AUC Score :
Short-term Tactic1 :
Dominant Strategy :
Time series to forecast n:
ML Model Testing : Modular Neural Network (Market News Sentiment Analysis)
Hypothesis Testing : Wilcoxon Sign-Rank Test
Surveillance : Major exchange and OTC
1Short-term revised.
2Time series is updated based on short-term trends.
Key Points
Pembina's stock is projected to experience moderate growth, driven by ongoing demand for its pipeline and midstream services and expansion projects that should contribute to increased revenue. The company's steady dividend payments will likely continue to attract income-focused investors. However, Pembina faces risks including fluctuations in commodity prices, which can impact pipeline volumes and profitability. Regulatory hurdles and environmental concerns related to pipeline projects could lead to delays or increased costs, negatively affecting the company's financial performance. Competition from other pipeline operators also poses a challenge to maintaining market share and project development.About Pembina Pipeline Corp.
Pembina Pipeline Corporation, a prominent energy transportation and infrastructure company, operates primarily in North America. The company focuses on the transportation and storage of hydrocarbons, including crude oil, natural gas, and natural gas liquids (NGLs). Pembina's extensive network of pipelines and facilities connects various supply sources with key demand markets. The company also provides midstream services such as fractionation, storage, and terminalling. A key aspect of Pembina's operations is its commitment to safety, environmental responsibility, and community engagement.
Pembina's business strategy centers around providing essential infrastructure services to the energy industry. They actively pursue organic growth opportunities through expansion of their existing assets and strategic acquisitions. Pembina works on strengthening relationships with customers, prioritizing long-term contracts to generate stable cash flows. They are also increasingly focusing on energy transition initiatives and exploring opportunities in renewable energy to evolve its business model and adapt to changing energy demands. The corporation places an emphasis on financial discipline and delivering consistent returns to shareholders.
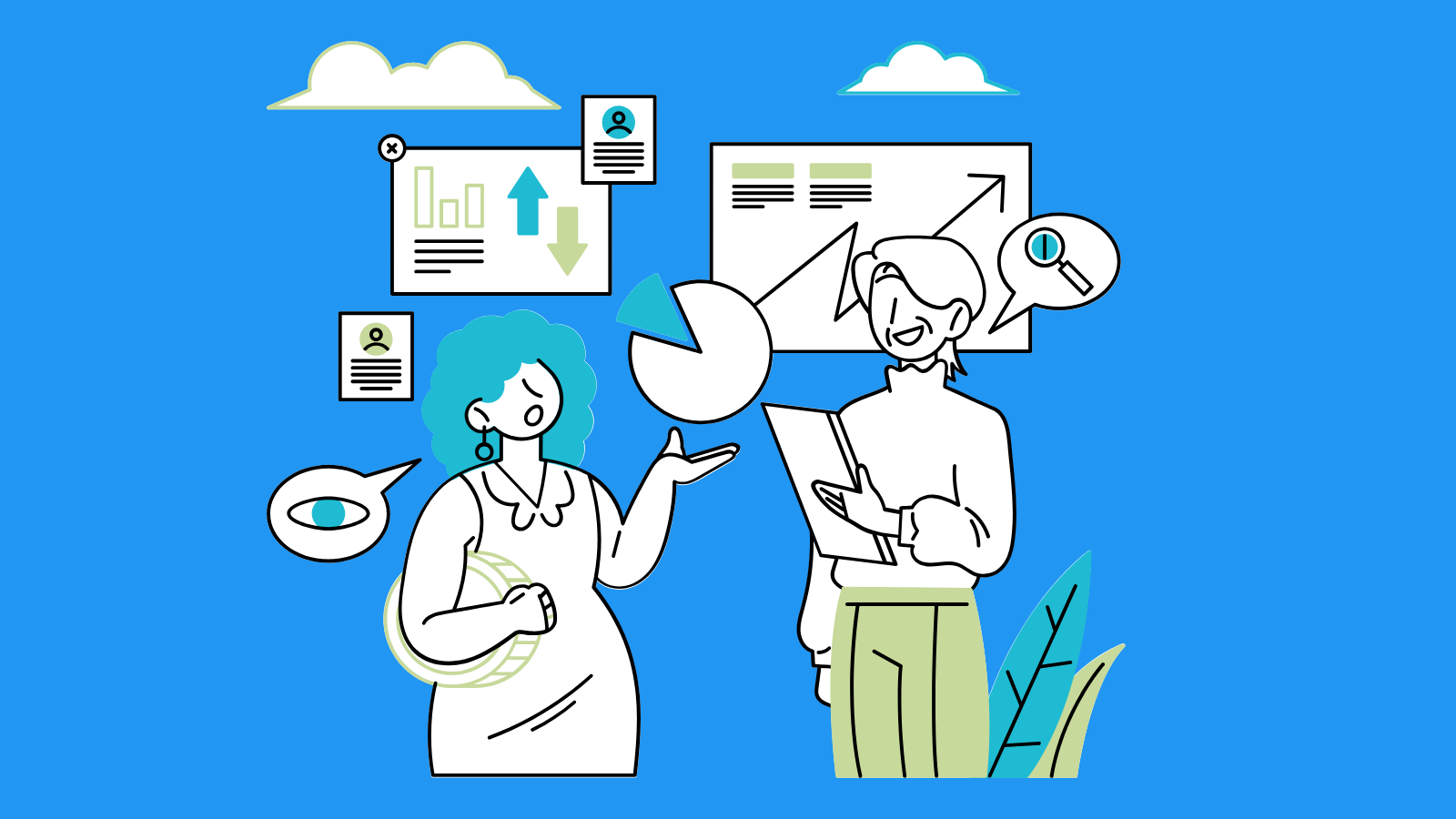
PBA Stock Price Forecasting Model
Our team proposes a comprehensive machine learning model for forecasting Pembina Pipeline Corp. (PBA) stock performance. This model integrates several key data sources to provide a robust and reliable prediction. We will utilize historical stock data, including daily opening, closing, highest, and lowest prices, as well as trading volume. Furthermore, we will incorporate fundamental financial data such as quarterly and annual earnings reports, revenue, debt levels, and dividend yields. Macroeconomic indicators, including Canadian GDP growth, inflation rates, interest rates, and oil and gas price indices (WTI and Canadian heavy oil) will also be included. We intend to account for any significant news events affecting the energy infrastructure sector, like regulatory changes or major pipeline project announcements.
