AUC Score :
Short-term Tactic1 :
Dominant Strategy :
Time series to forecast n:
ML Model Testing : Transfer Learning (ML)
Hypothesis Testing : Paired T-Test
Surveillance : Major exchange and OTC
1Short-term revised.
2Time series is updated based on short-term trends.
Key Points
KLA's stock demonstrates potential for moderate growth, fueled by sustained demand in the semiconductor industry and expansion into advanced packaging solutions. However, this growth is tempered by cyclicality inherent in the semiconductor sector, leading to potential downturns during industry corrections. Further risks include increased competition from rivals like ASML and Lam Research, supply chain disruptions that could impede production, and macroeconomic uncertainties impacting overall capital expenditure budgets within the industry. Positive catalysts include continued technological advancements and strategic partnerships, but investors should carefully weigh these prospects against the potential for volatility and the cyclical nature of the chip market.About KLA Corporation
KLA Corporation (KLA) is a leading global supplier of process control and yield management solutions for the semiconductor and related nanoelectronics industries. The company provides equipment and services that are critical for manufacturing microchips, enabling the production of increasingly complex and advanced electronic devices. KLA's technologies are used throughout the semiconductor manufacturing process, from wafer fabrication to final testing, to ensure the quality and performance of integrated circuits.
KLA offers a broad portfolio of products and services, including inspection and metrology systems, software, and process control solutions. Its equipment helps detect and analyze defects, measure critical dimensions, and optimize manufacturing processes to improve yield, reliability, and efficiency. KLA serves a diverse customer base, including semiconductor manufacturers, equipment suppliers, and research institutions, supporting the continuous advancement of the technology driving modern electronics.
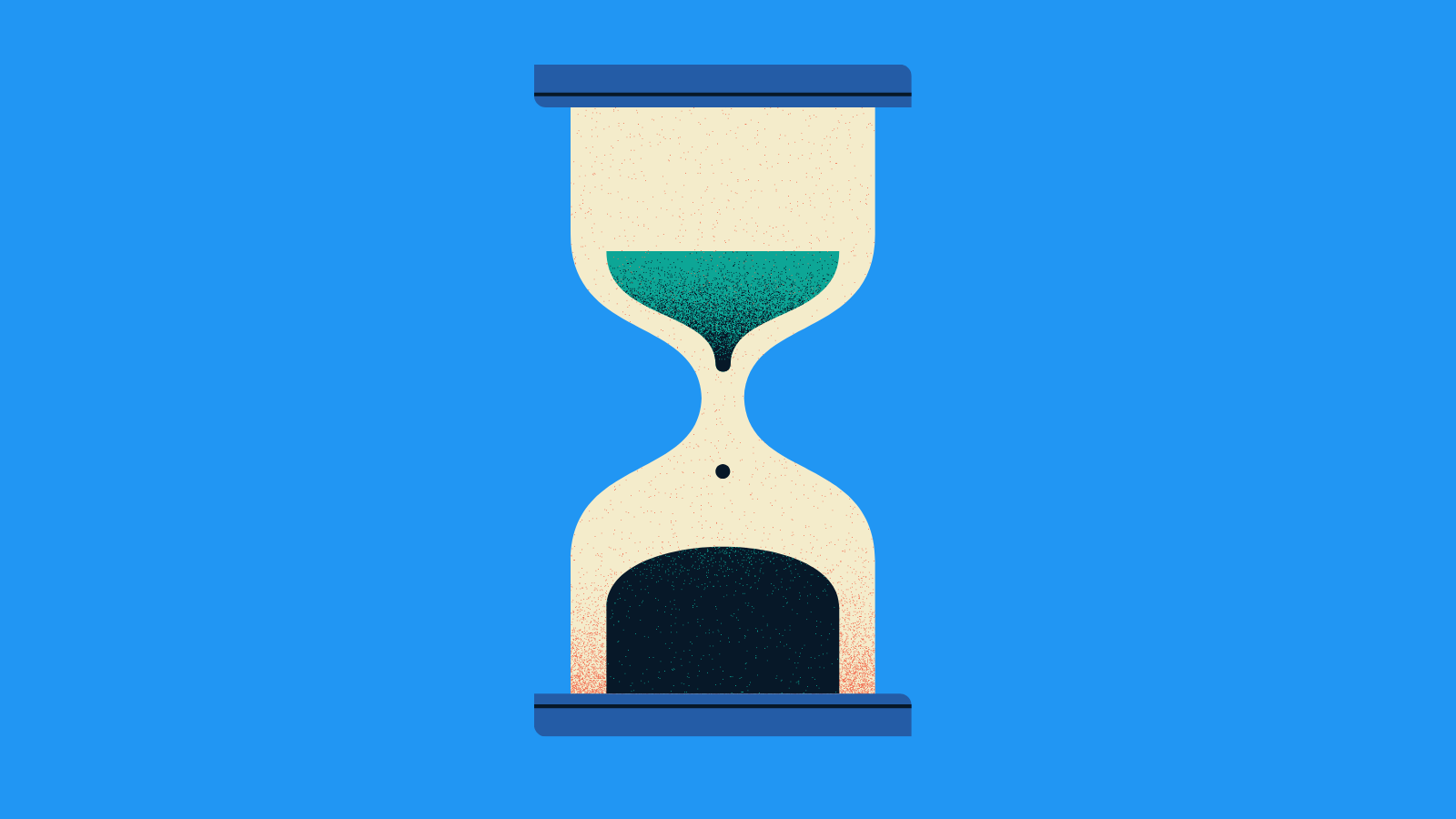
KLAC Stock Forecast Model: A Data Science and Economics Approach
Our team, comprising data scientists and economists, has developed a machine learning model to forecast the future performance of KLA Corporation Common Stock (KLAC). The model integrates a diverse range of features reflecting both macroeconomic conditions and company-specific factors. We employ time-series analysis techniques, including Recurrent Neural Networks (RNNs) with Long Short-Term Memory (LSTM) cells, to capture the inherent temporal dependencies in stock price movements. These models are particularly adept at processing sequential data and identifying patterns that might elude traditional statistical methods. Furthermore, the model incorporates external data sources such as industry reports, semiconductor equipment market trends, and geopolitical risk indices to provide a comprehensive view of the forces shaping KLAC's prospects. Feature engineering plays a critical role, where we transform raw data into meaningful inputs for the model, including technical indicators like moving averages and momentum oscillators.
