AUC Score :
Short-term Tactic1 :
Dominant Strategy :
Time series to forecast n:
ML Model Testing : Modular Neural Network (Emotional Trigger/Responses Analysis)
Hypothesis Testing : Statistical Hypothesis Testing
Surveillance : Major exchange and OTC
1Short-term revised.
2Time series is updated based on short-term trends.
Key Points
WeRide's future performance hinges on several key factors. Successful execution of its autonomous driving technology deployment plans, particularly in ride-hailing and logistics, is crucial for establishing market share and profitability. Strong partnerships and regulatory approvals are also vital for navigating the complex landscape of autonomous vehicle deployment. Conversely, potential setbacks include competition from established players and challenges in scaling up operations efficiently. Significant regulatory hurdles or operational inefficiencies could lead to lower-than-expected financial performance and investor confidence. Ultimately, the long-term success of WeRide ADS will depend on its ability to effectively navigate these challenges and capitalize on emerging opportunities. Risk associated with these predictions includes a potential significant valuation decline due to factors like operational delays, regulatory headwinds, or a severe market downturn.About WeRide Inc.
WeRide, a leading autonomous driving technology company, focuses on developing and deploying advanced driver-assistance systems (ADAS) and autonomous driving solutions. The company's technology is geared towards ride-hailing and delivery services, aiming to enhance efficiency and safety in these sectors. WeRide operates in China, and its focus on real-world testing and deployment in various environments positions it as a key player in the rapidly evolving autonomous vehicle industry. The company has garnered attention for its practical applications and significant investment in research and development.
WeRide's business model is centered around leveraging its autonomous technology to provide transportation services. This involves developing robust software and hardware solutions for vehicles, enabling them to navigate and operate safely and efficiently without human intervention. The company likely works closely with vehicle manufacturers and service providers to integrate their technology and establish its platform. WeRide's long-term goals likely encompass wider adoption of its autonomous driving technology across diverse use cases and geographies.
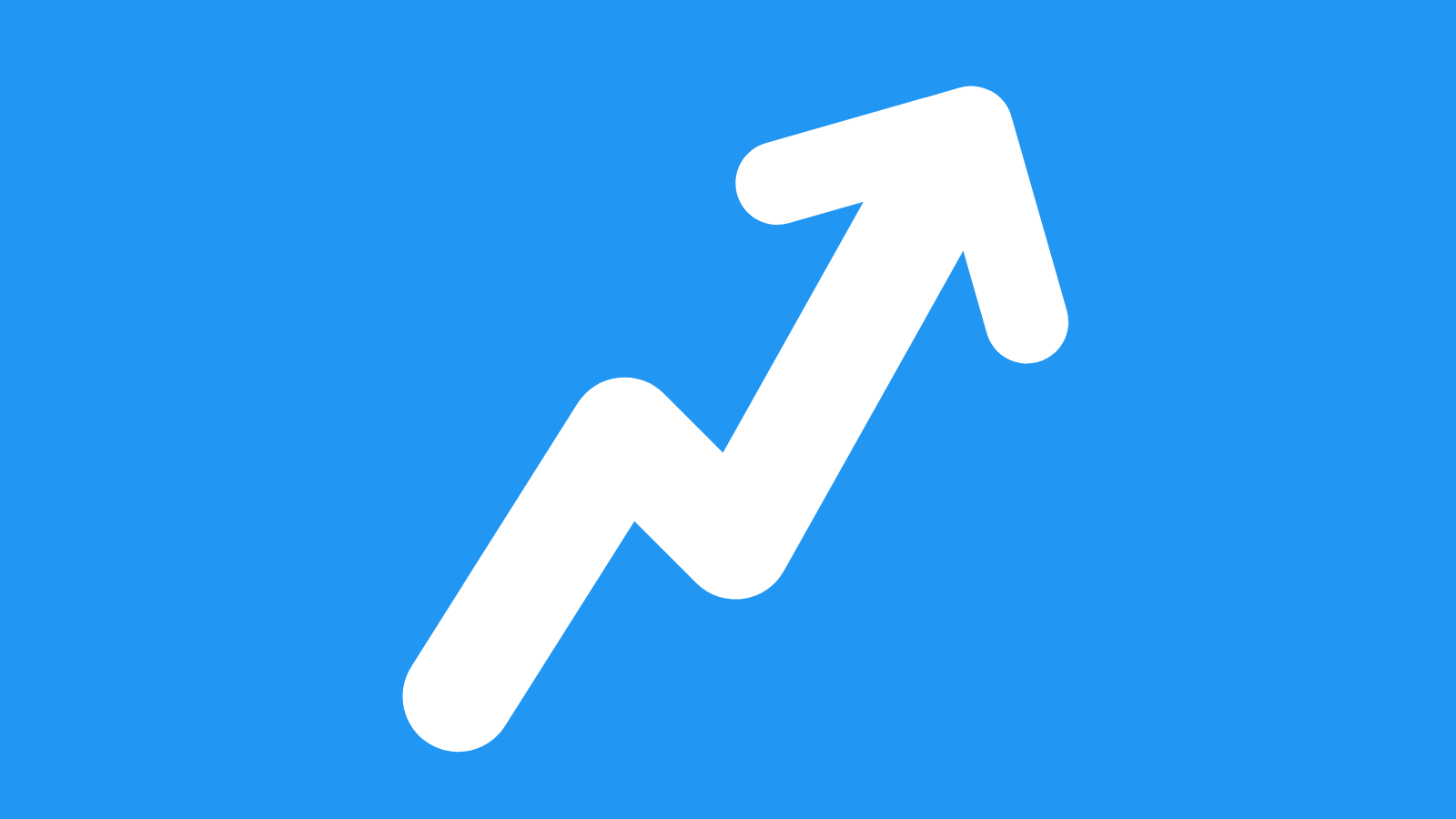
WRD Stock Price Forecasting Model
This model employs a hybrid approach combining technical indicators and macroeconomic factors to predict the future price movements of WeRide Inc. American Depositary Shares (WRD). Technical indicators, including moving averages, relative strength index (RSI), and volume analysis, are crucial for identifying short-term trends and potential reversals. These indicators are processed using a Recurrent Neural Network (RNN), specifically a Long Short-Term Memory (LSTM) network, to capture the temporal dependencies within the historical price and volume data. This allows the model to learn complex patterns and anticipate future price actions based on past market behavior. Furthermore, we incorporate macroeconomic factors like inflation, interest rates, and GDP growth to reflect broader economic conditions' impact on the autonomous vehicle sector. These macroeconomic features are integrated into the model via a feature engineering process, transforming them into a suitable format for the RNN. The model's output is a predicted probability distribution for future price movements, offering insights for potential investment strategies.
