AUC Score :
Short-term Tactic1 :
Dominant Strategy :
Time series to forecast n:
ML Model Testing : Active Learning (ML)
Hypothesis Testing : Multiple Regression
Surveillance : Major exchange and OTC
1Short-term revised.
2Time series is updated based on short-term trends.
Key Points
Predictions for Beamr Imaging's stock include potential growth driven by increasing demand for video optimization solutions, particularly in the streaming and broadcast sectors, alongside strategic partnerships that could expand market reach. The company's innovative technology, if widely adopted, could lead to increased revenue and profitability. However, significant risks are associated with these predictions. Competition from established players in the media technology space poses a constant challenge. The company's ability to secure and maintain crucial contracts is vital for success, and any delays or failures would negatively impact the stock. Further dilution through future funding rounds and the overall economic environment, which can influence media consumption trends, present additional areas of concern.About Beamr Imaging
Beamr Imaging Ltd. develops and licenses video optimization technology for the media and entertainment industries. The company's core technology focuses on improving video compression efficiency while maintaining or enhancing visual quality. This allows for significant reductions in file size, bandwidth consumption, and storage costs. Beamr's solutions are applicable across various video formats and are designed to be integrated into content creation, distribution, and playback workflows. Their products are aimed at broadcasters, content creators, and streaming platforms.
Beamr's business model revolves around licensing its technology and providing related services, including integration support and custom development. The company seeks to address the growing demand for efficient video delivery driven by the increasing volume of video content and the prevalence of high-resolution formats like 4K and 8K. Its technology is positioned to optimize video across multiple platforms, thus providing benefits to both content providers and consumers by improving streaming quality and reducing bandwidth usage.
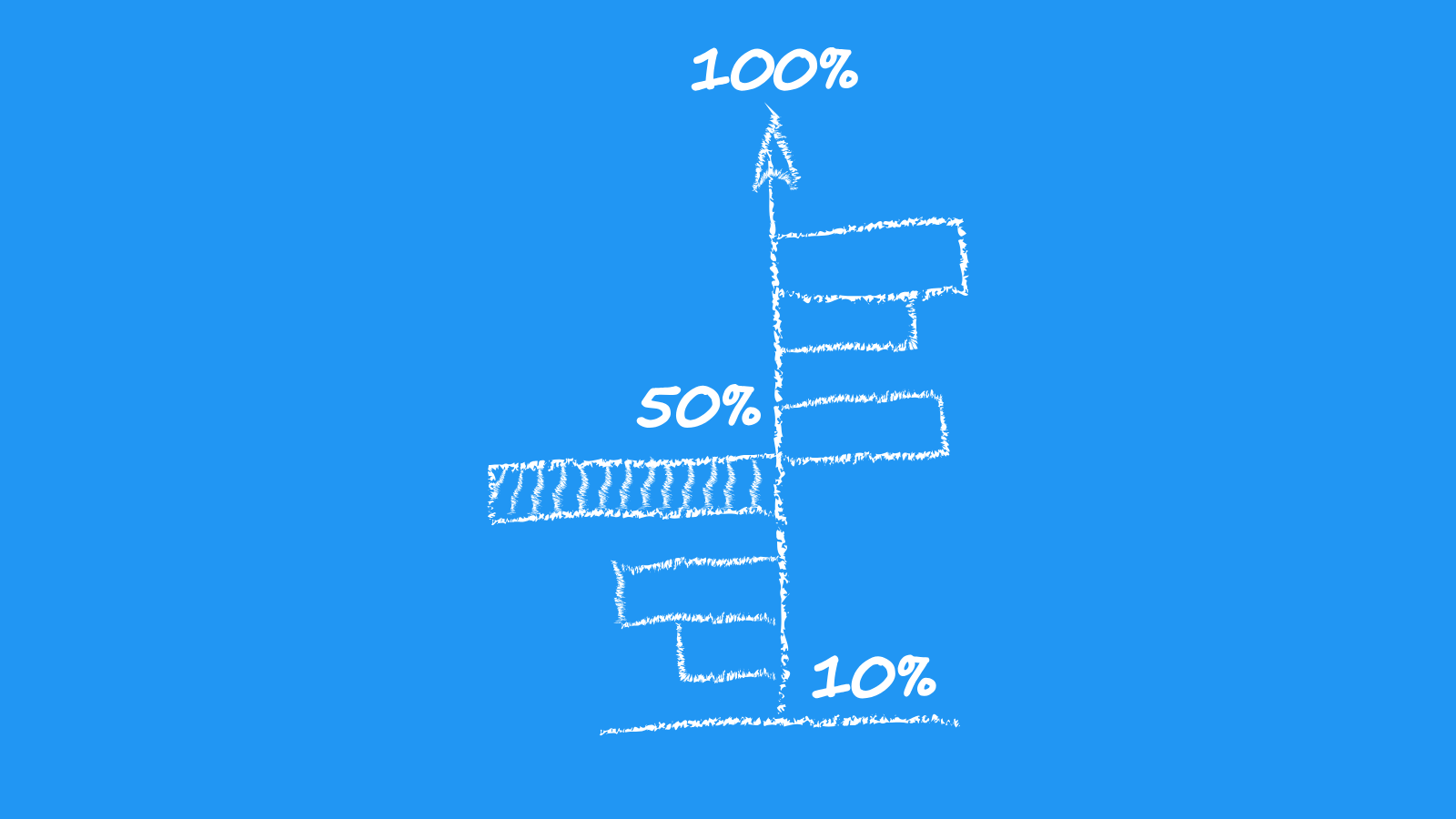
BMR Stock Forecast Machine Learning Model
Our team, comprised of data scientists and economists, proposes a comprehensive machine learning model for forecasting Beamr Imaging Ltd. (BMR) stock performance. The model's architecture leverages a multi-faceted approach, incorporating diverse data sources and analytical techniques. We will utilize historical stock data, including trading volume, open, high, low, and close prices, as the primary input. Complementing this, we will integrate fundamental data, such as Beamr Imaging's financial statements (revenue, earnings, debt levels, cash flow), market capitalization, and