: *Potential Impact% (The higher the percentage value, the more likely the price will deviate.)
Z axis (Grey to Black): *Technical Analysis%
TEGNA Inc. Financial Outlook and Forecast
TEGNA's financial trajectory for the upcoming period presents a complex landscape shaped by evolving media consumption habits, fluctuating advertising revenue, and strategic business decisions. The company is actively navigating a dynamic media environment, focusing on strengthening its digital presence, investing in high-quality content, and optimizing its operational efficiency. The company is expected to continue investing in its digital platforms, including its over-the-top (OTT) offerings, and expand its local news and information offerings. This strategic shift aims to capture a growing audience segment that increasingly relies on digital platforms for news and entertainment. Furthermore, the company will likely prioritize cost management and operational efficiency to maintain profitability in the face of potential revenue volatility, including focusing on targeted acquisitions. This comprehensive approach positions the company to adapt to changing market demands and mitigate potential financial challenges, setting a foundation for sustainable growth in the long term.
Advertising revenue, a crucial component of TEGNA's financial performance, will be subject to both cyclical and secular pressures. Local advertising, traditionally a significant revenue source, could face headwinds from broader economic uncertainties and increased competition from digital platforms. The performance of the political advertising market, tied to election cycles, also substantially influences TEGNA's revenues, creating significant fluctuations from year to year. The company's digital advertising revenue growth is a key factor in offsetting the potential decline in traditional advertising. The company aims to leverage its local content and audience reach to attract digital advertising dollars and establish new revenue streams, including subscriptions and content licensing, to diversify its revenue base and reduce its reliance on advertising alone. This strategy is critical for the company's financial health.
Strategic initiatives, including acquisitions and divestitures, significantly shape TEGNA's financial outlook. Strategic acquisitions of local television stations or digital media assets can enhance its market presence and boost its revenue-generating potential. Divestitures of certain assets could be undertaken to improve cash flow or reshape its portfolio. The company is expected to continue optimizing its portfolio, including its programming and content, which involves assessing the viability of certain programs and content to ensure maximum return on investment. The company could undertake strategic partnerships and collaborations to enhance its content offerings, expand its audience reach, and strengthen its competitive position. Such moves could include partnerships with streaming platforms or content creators. The effectiveness of these initiatives will directly influence TEGNA's overall financial results and its ability to achieve its strategic goals.
The overall outlook for TEGNA is cautiously optimistic, with expected long-term revenue growth, driven by the company's ability to expand its digital footprint, adapt to changing consumer preferences, and optimize its operations. The main risks associated with this outlook include the fluctuations in advertising revenue, the pressure from the traditional market, and the evolving digital media landscape. The company's ability to successfully execute its strategic initiatives, effectively integrate any new acquisitions, and navigate the complexities of the media industry will play a crucial role in determining its ultimate financial success. Despite these risks, the company's proactive approach to innovation and its focus on audience engagement create the potential for substantial growth and value creation for shareholders over the long term.
Rating | Short-Term | Long-Term Senior |
---|---|---|
Outlook | B1 | B3 |
Income Statement | B1 | Caa2 |
Balance Sheet | Baa2 | B1 |
Leverage Ratios | Caa2 | Caa2 |
Cash Flow | Ba3 | C |
Rates of Return and Profitability | B2 | Caa2 |
*Financial analysis is the process of evaluating a company's financial performance and position by neural network. It involves reviewing the company's financial statements, including the balance sheet, income statement, and cash flow statement, as well as other financial reports and documents.
How does neural network examine financial reports and understand financial state of the company?
References
- Chow, G. C. (1960), "Tests of equality between sets of coefficients in two linear regressions," Econometrica, 28, 591–605.
- Chen X. 2007. Large sample sieve estimation of semi-nonparametric models. In Handbook of Econometrics, Vol. 6B, ed. JJ Heckman, EE Learner, pp. 5549–632. Amsterdam: Elsevier
- Athey S, Bayati M, Doudchenko N, Imbens G, Khosravi K. 2017a. Matrix completion methods for causal panel data models. arXiv:1710.10251 [math.ST]
- C. Szepesvári. Algorithms for Reinforcement Learning. Synthesis Lectures on Artificial Intelligence and Machine Learning. Morgan & Claypool Publishers, 2010
- Chow, G. C. (1960), "Tests of equality between sets of coefficients in two linear regressions," Econometrica, 28, 591–605.
- Bottomley, P. R. Fildes (1998), "The role of prices in models of innovation diffusion," Journal of Forecasting, 17, 539–555.
- Chernozhukov V, Chetverikov D, Demirer M, Duflo E, Hansen C, et al. 2018a. Double/debiased machine learning for treatment and structural parameters. Econom. J. 21:C1–68