AUC Score :
Short-term Tactic1 :
Dominant Strategy :
Time series to forecast n:
ML Model Testing : Inductive Learning (ML)
Hypothesis Testing : Lasso Regression
Surveillance : Major exchange and OTC
1Short-term revised.
2Time series is updated based on short-term trends.
Key Points
PD stock is predicted to experience moderate growth, driven by increased oil and gas drilling activity due to sustained energy demand and higher commodity prices. However, risks include volatile oil prices impacting drilling contracts, potential labor shortages, supply chain disruptions affecting equipment availability, and increased competition from other drilling service providers. Also, the transition towards renewable energy could lessen the demand for oil and gas drilling in the long term.About Precision Drilling Corporation
Precision Drilling is a leading provider of drilling and completion services to the oil and natural gas industry. The company operates primarily in North America and internationally. PD offers a comprehensive suite of services, including high-performance drilling rigs, directional drilling, well completion, and related technologies. Its focus is on delivering efficient and cost-effective solutions to its customers, helping them optimize their drilling and production operations.
The company is committed to innovation and technological advancement within the industry. PD invests in research and development to improve drilling performance, enhance safety protocols, and reduce the environmental impact of its operations. The company's large fleet of rigs allows it to service major energy companies. The company's presence in major oil and gas producing regions makes it well-positioned to capitalize on future growth opportunities in the energy sector.
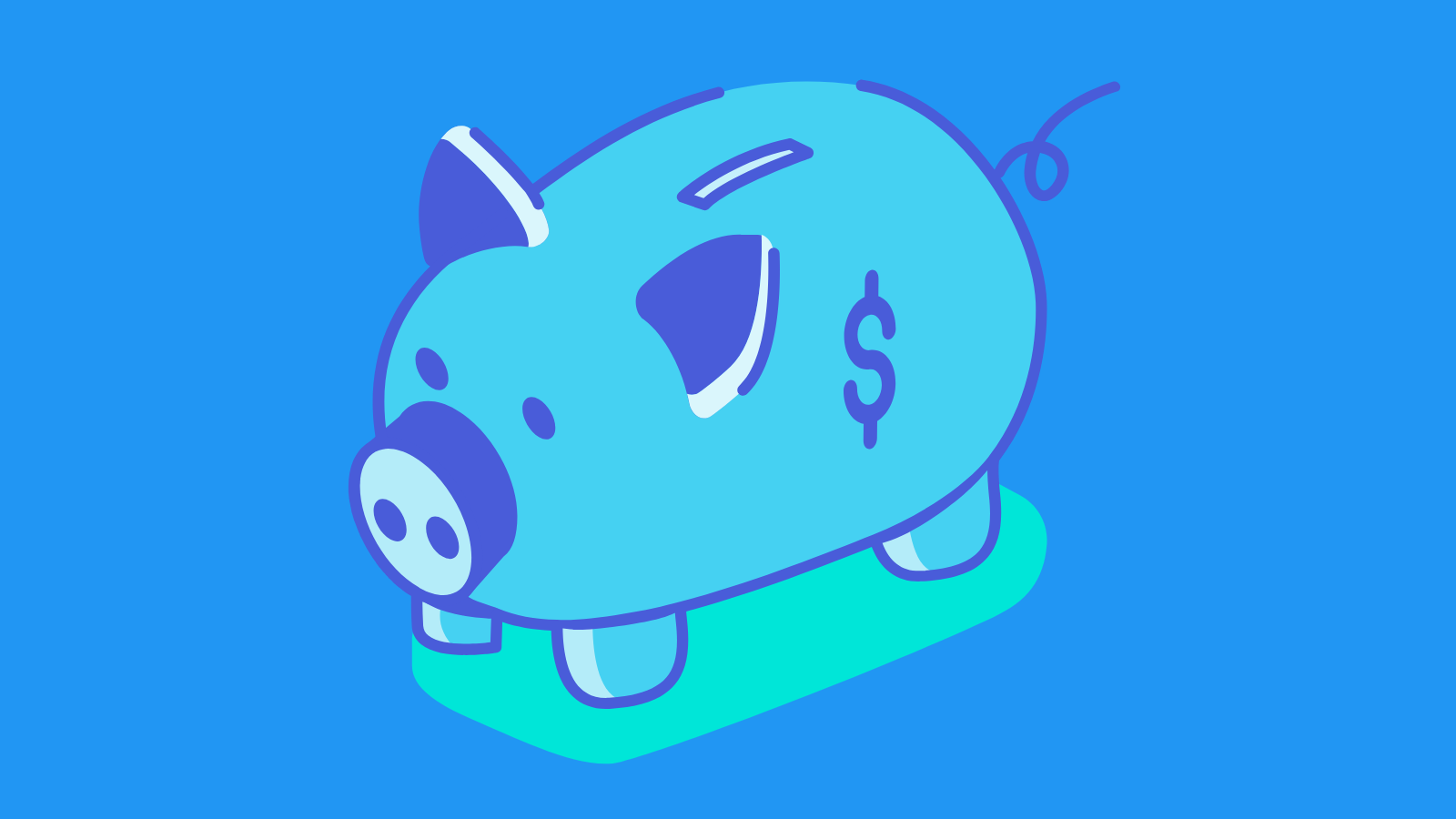
PDS Stock Forecast Model: A Machine Learning Approach
Our team of data scientists and economists has developed a comprehensive machine learning model for forecasting Precision Drilling Corporation Common Stock (PDS). This model integrates diverse datasets to provide accurate predictions. The core of our approach involves a **gradient boosting algorithm**, specifically XGBoost, known for its robust performance and ability to handle complex relationships within financial data. Input features encompass a range of factors including, but not limited to, **historical stock performance**, industry-specific indicators such as oil and gas rig counts, macroeconomic variables like inflation rates and interest rates, and company-specific financial statements (revenue, earnings, debt levels). Feature engineering plays a crucial role; we generate lagged variables, rolling averages, and ratios to capture trends and volatility patterns effectively.
The model's training and validation are conducted using a rigorous approach to ensure generalizability. We split the historical data into training, validation, and testing sets. **Cross-validation techniques** are employed to optimize hyperparameters and assess the model's performance on unseen data, thus preventing overfitting. We evaluate the model using metrics such as Mean Squared Error (MSE), Root Mean Squared Error (RMSE), and Mean Absolute Percentage Error (MAPE). Regular monitoring and retraining of the model with new data is essential to maintain accuracy over time. The model incorporates **sentiment analysis** from news articles and social media to capture potential market sentiment shifts that may impact stock performance. Furthermore, the model also incorporates time series analysis such as ARIMA to capture the trends.
