AUC Score :
Short-term Tactic1 :
Dominant Strategy :
Time series to forecast n:
ML Model Testing : Modular Neural Network (Speculative Sentiment Analysis)
Hypothesis Testing : Wilcoxon Sign-Rank Test
Surveillance : Major exchange and OTC
1Short-term revised.
2Time series is updated based on short-term trends.
Key Points
PLTN's future hinges on the clinical success and commercial viability of its investigational therapies. Predictions suggest potential upside driven by positive trial results for its lead product, leading to potential regulatory approval and market penetration. This could trigger significant revenue growth. However, PLTN faces substantial risks. Negative clinical trial outcomes could severely impact its stock price and overall business prospects. Additionally, intense competition within the pharmaceutical sector and challenges in securing sufficient funding pose constant hurdles. Dilution of shareholder value through further offerings is also a possibility, which could negatively affect stock price. The company's success is heavily reliant on its ability to efficiently manage research and development costs and effectively navigate regulatory pathways.About Palatin Technologies Inc.
Palatin Technologies (PTN) is a biopharmaceutical company focused on developing targeted, first-in-class therapeutics. The company primarily operates in the biotechnology sector, aiming to address unmet medical needs through innovative drug development. PTN's research and development efforts concentrate on developing drugs that act through melanocortin receptor systems. These receptor systems play a critical role in various physiological processes, including sexual function, inflammation, and metabolic control. The company's business model is centered on research, preclinical development, clinical trials, and, ultimately, regulatory approval and commercialization of its pharmaceutical products.
PTN's pipeline includes several product candidates targeting diverse therapeutic areas. The company often collaborates with other pharmaceutical entities to further the development and commercialization of its products. The success of Palatin Technologies depends heavily on its ability to advance its clinical trials, obtain regulatory approvals, and successfully market its approved drugs. As with all biotech companies, PTN faces inherent risks associated with drug development, including extensive regulatory hurdles, clinical trial uncertainties, and the competitive landscape of the pharmaceutical industry.
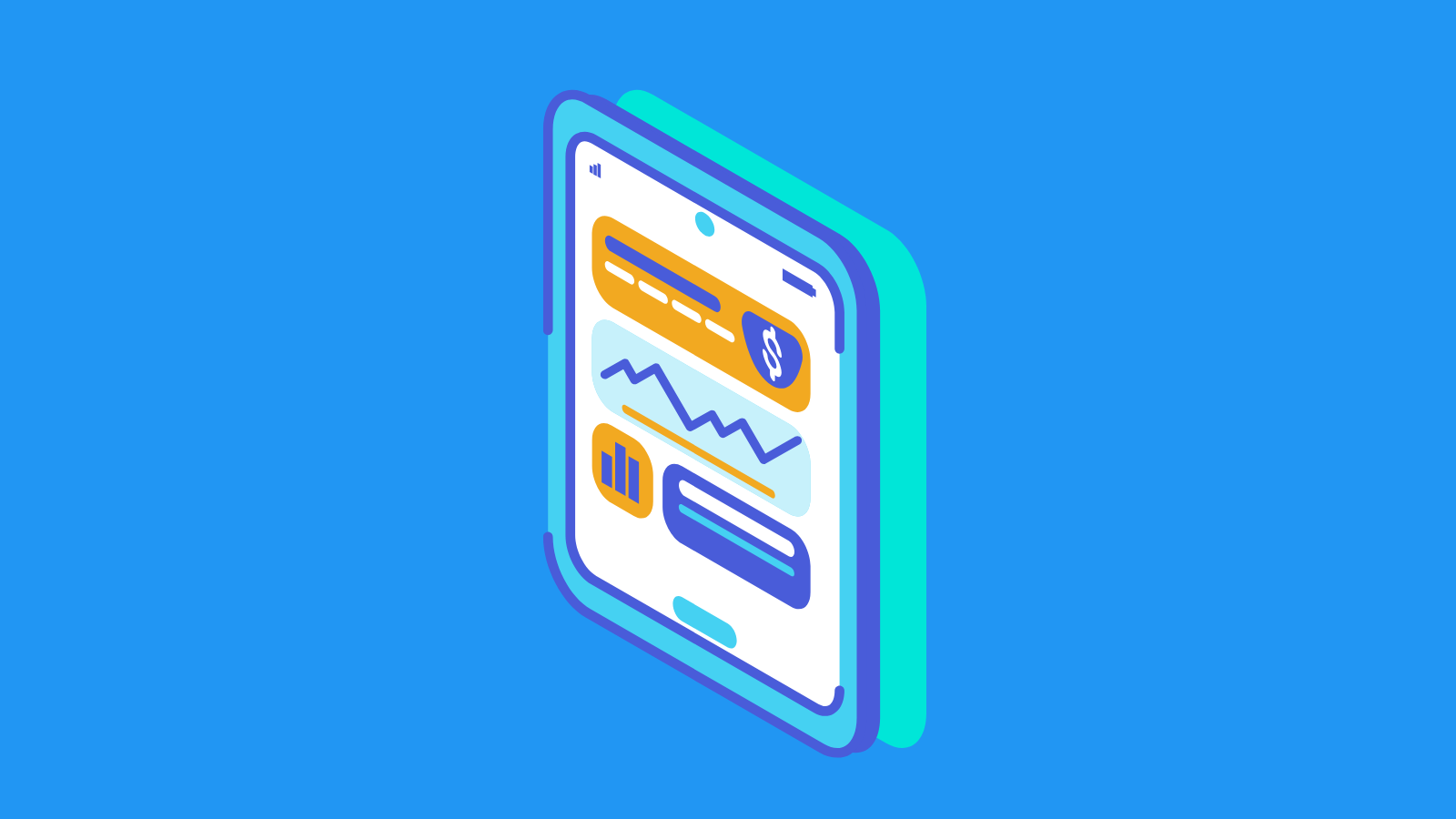
PTN Stock Prediction Model: A Data Science and Economic Approach
The development of a robust prediction model for Palatin Technologies Inc. (PTN) stock necessitates a multifaceted approach, integrating both technical and fundamental analysis alongside macroeconomic considerations. Our model will leverage a machine learning framework, likely a blend of recurrent neural networks (RNNs), specifically Long Short-Term Memory (LSTM) networks, and potentially gradient boosting algorithms. RNNs are well-suited for time-series data like stock prices, allowing them to capture temporal dependencies