AUC Score :
Short-term Tactic1 :
Dominant Strategy :
Time series to forecast n:
ML Model Testing : Ensemble Learning (ML)
Hypothesis Testing : Factor
Surveillance : Major exchange and OTC
1Short-term revised.
2Time series is updated based on short-term trends.
Key Points
SEDU faces a mixed outlook. Continued growth in its non-US programs and potential expansion into new educational areas suggest upward momentum for revenue. However, increasing competition within the online education market could limit SEDU's ability to gain significant market share. The company is also exposed to regulatory scrutiny, particularly concerning student loan practices, potentially impacting enrollment and profitability. Overall, the company is projected to experience moderate financial performance, and risks include heightened competition, evolving regulations, and fluctuations in enrollment, which may constrain growth and affect profit margins.About Strategic Education
SEI is a global education company focused on providing educational programs for working adults. Through its subsidiaries, SEI delivers online post-secondary education, primarily in business, management, information technology, and healthcare. The company operates primarily in the United States and internationally. SEI's business model emphasizes career-oriented programs designed to meet the needs of adult learners seeking to enhance their skills and advance their careers. The company also offers educational services to employers seeking to upskill their workforce.
SEI's strategy centers on expanding its program offerings, growing its student base, and leveraging technology to improve educational outcomes. The company emphasizes its commitment to quality and student success. SEI also focuses on partnerships with employers and educational institutions to offer tailored programs. SEI aims to be a leader in online education and a provider of accessible and relevant educational opportunities for adult learners worldwide. The company's long-term objectives involve sustainable growth and shareholder value creation through its diversified educational offerings.
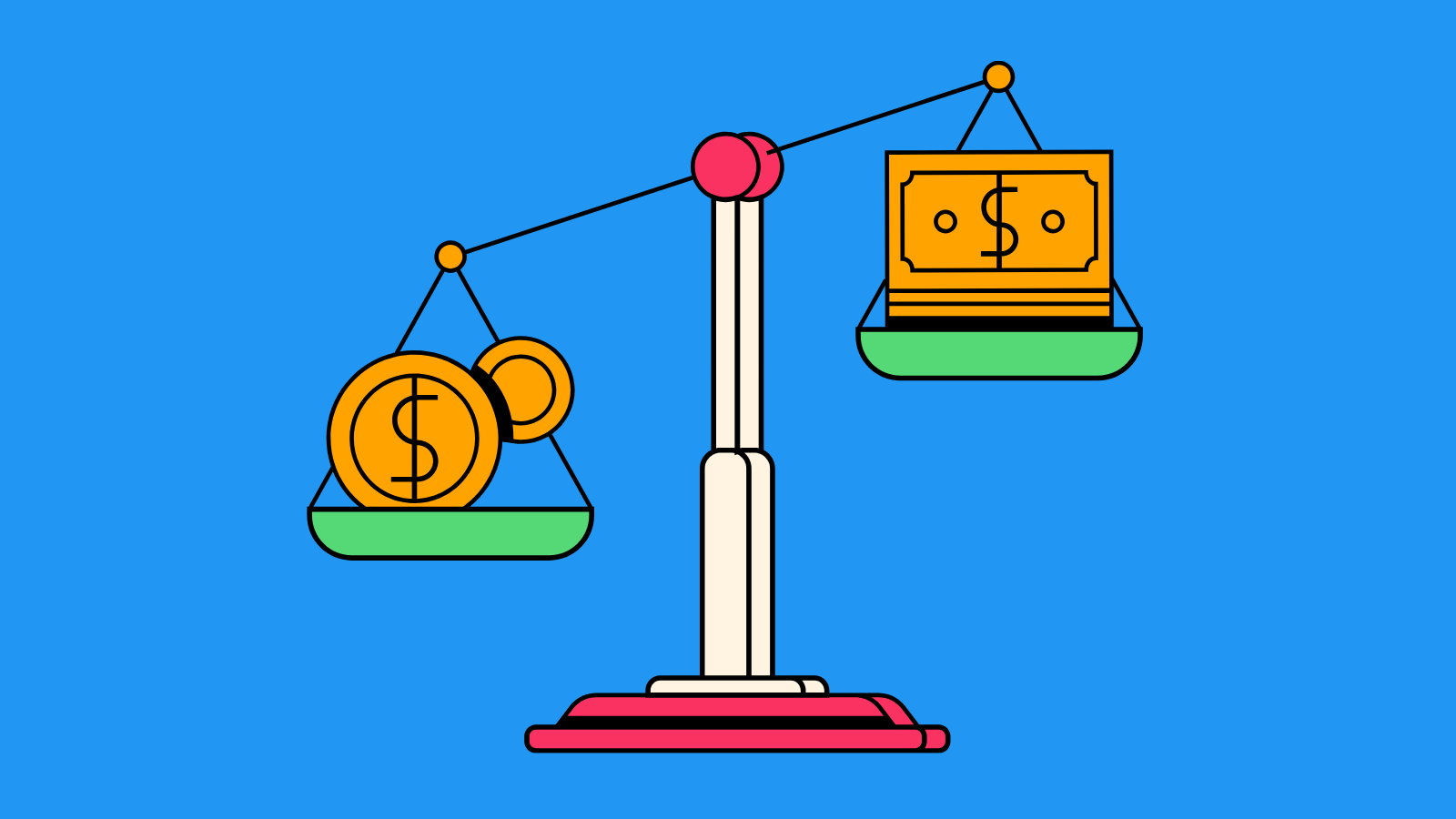
STRA Stock Forecast: A Machine Learning Model Approach
Our team proposes a robust machine learning model to forecast the performance of Strategic Education Inc. (STRA) stock. The model leverages a comprehensive set of both fundamental and technical indicators. Fundamental data will include financial statements (balance sheets, income statements, cash flow statements), key performance indicators (KPIs) such as student enrollment numbers, graduation rates, revenue per student, debt levels, and operating margins. We will incorporate macroeconomic factors, including interest rates, inflation, unemployment rates, and relevant government regulations affecting the education sector. Technical indicators will encompass historical price data, trading volume, moving averages (SMA, EMA), Relative Strength Index (RSI), Moving Average Convergence Divergence (MACD), and other relevant oscillators and chart patterns. Data preprocessing will involve cleaning, handling missing values, outlier detection, and feature scaling to ensure data quality and consistency.