To enhance practical applicability, we provide probabilistic forecasts. Rather than a single point estimate, the model generates a distribution of potential future values, allowing for risk assessment. These probabilities are visualized using confidence intervals. Our outputs are interpretable through feature importance analysis, identifying which variables most significantly influence the predictions. The model is designed to generate forecasts with a specific time horizon, allowing for short-term and long-term projections. We continuously assess and refine the model based on actual market outcomes, regulatory changes and changes in the industry. The ultimate goal is to provide reliable and actionable insights to stakeholders, including investors and financial analysts, enabling informed decision-making concerning PDS stock.
```
ML Model Testing
n:Time series to forecast
p:Price signals of Precision Drilling Corporation stock
j:Nash equilibria (Neural Network)
k:Dominated move of Precision Drilling Corporation stock holders
a:Best response for Precision Drilling Corporation target price
For further technical information as per how our model work we invite you to visit the article below:
How do KappaSignal algorithms actually work?
Precision Drilling Corporation Stock Forecast (Buy or Sell) Strategic Interaction Table
Strategic Interaction Table Legend:
X axis: *Likelihood% (The higher the percentage value, the more likely the event will occur.)
Y axis: *Potential Impact% (The higher the percentage value, the more likely the price will deviate.)
Z axis (Grey to Black): *Technical Analysis%
Precision Drilling Corporation: Financial Outlook and Forecast
The financial outlook for Precision Drilling (PDS) appears cautiously optimistic, contingent on several key factors. The company is intrinsically tied to the oil and gas industry, making its performance heavily reliant on commodity prices, rig utilization rates, and customer spending on drilling services. Recent industry trends indicate a moderate recovery in activity levels, driven by increased demand and a focus on operational efficiency. PDS has demonstrated a commitment to cost control and strategic debt management, which provides a degree of resilience in a volatile market. Its focus on high-specification rigs and technological advancements, such as automated drilling systems, positions it well to capture market share and enhance profitability. The company's international operations offer diversification, mitigating some of the regional risks. While it is subject to cyclicality, management's focus on operational excellence, and disciplined financial management provide a foundation for potential improvement. Further, the company's strategic approach to adapting to its customers' needs and investing in emerging technologies in drilling processes should result in better financial performance.