and patterns. The inputs to the model will be comprehensive, encompassing historical PTN stock performance data (volume, open, close, high, low prices), along with financial statements like quarterly earnings reports, revenue, and cash flow. Sentiment analysis of news articles, social media, and analyst reports will be incorporated to gauge market sentiment. Economic indicators such as interest rates, inflation, and industry-specific trends (biotechnology sector performance) will also form critical features, providing a broader context for the stock's valuation.
Feature engineering is paramount for model performance. This involves transforming raw data into informative variables. Examples include calculating technical indicators like Moving Averages, Relative Strength Index (RSI), and Bollinger Bands from price data. From financial statements, we will derive metrics such as Earnings Per Share (EPS), Price-to-Earnings (P/E) ratio, and debt-to-equity ratios. Text data from news and social media will be pre-processed using techniques like Natural Language Processing (NLP) to extract sentiment scores. Feature selection will be a critical step, employing techniques like Recursive Feature Elimination and feature importance from tree-based models to identify the most impactful variables. To mitigate the risk of overfitting and ensure generalizability, we will employ techniques like cross-validation and regularization. The model's hyperparameters will be meticulously tuned using methods like grid search or Bayesian optimization, optimizing for appropriate forecasting metrics such as Mean Absolute Error (MAE) or Root Mean Squared Error (RMSE).
The final model will provide a probabilistic forecast, estimating not only the expected direction of PTN stock movement but also the associated confidence intervals. We will continuously monitor and retrain the model with new data to maintain its accuracy and account for changing market dynamics. The output of the model will be delivered in a user-friendly dashboard for internal decision-making, and alongside the forecast, the dashboard will include a breakdown of the key drivers influencing the prediction, and a risk assessment section to allow informed decision making by considering various external factors. This iterative process, combining advanced machine learning with robust economic understanding, will allow our model to provide actionable insights into PTN's stock performance.
```
ML Model Testing
n:Time series to forecast
p:Price signals of Palatin Technologies Inc. stock
j:Nash equilibria (Neural Network)
k:Dominated move of Palatin Technologies Inc. stock holders
a:Best response for Palatin Technologies Inc. target price
For further technical information as per how our model work we invite you to visit the article below:
How do KappaSignal algorithms actually work?
Palatin Technologies Inc. Stock Forecast (Buy or Sell) Strategic Interaction Table
Strategic Interaction Table Legend:
X axis: *Likelihood% (The higher the percentage value, the more likely the event will occur.)
Y axis: *Potential Impact% (The higher the percentage value, the more likely the price will deviate.)