industry-specific metrics. Furthermore, sentiment analysis derived from news articles, social media, and financial analyst reports will be incorporated to gauge market sentiment towards BMR and the broader industry. The model will explore a suite of machine learning algorithms, including Recurrent Neural Networks (RNNs), specifically Long Short-Term Memory (LSTM) networks, known for their effectiveness in time-series forecasting, alongside Support Vector Machines (SVMs) and Random Forests. These algorithms will be trained on the comprehensive dataset to learn complex patterns and dependencies within the data.
The model's training and validation process will follow a rigorous methodology. The dataset will be split into training, validation, and testing sets. The training set will be used to train the machine learning algorithms, while the validation set will be utilized for hyperparameter tuning and model selection. Performance will be evaluated using appropriate metrics, such as Mean Absolute Error (MAE), Root Mean Squared Error (RMSE), and R-squared, to assess the model's accuracy and reliability. Cross-validation techniques will be employed to ensure robustness and prevent overfitting. A feature engineering process will transform raw data into features suitable for the model, incorporating technical indicators (Moving Averages, RSI, MACD) and feature scaling techniques. To mitigate risk and enhance predictive accuracy, the model will incorporate an ensemble approach, combining the predictions from different algorithms. Regular model updates and retraining, incorporating the newest data, are crucial for preserving the model's accuracy over time.
The final model will generate a probabilistic forecast for BMR stock performance, providing investors with a range of potential outcomes and associated confidence levels. The model's output will inform investment decisions by offering insights into the likelihood of price fluctuations over specific time horizons. This predictive capability, while not guaranteeing profits, will aid in risk management and strategic planning. Regular monitoring and analysis will be conducted to assess the model's performance and identify potential limitations. Continuous improvement will be a core feature of the project, incorporating feedback and adapting to new data and market dynamics. The successful implementation of this model will deliver important data-driven support for investment in BMR, and facilitate a better grasp of future potential and market risks.
```
ML Model Testing
n:Time series to forecast
p:Price signals of Beamr Imaging stock
j:Nash equilibria (Neural Network)
k:Dominated move of Beamr Imaging stock holders
a:Best response for Beamr Imaging target price
For further technical information as per how our model work we invite you to visit the article below:
How do KappaSignal algorithms actually work?