The core of our forecasting system will employ a hybrid machine learning approach. We will utilize a combination of algorithms, including Recurrent Neural Networks (RNNs), specifically Long Short-Term Memory (LSTM) networks, known for their strength in time-series analysis, and Gradient Boosting Machines (GBM), such as XGBoost or LightGBM, which excel at capturing complex non-linear relationships. LSTM networks will process the time-series data from historical stock prices, financial metrics and economic indicators to identify patterns and dependencies. GBMs will incorporate a broader set of features, including those from fundamental analysis and technical indicators, to predict the direction and magnitude of price movements. The output of these models will be combined using a weighted averaging technique or a meta-learner, like a stacking model, to create a final prediction, mitigating the individual biases of each algorithm. This ensemble approach aims to provide a more accurate and reliable forecast.
The model will undergo rigorous backtesting and validation using historical data, covering various market conditions. We will evaluate the model's performance using appropriate metrics, including Mean Absolute Error (MAE), Root Mean Squared Error (RMSE), and direction accuracy, to measure the forecast's accuracy. The model will be regularly retrained with updated data and adjusted based on performance. A risk management framework will be integrated, incorporating sensitivity analysis to assess the impact of changes in key input variables on the stock's forecast. The model will also incorporate scenario planning to explore potential outcomes under different economic and regulatory scenarios. Finally, we will implement robust monitoring and alert systems to identify potential model degradation and ensure timely updates.
ML Model Testing
n:Time series to forecast
p:Price signals of Strategic Education stock
j:Nash equilibria (Neural Network)
k:Dominated move of Strategic Education stock holders
a:Best response for Strategic Education target price
For further technical information as per how our model work we invite you to visit the article below:
How do KappaSignal algorithms actually work?
Strategic Education Stock Forecast (Buy or Sell) Strategic Interaction Table
Strategic Interaction Table Legend:
X axis: *Likelihood% (The higher the percentage value, the more likely the event will occur.)
Y axis: *Potential Impact% (The higher the percentage value, the more likely the price will deviate.)