Z axis (Grey to Black): *Technical Analysis%
Palatin Technologies Inc. Financial Outlook and Forecast
The financial outlook for PLIN, a biotechnology company focused on melanocortin receptor-targeted therapeutics, presents a complex landscape with both promising opportunities and significant challenges. Currently, the company's primary focus revolves around its lead product, Vyleesi, an on-demand treatment for hypoactive sexual desire disorder (HSDD) in premenopausal women. Revenue generation hinges almost entirely on Vyleesi sales, making the drug's commercial performance the most critical factor influencing the company's near-term financial health. Recent sales figures have fluctuated, and are impacted by various factors, including competition, insurance coverage, and patient awareness. PLIN is dependent on effectively marketing and positioning Vyleesi in a competitive pharmaceutical environment. Additionally, the company continues to invest in research and development, which is a significant expense and impacts profitability, yet is crucial for its long-term success. These investments focus on clinical trials for other potential indications for its melanocortin receptor platform, including areas like metabolic disorders and ophthalmology. These additional programs represent the potential for diversification and future revenue streams, but also come with the inherent risks associated with drug development, including high costs, uncertain clinical trial results, and regulatory hurdles.
The company's financial forecast largely depends on the trajectory of Vyleesi's market share and on successful execution of its development pipeline. The immediate forecast would be influenced by marketing strategies, including payer relations, and strategic partnerships. PLIN needs to achieve consistent revenue growth from Vyleesi to ensure its financial stability. Success is hinged on securing broader insurance coverage and generating greater patient awareness of the product. The company will be reliant on its collaborations and licensing deals. The licensing agreements for Vyleesi in international markets are important for revenue generation, although PLIN will receive royalties instead of directly handling distribution. The outlook for its other development programs is tied to the results of clinical trials and regulatory approvals. If successful, these programs can diversify its revenue streams and reduce reliance on Vyleesi. The market's perception of PLIN's progress in its clinical programs, like its metabolic programs, is essential in the company's valuation.
Longer term, PLIN's financial prospects hinge on its ability to translate its research and development efforts into marketable products and manage its debt load effectively. The company has faced challenges in the past, and has implemented measures to manage its cash resources to finance its operations. Prudent financial management will be critical to extend its runway, while it focuses on building revenue. Building strategic relationships with other pharmaceutical companies for drug development and commercialization offers PLIN options. These collaborations could provide valuable financial support, broaden its reach, and help reduce the financial risks inherent in drug development. The competitive landscape is also a key factor for PLIN. The successful development of its pipeline and its ongoing marketing efforts for Vyleesi are significant. The company is at risk if sales of Vyleesi stagnate or if any of its clinical programs fail.
Predicting a positive outlook for PLIN is contingent on several factors. The successful execution of its marketing strategy for Vyleesi, positive results from its development pipeline, strategic partnerships, and effective financial management are all critical. The risks to this positive outlook are substantial. Market competition, especially within the sexual health sector, is fierce. Delays or failures in clinical trials could severely impact the company's prospects. Regulatory hurdles and potential unfavorable rulings also pose risks. Furthermore, its success depends on securing additional funding. Although PLIN's platform technology represents potential, achieving commercial success will require overcoming hurdles. Therefore, while potential for upside exists, the financial future is inherently risky and dependent on successful execution of its business plan and ongoing developments within its pipeline.
```
Rating | Short-Term | Long-Term Senior |
---|---|---|
Outlook | Baa2 | Ba3 |
Income Statement | Baa2 | Ba2 |
Balance Sheet | Baa2 | B2 |
Leverage Ratios | B2 | B2 |
Cash Flow | Baa2 | B3 |
Rates of Return and Profitability | Ba3 | Baa2 |
*Financial analysis is the process of evaluating a company's financial performance and position by neural network. It involves reviewing the company's financial statements, including the balance sheet, income statement, and cash flow statement, as well as other financial reports and documents.
How does neural network examine financial reports and understand financial state of the company?
References
- Alexander, J. C. Jr. (1995), "Refining the degree of earnings surprise: A comparison of statistical and analysts' forecasts," Financial Review, 30, 469–506.
- Sutton RS, Barto AG. 1998. Reinforcement Learning: An Introduction. Cambridge, MA: MIT Press
- E. Altman, K. Avrachenkov, and R. N ́u ̃nez-Queija. Perturbation analysis for denumerable Markov chains with application to queueing models. Advances in Applied Probability, pages 839–853, 2004
- Burgess, D. F. (1975), "Duality theory and pitfalls in the specification of technologies," Journal of Econometrics, 3, 105–121.
- E. Collins. Using Markov decision processes to optimize a nonlinear functional of the final distribution, with manufacturing applications. In Stochastic Modelling in Innovative Manufacturing, pages 30–45. Springer, 1997
- N. B ̈auerle and A. Mundt. Dynamic mean-risk optimization in a binomial model. Mathematical Methods of Operations Research, 70(2):219–239, 2009.
- Holland PW. 1986. Statistics and causal inference. J. Am. Stat. Assoc. 81:945–60