AUC Score :
Short-term Tactic1 :
Dominant Strategy :
Time series to forecast n:
ML Model Testing : Modular Neural Network (Market News Sentiment Analysis)
Hypothesis Testing : Beta
Surveillance : Major exchange and OTC
1Short-term revised.
2Time series is updated based on short-term trends.
Key Points
The S&P Bitcoin Index is predicted to exhibit significant volatility, potentially experiencing periods of substantial gains followed by sharp corrections. This volatility stems from factors such as regulatory uncertainty, evolving institutional adoption, and speculative trading activity. The index's value could increase substantially if wider adoption and mainstream acceptance of Bitcoin continues, and if more institutional investors enter the market. However, downside risks are considerable. Adverse regulatory actions or a loss of investor confidence could trigger a major price crash. Furthermore, technological advancements impacting Bitcoin's network or competition from other cryptocurrencies could negatively impact the index.About S&P Bitcoin Index
The S&P Bitcoin Index, a product of S&P Dow Jones Indices, provides a benchmark for the performance of the cryptocurrency Bitcoin. It aims to offer investors a transparent and reliable tool to track Bitcoin's market movements. The index is designed to be a tradable and investable benchmark, making it easier for market participants to understand and potentially gain exposure to Bitcoin's performance. It adheres to S&P DJI's rigorous methodology, which encompasses rules for index construction, maintenance, and governance, intended to ensure its integrity and representativeness.
The index is constructed using publicly available data, primarily from established Bitcoin exchanges. The methodology employed for the S&P Bitcoin Index is designed to be straightforward, ensuring the index's replicability and ease of understanding. The index undergoes periodic reviews to account for market developments and maintain its accuracy. The S&P Bitcoin Index aims to offer institutional and retail investors a standardized metric for evaluating Bitcoin's performance, facilitating investment decisions and providing a basis for product development within the digital asset space.
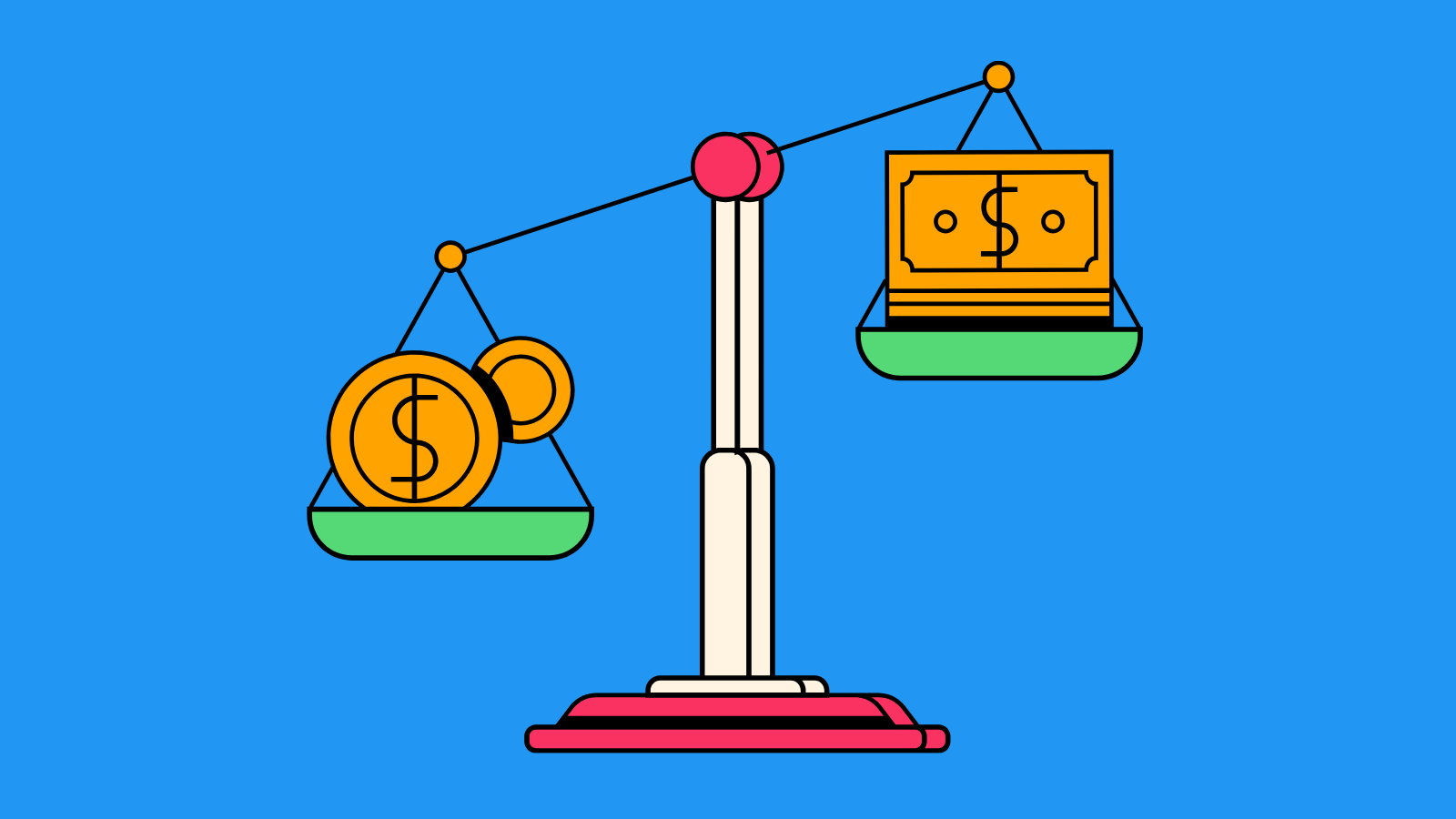
S&P Bitcoin Index Forecasting Model
Our team, comprised of data scientists and economists, has developed a machine learning model for forecasting the S&P Bitcoin Index. The model leverages a comprehensive set of predictor variables categorized into several key areas. These include historical price data, encompassing various time-series features such as moving averages, volatility measures (e.g., Bollinger Bands, Average True Range), and lagged price values. We also incorporate market sentiment analysis, utilizing natural language processing techniques to gauge investor sentiment from news articles, social media posts, and financial reports related to Bitcoin. Furthermore, the model incorporates on-chain metrics, such as transaction volumes, active addresses, mining difficulty, and the number of large transactions, as these provide insights into the underlying network activity and investor behavior. Finally, external factors such as macroeconomic indicators (e.g., inflation rates, interest rates, and economic growth) and regulatory developments are considered as potential influencing factors.