The model will leverage a combination of machine learning algorithms. Time series analysis techniques, such as ARIMA and its variants, will be employed to capture the temporal dependencies inherent in stock price movements. Additionally, we will use ensemble methods, such as Random Forests and Gradient Boosting, as these excel in handling high-dimensional data and non-linear relationships. Data pre-processing will be crucial; including data cleaning, missing value imputation, and feature scaling to improve model accuracy. Feature engineering will involve creating technical indicators such as Moving Averages, Relative Strength Index (RSI), and Moving Average Convergence Divergence (MACD), as well as incorporating lagged variables. The model's performance will be evaluated using metrics like Mean Absolute Error (MAE), Root Mean Squared Error (RMSE), and R-squared, with cross-validation to ensure generalization capability.
The final model will be designed to provide forecasts for PBA's stock movement over various time horizons, for example, short-term predictions (daily or weekly) to medium-term (monthly or quarterly). The model will include confidence intervals to demonstrate the expected accuracy. Furthermore, we will build a dynamic update mechanism to train the model periodically with the latest data, ensuring its continued predictive power. The model's output will be presented in an easy-to-understand format, including visual representations of the forecasts. This approach allows for informed investment decisions for Pembina Pipeline Corp. Our model's limitations will be clearly outlined, recognizing that stock markets are inherently unpredictable and affected by unforeseen events.
ML Model Testing
n:Time series to forecast
p:Price signals of Pembina Pipeline Corp. stock
j:Nash equilibria (Neural Network)
k:Dominated move of Pembina Pipeline Corp. stock holders
a:Best response for Pembina Pipeline Corp. target price
For further technical information as per how our model work we invite you to visit the article below:
How do KappaSignal algorithms actually work?
Pembina Pipeline Corp. Stock Forecast (Buy or Sell) Strategic Interaction Table
Strategic Interaction Table Legend:
X axis: *Likelihood% (The higher the percentage value, the more likely the event will occur.)
Y axis: *Potential Impact% (The higher the percentage value, the more likely the price will deviate.)
