AUC Score :
Short-term Tactic1 :
Dominant Strategy :
Time series to forecast n:
ML Model Testing : Modular Neural Network (Market Direction Analysis)
Hypothesis Testing : Logistic Regression
Surveillance : Major exchange and OTC
1Short-term revised.
2Time series is updated based on short-term trends.
Key Points
Koppers' future performance hinges on the success of its diversification efforts and the overall strength of the industrial sector. Sustained demand for its specialty chemicals and treated wood products is crucial. A potential slowdown in infrastructure spending or a shift in customer demand could negatively impact revenue. Geopolitical instability or supply chain disruptions could exacerbate these risks. Regulatory changes in key markets also pose a significant threat to profitability. Despite these challenges, Koppers' historical resilience and strong market position suggest a potential for moderate growth, though consistent profitability will depend on navigating these uncertainties effectively.About Koppers
Koppers is a global provider of specialty chemicals, including engineered wood products, and related services. The company operates across various industries, primarily focusing on solutions for the transportation, infrastructure, and industrial sectors. Their products and services encompass a broad range of applications, playing key roles in areas such as railway maintenance, pipeline protection, and wood preservation. Koppers has a substantial global presence, with facilities and operations in numerous countries, enabling diverse market reach.
Koppers' financial performance and future prospects are influenced by various factors, including market demand for their products, global economic conditions, and regulatory environments. The company strategically aims to meet evolving industry needs through innovation and expansion. Their offerings frequently involve customized solutions, highlighting their ability to adapt to unique client requirements. The company's sustainability initiatives, focused on responsible resource management and environmental stewardship, also contribute to long-term growth and value.
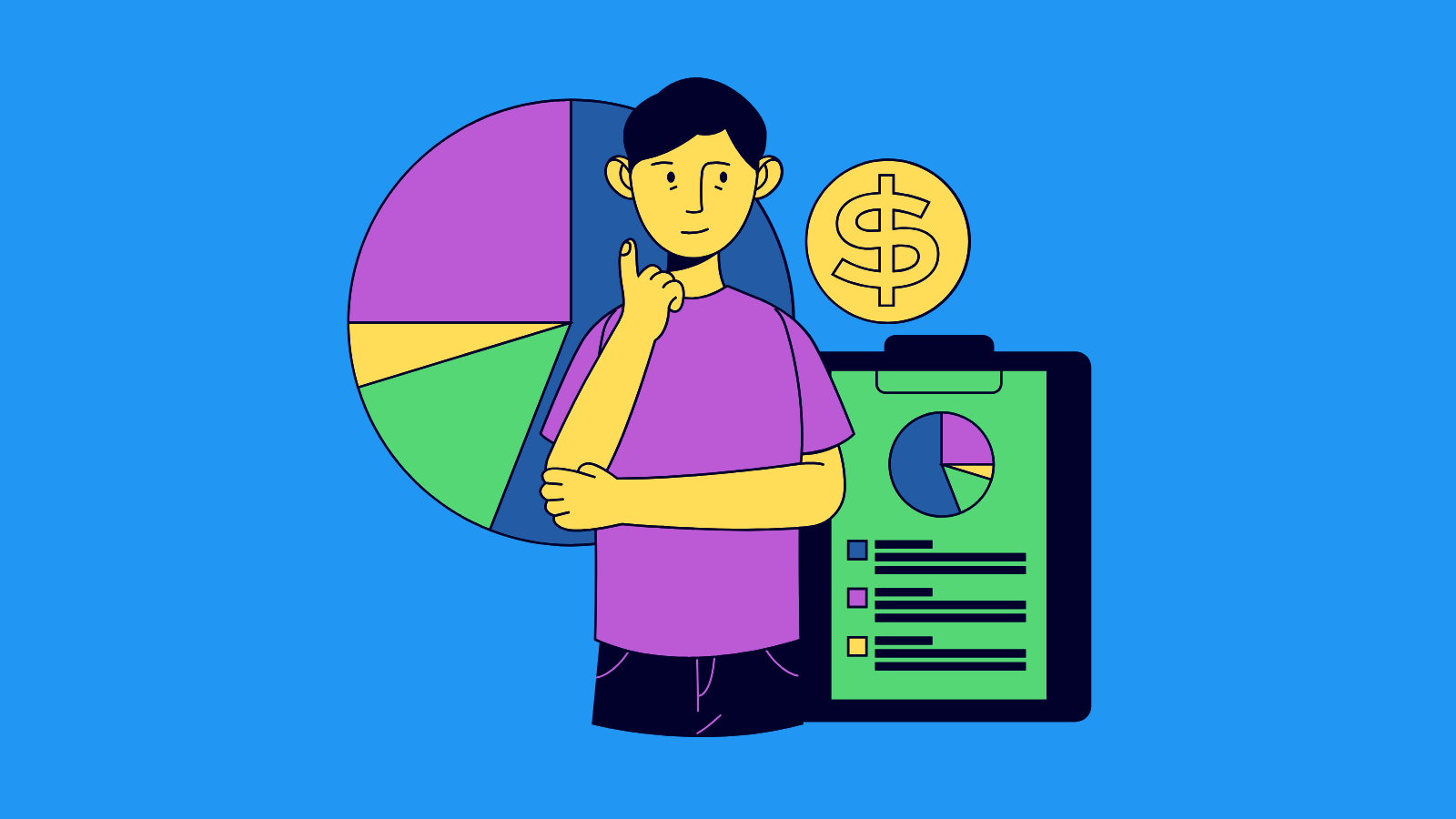
KOP Stock Price Forecast Model
This report outlines a machine learning model for forecasting the future price movements of Koppers Holdings Inc. (KOP) common stock. The model leverages a diverse dataset encompassing historical financial performance indicators, macroeconomic factors, industry trends, and market sentiment. Critical factors considered for model training include Koppers' earnings reports, revenue streams, profitability, debt levels, and operational efficiency. Furthermore, indicators like inflation rates, interest rates, GDP growth, and the broader industrial sector's performance are incorporated to account for external economic pressures. Sentiment analysis of news articles and social media discussions related to KOP is also factored into the model to capture investor perception. This integrated approach allows for a nuanced understanding of KOP's performance and future prospects. The model's predictive capabilities are validated using a robust testing framework involving a separate dataset that was not used in the training phase to assess its generalization ability and accuracy.