Z axis (Grey to Black): *Technical Analysis%
Strategic Education, Inc. (SEI) Financial Outlook and Forecast
The financial outlook for SEI presents a mixed bag, shaped by the evolving landscape of higher education and workforce development. SEI's primary segments, Strayer University and Capella University, cater to working adults seeking career advancement through online programs. Revenue growth has been observed due to a sustained demand for online learning. Key factors influencing the financial performance include student enrollment trends, tuition pricing strategies, and the effectiveness of student retention initiatives. Furthermore, SEI's strategic diversification into workforce solutions, such as the recent acquisition of the Fullstack Academy, are poised to add new revenue streams and growth opportunities, especially by tapping into in-demand skills training programs. This diversification reduces the company's reliance solely on traditional degree programs. Operational efficiency, management of costs, and successful integration of acquisitions remain critical to its success. These factors, coupled with broader economic conditions and the regulatory environment affecting the higher education sector, will collectively determine SEI's trajectory.
The forecast for SEI's financial performance in the next few years is cautiously optimistic. Enrollment in online programs is expected to remain steady, reflecting the ongoing preference for flexible learning options. SEI's focus on career-oriented programs and its ability to adapt to changing market demands gives the company a competitive advantage. The workforce solutions segment is expected to experience robust growth, driven by the increasing demand for skilled workers and the effectiveness of these programs. SEI's investments in technology and marketing will likely support student acquisition. These strategies would strengthen the company's position within the marketplace. However, maintaining positive financial performance requires consistent effort. The ability to manage expenses, improve student retention, and to navigate regulatory changes are crucial for financial stability and sustained growth.
In terms of long-term prospects, SEI's success will depend on its ability to innovate and adapt to emerging trends. SEI's investment in technology and data analytics could yield greater effectiveness of programs. The development of new programs aligned with evolving industry needs is also critical. Maintaining a strong focus on student outcomes, including job placement rates and career advancement, is of utmost importance to enhance the company's reputation and appeal to prospective students. Furthermore, SEI's ability to form partnerships with employers and industry leaders could significantly expand its reach and influence. The competitive environment of higher education would influence the company's market share. The successful integration of acquisitions and the ability to leverage synergies across its various business units will enhance operational efficiencies and drive sustainable growth.
Based on current trends and strategic initiatives, it is predicted that SEI will maintain a positive growth trajectory over the next few years, driven by the expansion of workforce solutions and steady growth in its online university segments. However, this prediction is subject to certain risks. Increased competition from other online education providers, potential changes in government regulations, and economic downturns that may impact student enrollment pose considerable challenges. Furthermore, SEI faces the risk of not adequately adapting to the changing demands of the labor market, which could negatively impact the growth of its workforce solutions segment. The company's ability to successfully integrate acquisitions and realize anticipated synergies also represents a key risk that must be addressed to mitigate its challenges. Successfully navigating these risks will be essential for SEI to deliver on its financial forecast.
Rating | Short-Term | Long-Term Senior |
---|---|---|
Outlook | B2 | B1 |
Income Statement | B3 | Caa2 |
Balance Sheet | Caa2 | Caa2 |
Leverage Ratios | B3 | B2 |
Cash Flow | Ba3 | Baa2 |
Rates of Return and Profitability | Baa2 | B3 |
*Financial analysis is the process of evaluating a company's financial performance and position by neural network. It involves reviewing the company's financial statements, including the balance sheet, income statement, and cash flow statement, as well as other financial reports and documents.
How does neural network examine financial reports and understand financial state of the company?
References
- Scott SL. 2010. A modern Bayesian look at the multi-armed bandit. Appl. Stoch. Models Bus. Ind. 26:639–58
- N. B ̈auerle and A. Mundt. Dynamic mean-risk optimization in a binomial model. Mathematical Methods of Operations Research, 70(2):219–239, 2009.
- J. Z. Leibo, V. Zambaldi, M. Lanctot, J. Marecki, and T. Graepel. Multi-agent Reinforcement Learning in Sequential Social Dilemmas. In Proceedings of the 16th International Conference on Autonomous Agents and Multiagent Systems (AAMAS 2017), Sao Paulo, Brazil, 2017
- Cheung, Y. M.D. Chinn (1997), "Further investigation of the uncertain unit root in GNP," Journal of Business and Economic Statistics, 15, 68–73.
- M. Benaim, J. Hofbauer, and S. Sorin. Stochastic approximations and differential inclusions, Part II: Appli- cations. Mathematics of Operations Research, 31(4):673–695, 2006
- S. Bhatnagar and K. Lakshmanan. An online actor-critic algorithm with function approximation for con- strained Markov decision processes. Journal of Optimization Theory and Applications, 153(3):688–708, 2012.
- V. Borkar. A sensitivity formula for the risk-sensitive cost and the actor-critic algorithm. Systems & Control Letters, 44:339–346, 2001