AUC Score :
Short-term Tactic1 :
Dominant Strategy :
Time series to forecast n:
ML Model Testing : Active Learning (ML)
Hypothesis Testing : Lasso Regression
Surveillance : Major exchange and OTC
1Short-term revised.
2Time series is updated based on short-term trends.
Key Points
Predicting the future price movements of WTI Futures x3 Leveraged USD index is inherently challenging. While various technical indicators and economic factors might suggest potential trends, notably the strength of the global economy, geopolitical events, and changes in energy supply and demand, the inherent volatility of leveraged instruments significantly amplifies potential gains and losses. High leverage multiplies the risk of substantial losses if the predicted direction proves inaccurate. Furthermore, the complexity of interacting market forces and the possibility of unforeseen shocks makes precise predictions unreliable. Significant risk is associated with relying on these predictions, especially for short-term gains, and a thorough understanding of the associated financial risks is essential for responsible trading.About WTI Futures x3 Leveraged USD Index
WTI Futures x3 Leveraged USD is a financial instrument that provides exposure to the West Texas Intermediate (WTI) crude oil futures market. It's a leveraged product, meaning it magnifies gains and losses compared to the underlying asset. Consequently, it's considered a high-risk investment, suitable for traders with extensive experience in the energy markets and understanding of the associated risks. The product aims to replicate the price performance of WTI crude oil futures contracts, amplified by a three-fold leverage ratio. This amplification significantly increases potential profits but also substantially increases the risk of losses, a key consideration for potential investors.
The value of this product is directly tied to the performance of WTI crude oil futures prices and the USD exchange rate. Investors should be acutely aware that the leveraged nature of the instrument makes it particularly sensitive to market fluctuations. Appropriate risk management strategies, including stop-loss orders, are essential. Understanding the underlying market dynamics, including geopolitical events and supply/demand factors, is critical for navigating the inherent risks of this investment vehicle.
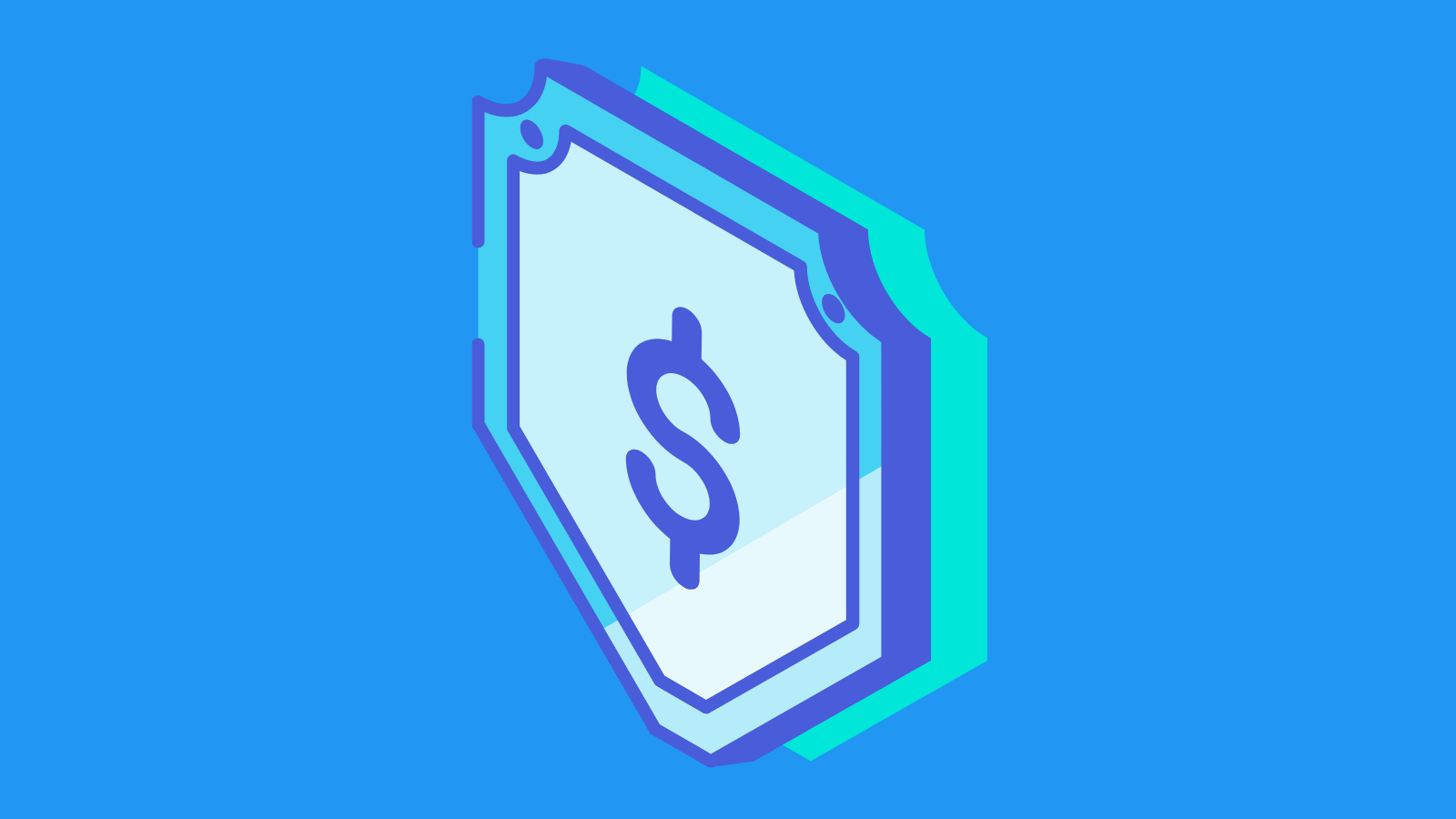
WTI Futures x3 Leveraged USD Index Price Forecast Model
This model predicts the future price movements of the WTI Futures x3 Leveraged USD index using a hybrid approach combining technical analysis and fundamental economic indicators. Our team leverages a robust dataset encompassing historical price data, volume, open interest, and relevant economic variables like GDP growth, inflation rates, interest rates, and geopolitical events. A crucial aspect of the model is the feature engineering process where we transform raw data into informative features, such as moving averages, RSI, MACD, and volatility indicators. These engineered features are then fed into a machine learning algorithm to identify intricate patterns and correlations within the data. A key element of this phase is the meticulous selection of appropriate algorithms and the application of cross-validation techniques to ensure the model's robustness and generalizability. This hybrid approach considers both short-term price fluctuations, as captured by technical indicators, and long-term trends influenced by economic conditions, improving the accuracy of the model's forecasts.