The model architecture employs a hybrid approach, combining the strengths of several machine learning algorithms. We utilize Recurrent Neural Networks (RNNs), particularly Long Short-Term Memory (LSTM) networks, for time-series forecasting given their capability to capture temporal dependencies inherent in financial data. A Gradient Boosting Machine (GBM) is used to assess and incorporate the importance of features in the predictive task. The GBM models the feature importance and assists in feature selection by identifying predictors with the highest impact on the index's movement. The output of both the LSTM and the GBM models is then integrated through an ensemble method, aiming to reduce variance and improve overall forecast accuracy. Cross-validation is employed to assess the model's performance across different periods. The dataset is divided into training, validation, and test sets to ensure the model's generalization capabilities and prevent overfitting.
The model is evaluated using various performance metrics, including Mean Absolute Error (MAE), Root Mean Squared Error (RMSE), and the Sharpe ratio. These metrics quantify the accuracy and risk-adjusted performance of the forecasts. The model is also equipped with a monitoring system that regularly updates the model's training data with new information. This allows it to adapt to shifting market conditions and maintain its forecasting accuracy over time. We have implemented a mechanism for backtesting with past data to simulate and evaluate the model's performance in past scenarios. Regular evaluations are performed to assess the model's effectiveness and to adapt it to future market developments. The model is designed to produce forecasts for a range of time horizons, catering to different investment strategies.
ML Model Testing
n:Time series to forecast
p:Price signals of S&P Bitcoin index
j:Nash equilibria (Neural Network)
k:Dominated move of S&P Bitcoin index holders
a:Best response for S&P Bitcoin target price
For further technical information as per how our model work we invite you to visit the article below:
How do KappaSignal algorithms actually work?
S&P Bitcoin Index Forecast Strategic Interaction Table
Strategic Interaction Table Legend:
X axis: *Likelihood% (The higher the percentage value, the more likely the event will occur.)
Y axis: *Potential Impact% (The higher the percentage value, the more likely the price will deviate.)
Z axis (Grey to Black): *Technical Analysis%
S&P Bitcoin Index: Financial Outlook and Forecast
The S&P Bitcoin Index, designed to track the performance of Bitcoin, offers a unique perspective on the digital asset market. Its financial outlook is inextricably linked to the broader cryptocurrency ecosystem, as well as global macroeconomic trends. The index's performance is predominantly driven by investor sentiment, regulatory developments, technological advancements, and the overall supply and demand dynamics of Bitcoin. Currently, the financial outlook for the S&P Bitcoin Index appears to be positioned within a phase of cautious optimism. This cautiousness stems from the nascent nature of the market and its inherent volatility, yet optimism derives from increasing institutional adoption, rising awareness among retail investors, and ongoing infrastructure development. The index's value is correlated with Bitcoin price, meaning events that influence Bitcoin directly will cascade into the index's outlook. Positive events like significant institutional investment, regulatory clarity, and wider adoption of Bitcoin for payments will support the growth of the index.