The model's architecture is designed to handle both structured and unstructured data. Macroeconomic indicators such as GDP growth, inflation rates, and interest rates are included as fundamental inputs, reflecting the broader economic environment. We also incorporate data reflecting the health of the semiconductor industry, including manufacturing output and capacity utilization, as KLAC is a key player in that domain. Furthermore, the model considers financial data from KLAC itself, including revenue, earnings per share, debt levels, and R&D spending, which directly impact its growth and profitability. Regularization techniques are employed during training to prevent overfitting, ensuring the model generalizes well to unseen data. Model performance will be regularly evaluated using metrics such as mean absolute error (MAE) and root mean squared error (RMSE) and will be validated using out-of-sample data.
To provide a robust and reliable forecast, our team focuses on model interpretability and continuous improvement. We employ techniques to understand how each feature impacts the model's predictions. This includes feature importance analysis, sensitivity analysis, and visualization techniques to provide actionable insights. Furthermore, the model will be continuously updated, leveraging the latest available data, and retrained periodically to adapt to changing market dynamics. This proactive approach, combined with rigorous backtesting and validation procedures, allows us to offer a realistic and valuable forecast for KLAC's future performance. The final output will include not only a point forecast but also a confidence interval to reflect the uncertainty in market predictions.
ML Model Testing
n:Time series to forecast
p:Price signals of KLA Corporation stock
j:Nash equilibria (Neural Network)
k:Dominated move of KLA Corporation stock holders
a:Best response for KLA Corporation target price
For further technical information as per how our model work we invite you to visit the article below:
How do KappaSignal algorithms actually work?
KLA Corporation Stock Forecast (Buy or Sell) Strategic Interaction Table
Strategic Interaction Table Legend:
X axis: *Likelihood% (The higher the percentage value, the more likely the event will occur.)
Y axis: *Potential Impact% (The higher the percentage value, the more likely the price will deviate.)
Z axis (Grey to Black): *Technical Analysis%
KLA Corporation Financial Outlook and Forecast
The financial outlook for KLA, a leading provider of process control and yield management solutions for the semiconductor and related industries, is generally positive. This optimistic view stems from several key factors driving demand. The continued growth of the semiconductor industry, fueled by advancements in artificial intelligence, data centers, automotive electronics, and 5G technology, provides a strong underpinning for KLA's business. These advancements require increasingly sophisticated and complex semiconductor manufacturing processes, which directly benefit KLA. The company's advanced inspection and metrology tools are essential for identifying and correcting defects, ensuring high yield rates, and optimizing manufacturing efficiency. Furthermore, the company has a strong customer base that ensures a steady stream of revenue.