Z axis (Grey to Black): *Technical Analysis%
Pembina's Financial Outlook and Forecast
Pembina Pipeline Corporation's financial outlook reflects a company positioned for moderate but consistent growth, largely driven by its diversified portfolio of energy infrastructure assets. The corporation's core business segments – pipelines, facilities, and marketing – generate a stable cash flow stream. Strong demand for energy resources and the gradual recovery in economic activities support Pembina's overall performance. Strategic acquisitions and project expansions, such as the addition of the Trans Mountain Pipeline system, have further solidified its market position and expanded its revenue base. Pembina's focus on providing essential services, even during periods of economic uncertainty, contributes to the financial stability. This also ensures that it will continue to attract investors seeking predictable income and a hedge against market volatility. Furthermore, Pembina's commitment to environmental, social, and governance (ESG) factors is important to ensure the company's long-term viability.
The corporation is expected to benefit from the rising demand for natural gas, crude oil, and natural gas liquids (NGLs) as the global economy continues to grow. The company's existing infrastructure network, including its pipelines and processing facilities, is strategically located to capitalize on these trends. Pembina's expansion plans, which involve capacity enhancements and new project developments, will contribute to increased volumes and revenues. The company's ability to efficiently transport and process energy products, while maintaining a competitive cost structure, further strengthens its financial forecast. The company also continues to focus on cost optimization and operational efficiencies to maximize profitability. This includes a continued focus on maintaining financial discipline to manage its debt levels effectively and to ensure long-term sustainability. The firm is expected to provide stable and growing dividends to its shareholders.
Key elements of the financial forecast include: moderate revenue growth driven by expanded pipeline capacity and increased throughput volumes; stable EBITDA (Earnings Before Interest, Taxes, Depreciation, and Amortization) margins due to the nature of its long-term, take-or-pay contracts; continued investment in strategic projects to expand its infrastructure network, and a commitment to returning cash to shareholders through dividends and share repurchases. The company's management is expected to maintain a balanced approach to capital allocation. This means investing in growth opportunities, reducing debt, and rewarding shareholders. The company continues to prioritize safe and reliable operations to ensure the integrity of its assets and minimize operational disruptions, such as unplanned pipeline outages.
The financial outlook for Pembina is positive. Continued demand growth for energy transportation and processing is expected to benefit the company. However, several risks could potentially impact this outlook. Economic downturns could reduce energy demand and volumes, and regulatory and political changes could affect pipeline project approvals and operations. Changes in environmental regulations related to emissions could increase operational costs. Fluctuations in commodity prices could impact margins in the marketing business segment. Increased competition from other energy infrastructure companies and the ongoing transition to renewable energy sources could represent long-term challenges. The company is likely to be moderately affected by these risks. Therefore, Pembina's success depends on its ability to adapt to evolving market conditions, efficiently manage its portfolio, and maintain a strong balance sheet to manage unexpected events.
Rating | Short-Term | Long-Term Senior |
---|---|---|
Outlook | B2 | B2 |
Income Statement | Ba3 | Baa2 |
Balance Sheet | B2 | B2 |
Leverage Ratios | C | Caa2 |
Cash Flow | Baa2 | Caa2 |
Rates of Return and Profitability | C | B2 |
*Financial analysis is the process of evaluating a company's financial performance and position by neural network. It involves reviewing the company's financial statements, including the balance sheet, income statement, and cash flow statement, as well as other financial reports and documents.
How does neural network examine financial reports and understand financial state of the company?
References
- R. Williams. Simple statistical gradient-following algorithms for connectionist reinforcement learning. Ma- chine learning, 8(3-4):229–256, 1992
- Athey S, Bayati M, Doudchenko N, Imbens G, Khosravi K. 2017a. Matrix completion methods for causal panel data models. arXiv:1710.10251 [math.ST]
- Kitagawa T, Tetenov A. 2015. Who should be treated? Empirical welfare maximization methods for treatment choice. Tech. Rep., Cent. Microdata Methods Pract., Inst. Fiscal Stud., London
- Scholkopf B, Smola AJ. 2001. Learning with Kernels: Support Vector Machines, Regularization, Optimization, and Beyond. Cambridge, MA: MIT Press
- Breiman L, Friedman J, Stone CJ, Olshen RA. 1984. Classification and Regression Trees. Boca Raton, FL: CRC Press
- Knox SW. 2018. Machine Learning: A Concise Introduction. Hoboken, NJ: Wiley
- Efron B, Hastie T, Johnstone I, Tibshirani R. 2004. Least angle regression. Ann. Stat. 32:407–99