Beamr Imaging Stock Forecast (Buy or Sell) Strategic Interaction Table
Strategic Interaction Table Legend:
X axis: *Likelihood% (The higher the percentage value, the more likely the event will occur.)
Y axis: *Potential Impact% (The higher the percentage value, the more likely the price will deviate.)
Z axis (Grey to Black): *Technical Analysis%
Beamr Imaging Ltd. Ordinary Share: Financial Outlook and Forecast
The financial outlook for Beamr Imaging (BMR) appears promising, driven by its innovative video encoding and optimization technology. The company's core business revolves around providing software solutions that enhance video quality, reduce file sizes, and improve bandwidth efficiency. This is particularly relevant in an era of exploding video consumption across various platforms, including streaming services, mobile devices, and social media. BMR's ability to offer significant cost savings and performance improvements to content providers and distributors positions it favorably in the competitive landscape. The ongoing shift towards 4K and 8K video resolutions, coupled with the increasing demand for efficient video delivery, provides a substantial tailwind for the company's growth. Furthermore, its focus on technologies like High Dynamic Range (HDR) optimization further enhances its value proposition. Strategic partnerships and collaborations with industry leaders could further bolster its market presence and revenue streams.
BMR's financial forecast is expected to reflect this positive trajectory. Revenue growth is anticipated to be driven by increased adoption of its encoding and optimization solutions. As the demand for high-quality, efficient video delivery continues to rise, BMR is well-positioned to capture a larger share of the market. Operating margins are likely to improve over time as the company scales its operations and achieves greater economies of scale. Investing in research and development (R&D) to maintain a competitive edge and to drive innovation is key to long-term sustainability. The company's ability to secure and retain key customer relationships and successfully navigate the evolving technological landscape will be crucial for the overall financial performance.
Factors that could impact BMR's financial outlook includes the competitive landscape and the adoption rates of new video encoding standards. Competitors in the video processing space pose a constant challenge, necessitating continuous innovation and improvements to maintain market share. The success of the company hinges on its ability to adapt its technology to meet evolving industry standards and customer demands. Additionally, broader macroeconomic factors, such as economic downturns, could potentially reduce spending on video content, thus impacting the growth of the video encoding market. Furthermore, the cost of acquiring new customers and the ability to effectively market and sell its products will also influence its financial performance.
Overall, the forecast for BMR is positive, with expectations of solid revenue growth and improving profitability. The company's innovative technology, coupled with the growing demand for efficient video delivery, creates a favorable environment for expansion. However, this prediction is subject to risks. Competition in the industry and potential shifts in technology standards could impede growth. Therefore, the company needs to remain proactive in its technological advancements and customer acquisition efforts. Any failure to address these risks could hinder the realization of the predicted growth trajectory.
Rating | Short-Term | Long-Term Senior |
---|---|---|
Outlook | Ba3 | B1 |
Income Statement | Baa2 | B3 |
Balance Sheet | Baa2 | Baa2 |
Leverage Ratios | Baa2 | Baa2 |
Cash Flow | C | Caa2 |
Rates of Return and Profitability | Ba2 | Caa2 |
*Financial analysis is the process of evaluating a company's financial performance and position by neural network. It involves reviewing the company's financial statements, including the balance sheet, income statement, and cash flow statement, as well as other financial reports and documents.
How does neural network examine financial reports and understand financial state of the company?
References
- Morris CN. 1983. Parametric empirical Bayes inference: theory and applications. J. Am. Stat. Assoc. 78:47–55
- R. Howard and J. Matheson. Risk sensitive Markov decision processes. Management Science, 18(7):356– 369, 1972
- Bai J, Ng S. 2017. Principal components and regularized estimation of factor models. arXiv:1708.08137 [stat.ME]
- S. Bhatnagar, H. Prasad, and L. Prashanth. Stochastic recursive algorithms for optimization, volume 434. Springer, 2013
- J. Harb and D. Precup. Investigating recurrence and eligibility traces in deep Q-networks. In Deep Reinforcement Learning Workshop, NIPS 2016, Barcelona, Spain, 2016.
- Alpaydin E. 2009. Introduction to Machine Learning. Cambridge, MA: MIT Press
- Bell RM, Koren Y. 2007. Lessons from the Netflix prize challenge. ACM SIGKDD Explor. Newsl. 9:75–79