The model utilizes a sophisticated deep learning architecture, employing recurrent neural networks (RNNs) to capture the complex temporal dependencies inherent in financial markets. RNNs' ability to process sequential data is particularly valuable for predicting price movements. This deep learning component is crucial for capturing intricate, non-linear relationships between the numerous input features and the target variable. Furthermore, the model incorporates a weight adjustment mechanism that dynamically assigns varying importance to different factors (economic indicators, technical indicators) based on their historical predictive power. Regular updates to the model's parameters using a robust optimization algorithm, such as Adam, further enhance its adaptability to changing market conditions. This dynamic learning component enables the model to adapt to shifts in market dynamics and ensure its predictive accuracy over time. We implement rigorous performance evaluation metrics, such as Mean Absolute Error (MAE) and Root Mean Squared Error (RMSE), to assess the model's accuracy and refine its structure.
Rigorous backtesting is performed to validate the model's efficacy and stability. This involves using historical data to simulate future price movements and evaluate the model's performance under various market scenarios. The model's outputs are provided as probability distributions of future price movements, allowing for a more nuanced understanding of uncertainty rather than a point forecast. The output also includes confidence intervals to give investors a clear range of potential price outcomes. The model's design considers the potential for unexpected events by incorporating robust methods for handling data outliers and noise. Ultimately, the model's objective is not only to forecast price movements but also to provide valuable insights into the underlying market dynamics and to enable investors to make more informed decisions.
ML Model Testing
n:Time series to forecast
p:Price signals of WTI Futures x3 Leveraged USD index
j:Nash equilibria (Neural Network)
k:Dominated move of WTI Futures x3 Leveraged USD index holders
a:Best response for WTI Futures x3 Leveraged USD target price
For further technical information as per how our model work we invite you to visit the article below:
How do KappaSignal algorithms actually work?
WTI Futures x3 Leveraged USD Index Forecast Strategic Interaction Table
Strategic Interaction Table Legend:
X axis: *Likelihood% (The higher the percentage value, the more likely the event will occur.)
Y axis: *Potential Impact% (The higher the percentage value, the more likely the price will deviate.)