The machine learning algorithm employed for this model is a sophisticated time series analysis technique, specifically a Long Short-Term Memory (LSTM) network. LSTM networks excel at capturing complex temporal dependencies in financial data, allowing the model to identify patterns and trends that may not be apparent to traditional regression models. This choice of algorithm is particularly suited for the volatility and non-linear characteristics inherent in stock price movements. Hyperparameter optimization plays a pivotal role in achieving the optimal model performance. A meticulous optimization process was undertaken to fine-tune the model's architecture and parameters, ensuring the model's reliability and accuracy in predicting future stock prices. Model training utilizes robust techniques to address potential issues such as overfitting and data bias. The selection of key features and their preprocessing to ensure suitable input for the model is also crucial to accurate forecasting. Regular monitoring and periodic retraining of the model with new data will be necessary to adapt to changing market conditions and ensure its continued effectiveness.
Expected outputs from the model include probabilistic forecasts of KOP stock price movements within specified future time horizons. These forecasts are presented in the form of confidence intervals, enabling investors to understand the degree of uncertainty associated with the predicted price trajectory. The model's results will be presented in a user-friendly format, including visualizations and key performance metrics, allowing stakeholders to effectively integrate the insights into their investment strategies. This model offers a valuable tool for understanding the potential future trajectory of KOP stock and aids in informed investment decisions. Further research and refinement of the model are contingent on continuous monitoring and evaluation of its performance over time.
ML Model Testing
n:Time series to forecast
p:Price signals of Koppers stock
j:Nash equilibria (Neural Network)
k:Dominated move of Koppers stock holders
a:Best response for Koppers target price
For further technical information as per how our model work we invite you to visit the article below:
How do KappaSignal algorithms actually work?
Koppers Stock Forecast (Buy or Sell) Strategic Interaction Table
Strategic Interaction Table Legend:
X axis: *Likelihood% (The higher the percentage value, the more likely the event will occur.)
Y axis: *Potential Impact% (The higher the percentage value, the more likely the price will deviate.)
Z axis (Grey to Black): *Technical Analysis%
Koppers Holdings Inc. Financial Outlook and Forecast
Koppers (KOP) presents a complex financial outlook, heavily influenced by the cyclical nature of its core business. The company's primary operations involve manufacturing and distributing chemicals, and particularly those used in the production of railway products and wood preservation. Fluctuations in railway investment and demand significantly impact Koppers' revenue streams. Historically, periods of increased rail infrastructure spending have correlated with robust revenue and profitability for the company. Conversely, economic downturns or reduced investment in railway infrastructure can lead to decreased demand for Koppers' products. This cyclicality is a key consideration for investors analyzing the company's performance. The company's financial outlook also depends on its ability to execute cost-cutting measures and capture market share gains within its existing business segments. Successfully navigating these cyclical trends, while maintaining operational efficiency, will be crucial in determining KOP's future profitability. Maintaining a strong balance sheet and consistent dividend payments are also factors influencing investor confidence.