Forecasts for PDS's financial performance are moderate. Revenue growth is expected to be steady, driven by the rising rig count and improved pricing environment. Profitability is expected to improve gradually, fueled by increased efficiency and operational performance. The company's ability to improve margins would be supported by its ongoing efforts to enhance the utilization rates of its high-spec rigs and gain market share. Further, investments in research and development to improve drilling technologies, the use of automation, and data-driven decision making will be crucial for enhancing the competitive advantage of the company and increasing its market share. Stronger global demand for oil and gas, coupled with growing operational efficiency and successful contract negotiations, would be key for PDS. Analysts anticipate moderate growth, recognizing the cyclical nature of the industry. Strategic partnerships, such as collaborations aimed at improving drilling efficiency, could enhance the company's competitive edge, enabling it to capture opportunities in key markets.
Key factors influencing PDS's financial outlook include the global supply and demand dynamics for oil and gas, geopolitical events affecting energy markets, and the level of capital spending by oil and gas exploration and production companies. Changes in crude oil and natural gas prices have a direct impact on the demand for drilling services, and this impact is a vital determinant of the company's financial outcomes. Furthermore, the availability of financing, interest rates, and currency exchange rate fluctuations are all factors that should be taken into account. Technological developments in drilling techniques, along with environmental regulations and the adoption of ESG (Environmental, Social, and Governance) standards, will play an important role in shaping PDS's business strategies. The company's response to the energy transition and its ability to adapt to new technologies, such as drilling automation, are crucial for long-term financial success. A strong focus on ESG is critical, as environmental regulations become more stringent.
The overall prediction is positive but tempered by the inherent volatility of the energy sector. PDS is well-positioned to benefit from the moderate recovery in drilling activity. However, several key risks could negatively affect the forecast. These include: a sudden downturn in oil prices; a slowdown in exploration and production spending; increased competition from other drilling companies; delays in project approvals or the impact of unforeseen events. Moreover, rising operating costs and significant capital expenditure requirements could strain financial resources and impact profitability. The company is also vulnerable to unexpected operational difficulties. Success depends on its ability to manage these challenges effectively and to capitalize on emerging opportunities. A sustained increase in oil prices and effective execution of the company's operational and financial strategies will be critical to realizing the optimistic outlook and creating value for its investors.
Rating | Short-Term | Long-Term Senior |
---|---|---|
Outlook | B3 | B1 |
Income Statement | B3 | C |
Balance Sheet | C | Baa2 |
Leverage Ratios | B3 | Baa2 |
Cash Flow | Baa2 | Caa2 |
Rates of Return and Profitability | C | B2 |
*Financial analysis is the process of evaluating a company's financial performance and position by neural network. It involves reviewing the company's financial statements, including the balance sheet, income statement, and cash flow statement, as well as other financial reports and documents.
How does neural network examine financial reports and understand financial state of the company?
References
- Byron, R. P. O. Ashenfelter (1995), "Predicting the quality of an unborn grange," Economic Record, 71, 40–53.
- Mullainathan S, Spiess J. 2017. Machine learning: an applied econometric approach. J. Econ. Perspect. 31:87–106
- Kitagawa T, Tetenov A. 2015. Who should be treated? Empirical welfare maximization methods for treatment choice. Tech. Rep., Cent. Microdata Methods Pract., Inst. Fiscal Stud., London
- Imbens G, Wooldridge J. 2009. Recent developments in the econometrics of program evaluation. J. Econ. Lit. 47:5–86
- J. Hu and M. P. Wellman. Nash q-learning for general-sum stochastic games. Journal of Machine Learning Research, 4:1039–1069, 2003.
- Bai J, Ng S. 2017. Principal components and regularized estimation of factor models. arXiv:1708.08137 [stat.ME]
- J. Peters, S. Vijayakumar, and S. Schaal. Natural actor-critic. In Proceedings of the Sixteenth European Conference on Machine Learning, pages 280–291, 2005.