KLA's forecast is further enhanced by its position within a market characterized by high barriers to entry. KLA possesses a significant technological advantage due to its strong research and development capabilities and a long history of innovation. The company continually invests in advanced technologies to maintain its leadership position. Moreover, KLA's customer relationships are deep and long-standing, as customers rely heavily on the company's tools and expertise throughout their manufacturing processes. These factors create a competitive moat, shielding KLA from aggressive competitors. The current macroeconomic environment, with its evolving dynamics in the semiconductor industry, presents both opportunities and challenges. Strong demand in specific segments, particularly advanced logic and memory chips, is expected to drive growth. Geographic factors are also playing a part, and KLA's presence in Asia is expected to remain strong.
The company's strategic focus on innovation, customer relationships, and market share expansion is expected to support continued financial success. KLA's investments in cutting-edge technologies, such as EUV inspection and advanced packaging solutions, are well-aligned with the evolving needs of its customers. The company's strong balance sheet and efficient capital allocation strategy provide flexibility for strategic acquisitions and share repurchases. These strategic decisions are essential for maintaining a competitive advantage in a dynamic environment. KLA's management team has demonstrated a track record of sound financial management and disciplined execution. This focus on long-term value creation should drive continued positive financial performance.
In conclusion, the outlook for KLA is positive, supported by secular trends in the semiconductor industry and the company's strong market position. Continued revenue and earnings growth are anticipated. However, this positive outlook is subject to certain risks. The cyclical nature of the semiconductor industry, geopolitical tensions, and supply chain disruptions could negatively impact the company's performance. Furthermore, the increasing competition within the process control and yield management space presents a challenge. Despite these risks, KLA's strong fundamentals and strategic focus position it well for continued success. The prediction is for sustained growth with a potential for outpacing the broader market, but investors must closely monitor the evolving macroeconomic and competitive landscapes.
Rating | Short-Term | Long-Term Senior |
---|---|---|
Outlook | B1 | Ba1 |
Income Statement | Ba2 | B2 |
Balance Sheet | B2 | Baa2 |
Leverage Ratios | Baa2 | Ba3 |
Cash Flow | C | Baa2 |
Rates of Return and Profitability | Ba3 | Ba2 |
*Financial analysis is the process of evaluating a company's financial performance and position by neural network. It involves reviewing the company's financial statements, including the balance sheet, income statement, and cash flow statement, as well as other financial reports and documents.
How does neural network examine financial reports and understand financial state of the company?
References
- Burgess, D. F. (1975), "Duality theory and pitfalls in the specification of technologies," Journal of Econometrics, 3, 105–121.
- Chernozhukov V, Chetverikov D, Demirer M, Duflo E, Hansen C, Newey W. 2017. Double/debiased/ Neyman machine learning of treatment effects. Am. Econ. Rev. 107:261–65
- Zeileis A, Hothorn T, Hornik K. 2008. Model-based recursive partitioning. J. Comput. Graph. Stat. 17:492–514 Zhou Z, Athey S, Wager S. 2018. Offline multi-action policy learning: generalization and optimization. arXiv:1810.04778 [stat.ML]
- Lai TL, Robbins H. 1985. Asymptotically efficient adaptive allocation rules. Adv. Appl. Math. 6:4–22
- Mnih A, Kavukcuoglu K. 2013. Learning word embeddings efficiently with noise-contrastive estimation. In Advances in Neural Information Processing Systems, Vol. 26, ed. Z Ghahramani, M Welling, C Cortes, ND Lawrence, KQ Weinberger, pp. 2265–73. San Diego, CA: Neural Inf. Process. Syst. Found.
- A. Tamar and S. Mannor. Variance adjusted actor critic algorithms. arXiv preprint arXiv:1310.3697, 2013.
- Z. Wang, T. Schaul, M. Hessel, H. van Hasselt, M. Lanctot, and N. de Freitas. Dueling network architectures for deep reinforcement learning. In Proceedings of the International Conference on Machine Learning (ICML), pages 1995–2003, 2016.