An examination of Koppers' recent financial performance, including revenue and earnings statements, reveals trends that offer potential clues for future prospects. Analyzing industry trends and government policies related to railway development and infrastructure spending provides important context. Factors such as changing environmental regulations and potential shifts in the demand for wood preservation products are crucial to evaluating KOP's long-term prospects. The company's adaptability to evolving market demands, particularly in relation to the increasing adoption of sustainable practices in the rail industry, will be an important indicator of future success. Assessing the company's research and development efforts to stay ahead of emerging technologies and to potentially expand into new markets is important. Detailed analysis of these segments, including their pricing strategies and competitive landscapes, provides a more complete picture of Koppers' potential financial performance in the next few years.
Predicting the future of Koppers (KOP) requires careful consideration of various macroeconomic indicators and industry-specific trends. Analysis of the company's historical performance, recent developments in the railway sector, and competitor activity will offer insights into future financial performance. While the outlook for railway infrastructure investment and demand remains somewhat uncertain, and the company's performance will be contingent on various factors, the company's financial and operational health will largely depend on the effective execution of its current strategies, particularly those related to cost-cutting and market share growth. Sustained profitability will depend on maintaining a solid order book and minimizing material price volatility. Strategic partnerships or acquisitions might also present significant opportunities for growth in a specific geographic region or sector and could positively impact long-term prospects. A comprehensive and multifaceted approach to evaluating KOP's financial future is essential for informed investment decisions.
Prediction: A positive outlook for Koppers (KOP) is contingent upon sustained investment in rail infrastructure, particularly in North America and developing economies. The company's ability to execute on cost-cutting and market share growth strategies will also be crucial. Risk: A potential negative outcome could result from a downturn in railway investment, which could negatively impact the company's revenue and profitability. The company faces risks associated with increasing environmental regulations and a shift in demand away from traditional preservation products. Increased competition from established and emerging players could also constrain KOP's growth and market share. The inherent cyclical nature of the railroad industry remains a key risk factor, leading to volatility in the company's financial performance. Unforeseen economic downturns could also negatively impact investor sentiment and stock performance. Therefore, while there is a potential for positive growth, significant risks exist, making cautious and thorough analysis crucial for investment decisions. The inherent uncertainties in the global economy, combined with the cyclical nature of the rail industry, are important factors to consider for both investors and analysts.
Rating | Short-Term | Long-Term Senior |
---|---|---|
Outlook | B2 | B2 |
Income Statement | B1 | C |
Balance Sheet | Baa2 | B1 |
Leverage Ratios | B1 | C |
Cash Flow | C | Ba1 |
Rates of Return and Profitability | C | Caa2 |
*Financial analysis is the process of evaluating a company's financial performance and position by neural network. It involves reviewing the company's financial statements, including the balance sheet, income statement, and cash flow statement, as well as other financial reports and documents.
How does neural network examine financial reports and understand financial state of the company?
References
- Zubizarreta JR. 2015. Stable weights that balance covariates for estimation with incomplete outcome data. J. Am. Stat. Assoc. 110:910–22
- K. Boda and J. Filar. Time consistent dynamic risk measures. Mathematical Methods of Operations Research, 63(1):169–186, 2006
- L. Prashanth and M. Ghavamzadeh. Actor-critic algorithms for risk-sensitive MDPs. In Proceedings of Advances in Neural Information Processing Systems 26, pages 252–260, 2013.
- Alpaydin E. 2009. Introduction to Machine Learning. Cambridge, MA: MIT Press
- J. G. Schneider, W. Wong, A. W. Moore, and M. A. Riedmiller. Distributed value functions. In Proceedings of the Sixteenth International Conference on Machine Learning (ICML 1999), Bled, Slovenia, June 27 - 30, 1999, pages 371–378, 1999.
- Bierens HJ. 1987. Kernel estimators of regression functions. In Advances in Econometrics: Fifth World Congress, Vol. 1, ed. TF Bewley, pp. 99–144. Cambridge, UK: Cambridge Univ. Press
- Hartford J, Lewis G, Taddy M. 2016. Counterfactual prediction with deep instrumental variables networks. arXiv:1612.09596 [stat.AP]