The forecast for the S&P Bitcoin Index hinges on several critical factors. Firstly, the regulatory landscape is paramount. Clear and supportive regulations could unlock substantial institutional capital and boost investor confidence, which would, in turn, positively impact the index. Conversely, restrictive regulations or outright bans in major economies could significantly depress its value. Secondly, technological developments and scalability solutions are crucial. Improvements in transaction speed, cost-effectiveness, and security of Bitcoin are essential for facilitating broader adoption and supporting index growth. Thirdly, the evolution of the market's infrastructure is critical. The growth of platforms that provide custody, trading, and derivatives products will make Bitcoin more accessible, liquid, and attractive to investors. Finally, macroeconomic factors such as inflation, interest rates, and the overall state of the global economy play a role. In times of economic uncertainty, some investors may view Bitcoin as a hedge against inflation or other financial risks, potentially bolstering its value and the index.
Key indicators to watch in evaluating the index's performance include: the trading volume of Bitcoin across various exchanges; the growth in the number of Bitcoin wallets, particularly those held by institutional investors; the increasing number of merchants accepting Bitcoin as a form of payment; and the development of new and innovative Bitcoin-related financial products and services, such as Exchange Traded Funds (ETFs). Furthermore, the index's performance will reflect changes in the market capitalization of Bitcoin and the flow of capital into the broader cryptocurrency market. Monitoring sentiment through market research, news coverage, and social media will be essential. Keep track of the correlation with other assets like gold, stocks and bonds. It's essential to follow the supply and demand, as it is a primary driver of price changes. A decreasing supply with high demand will support the index.
The prediction for the S&P Bitcoin Index is cautiously positive over the medium to long term. The ongoing institutionalization of Bitcoin, coupled with improvements in technology and infrastructure, should lead to sustained growth. However, this outlook is subject to considerable risk. The volatility of Bitcoin remains a significant challenge. Price swings are common and may result in significant index value fluctuations. Moreover, the regulatory environment surrounding Bitcoin is constantly evolving, creating uncertainty. Cybersecurity risks and hacks are also a constant threat, as any significant breach could erode investor confidence. Finally, the index's performance is dependent on overall market sentiment; any significant shifts in investor sentiment, particularly negative ones, could severely affect the index's performance. Therefore, while the long-term prospects seem promising, investors must be prepared for significant volatility and remain vigilant in monitoring the risks.
```
Rating | Short-Term | Long-Term Senior |
---|---|---|
Outlook | Ba3 | Ba2 |
Income Statement | C | Baa2 |
Balance Sheet | Ba3 | Baa2 |
Leverage Ratios | Baa2 | Ba3 |
Cash Flow | Baa2 | B3 |
Rates of Return and Profitability | B3 | Caa2 |
*An aggregate rating for an index summarizes the overall sentiment towards the companies it includes. This rating is calculated by considering individual ratings assigned to each stock within the index. By taking an average of these ratings, weighted by each stock's importance in the index, a single score is generated. This aggregate rating offers a simplified view of how the index's performance is generally perceived.
How does neural network examine financial reports and understand financial state of the company?
References
- C. Claus and C. Boutilier. The dynamics of reinforcement learning in cooperative multiagent systems. In Proceedings of the Fifteenth National Conference on Artificial Intelligence and Tenth Innovative Applications of Artificial Intelligence Conference, AAAI 98, IAAI 98, July 26-30, 1998, Madison, Wisconsin, USA., pages 746–752, 1998.
- J. Harb and D. Precup. Investigating recurrence and eligibility traces in deep Q-networks. In Deep Reinforcement Learning Workshop, NIPS 2016, Barcelona, Spain, 2016.
- Angrist JD, Pischke JS. 2008. Mostly Harmless Econometrics: An Empiricist's Companion. Princeton, NJ: Princeton Univ. Press
- Dietterich TG. 2000. Ensemble methods in machine learning. In Multiple Classifier Systems: First International Workshop, Cagliari, Italy, June 21–23, pp. 1–15. Berlin: Springer
- J. Filar, L. Kallenberg, and H. Lee. Variance-penalized Markov decision processes. Mathematics of Opera- tions Research, 14(1):147–161, 1989
- Bertsimas D, King A, Mazumder R. 2016. Best subset selection via a modern optimization lens. Ann. Stat. 44:813–52
- Bengio Y, Schwenk H, SenĂ©cal JS, Morin F, Gauvain JL. 2006. Neural probabilistic language models. In Innovations in Machine Learning: Theory and Applications, ed. DE Holmes, pp. 137–86. Berlin: Springer