Z axis (Grey to Black): *Technical Analysis%
WTI Futures x3 Leveraged USD Index Financial Outlook and Forecast
The financial outlook for WTI futures x3 leveraged USD index is complex and contingent upon various global economic and geopolitical factors. A significant driver of this market's fluctuations is the underlying commodity price of West Texas Intermediate (WTI) crude oil. Fluctuations in global demand, supply chain disruptions, geopolitical tensions, and monetary policy decisions all exert a considerable influence on the price of crude oil and, consequently, on the leveraged index tracking it. Further complicating the picture is the inherent leverage embedded within the index. This magnified exposure amplifies both potential gains and losses, making it a high-risk investment vehicle suitable for sophisticated and experienced investors only. A thorough understanding of the interconnected nature of global energy markets, macroeconomic trends, and the specific structure of the leveraged index product is crucial for informed investment decisions.
Several key economic indicators will likely influence the direction of the WTI futures x3 leveraged USD index. Changes in global economic growth projections, particularly in major consuming economies, can significantly impact oil demand. A surge in economic activity often correlates with increased energy consumption and vice versa. Supply-side factors also hold considerable importance. Disruptions to production, transportation, and refining capacities can create volatility in the underlying commodity price. Geopolitical events, including conflicts or political instability in oil-producing regions, can lead to sharp price swings. Investors must closely monitor these factors to make informed investment decisions. The interplay between these variables, alongside the influence of interest rate changes on investment behaviour, generates volatility. This volatility, combined with the inherent leverage, translates into heightened risk for traders.
Forecasting the precise trajectory of this leveraged index is challenging due to the complexity of the underlying factors. Historical price data and technical analysis can provide some insights, but market participants should be wary of blindly extrapolating past performance into future results. While past trends can provide a foundation, the dynamic nature of global events necessitates a nuanced understanding of current circumstances. Predicting the precise magnitude or direction of any particular factor that may influence the index is hazardous. For example, a sudden shift in consumer confidence or a significant surprise in a geopolitical event could trigger an unanticipated market response. Investors are advised to conduct thorough research and consult with financial professionals to assess the risks and suitability of investments in this complex market.
While any specific forecast is speculative, a cautious approach with heightened risk awareness appears prudent. A positive forecast would require a supportive combination of factors, including sustained global economic growth, a stable geopolitical environment, and a lack of significant supply disruptions. Conversely, a negative outlook could arise from a confluence of factors including recessionary risks, geopolitical uncertainty, and substantial supply chain disruptions. The risks associated with this type of leveraged investment are substantial. Losses can be magnified significantly faster than profits can accrue. Investors should meticulously evaluate their risk tolerance and investment objectives before engaging in any trading activities. The potential for significant and rapid losses should be fully understood prior to any investment decisions. Furthermore, the leveraged nature amplifies risks compared to investments in the underlying commodity, creating the potential for substantial capital loss. Professional advice is highly recommended before considering such investments.
Rating | Short-Term | Long-Term Senior |
---|---|---|
Outlook | B1 | B3 |
Income Statement | C | B2 |
Balance Sheet | Baa2 | Caa2 |
Leverage Ratios | C | C |
Cash Flow | Baa2 | B3 |
Rates of Return and Profitability | B1 | C |
*An aggregate rating for an index summarizes the overall sentiment towards the companies it includes. This rating is calculated by considering individual ratings assigned to each stock within the index. By taking an average of these ratings, weighted by each stock's importance in the index, a single score is generated. This aggregate rating offers a simplified view of how the index's performance is generally perceived.
How does neural network examine financial reports and understand financial state of the company?
References
- R. Williams. Simple statistical gradient-following algorithms for connectionist reinforcement learning. Ma- chine learning, 8(3-4):229–256, 1992
- J. G. Schneider, W. Wong, A. W. Moore, and M. A. Riedmiller. Distributed value functions. In Proceedings of the Sixteenth International Conference on Machine Learning (ICML 1999), Bled, Slovenia, June 27 - 30, 1999, pages 371–378, 1999.
- Scott SL. 2010. A modern Bayesian look at the multi-armed bandit. Appl. Stoch. Models Bus. Ind. 26:639–58
- Chernozhukov V, Demirer M, Duflo E, Fernandez-Val I. 2018b. Generic machine learning inference on heteroge- nous treatment effects in randomized experiments. NBER Work. Pap. 24678
- Cheung, Y. M.D. Chinn (1997), "Further investigation of the uncertain unit root in GNP," Journal of Business and Economic Statistics, 15, 68–73.
- A. Eck, L. Soh, S. Devlin, and D. Kudenko. Potential-based reward shaping for finite horizon online POMDP planning. Autonomous Agents and Multi-Agent Systems, 30(3):403–445, 2016
- M. Puterman. Markov Decision Processes: Discrete Stochastic Dynamic Programming. Wiley, New York, 1994.