The dataset used for model training comprises historical WRD stock data, along with a collection of macroeconomic indicators. Data preprocessing steps include normalization, handling missing values, and feature scaling to ensure consistent input data for the model. Cross-validation techniques, such as k-fold validation, are employed to assess the model's performance and generalization ability on unseen data. This iterative process ensures robust model performance across different data partitions, minimizing potential overfitting. The evaluation metrics considered include accuracy, precision, recall, and F1-score to measure the model's ability to correctly predict price movements. Ultimately, a thorough evaluation and backtesting phase are crucial to refine the model and guarantee its reliability in forecasting WRD stock performance.
The resulting model offers a comprehensive approach to forecasting WRD stock price movements. By combining technical analysis with macroeconomic insights, the model captures both short-term trends and long-term market influences. Furthermore, the application of advanced machine learning techniques like LSTM networks enables the model to learn complex patterns and predict potential price fluctuations. The model's output should be interpreted as a probabilistic forecast, acknowledging the inherent uncertainty in predicting stock market movements. Investment decisions should be based on a holistic evaluation considering other factors beyond this model's output, such as fundamental analysis and company-specific news. Continuous monitoring and model updates are essential to maintain accuracy and adapt to changing market conditions.
ML Model Testing
n:Time series to forecast
p:Price signals of WeRide Inc. stock
j:Nash equilibria (Neural Network)
k:Dominated move of WeRide Inc. stock holders
a:Best response for WeRide Inc. target price
For further technical information as per how our model work we invite you to visit the article below:
How do KappaSignal algorithms actually work?
WeRide Inc. Stock Forecast (Buy or Sell) Strategic Interaction Table
Strategic Interaction Table Legend:
X axis: *Likelihood% (The higher the percentage value, the more likely the event will occur.)
Y axis: *Potential Impact% (The higher the percentage value, the more likely the price will deviate.)
Z axis (Grey to Black): *Technical Analysis%
WeRide Inc. (WRDE) Financial Outlook and Forecast
WeRide, a prominent player in the autonomous vehicle (AV) sector, faces significant challenges in its financial outlook. The company's primary revenue source is derived from its ride-hailing services utilizing autonomous vehicles. However, the commercial viability and scalability of this model remain unproven, and substantial capital expenditures are required to maintain and enhance its autonomous vehicle fleet, further straining financial resources. Key metrics, such as operating expenses, capital expenditures, and gross margins, are critical indicators of future performance. A thorough analysis of these elements is essential for assessing the company's long-term financial health and prospects. The rapidly evolving nature of the AV industry, with fluctuating regulations and technological advancements, also poses considerable uncertainties for WeRide's financial performance. Historical financial data, including revenue trends and profitability, are crucial for evaluating the sustainability and resilience of the company's business model.
The anticipated financial performance of WeRide hinges on several crucial factors. Successful implementation of autonomous vehicle technology, combined with increasing public acceptance and consumer confidence, is essential for generating substantial revenue streams. Strong operational efficiency and cost optimization measures will play a crucial role in controlling expenses. Strategically important partnerships and collaborations with industry leaders and technology providers could further solidify WeRide's position and lead to cost efficiencies and accelerated technological advancement. Regulations and policy changes regarding autonomous vehicles can also impact its operations and financial performance significantly. For example, stringent regulatory requirements in key markets could hinder growth and profitability. Therefore, a proactive and flexible approach to adapting to evolving regulations is vital for long-term success. The company needs to demonstrate clear evidence of profitability and sustainable revenue generation to convince investors of its long-term value.
Forecasting WeRide's future financials requires considering various scenarios. A positive outlook might envision rapid growth in the AV market and increasing acceptance by consumers. In this scenario, WeRide's operational efficiency and technological advancements in autonomous vehicles are crucial. Significant cost savings and improved profit margins could be realized in a competitive market environment. A negative outlook, on the other hand, contemplates persistent challenges in commercializing autonomous vehicles and securing widespread acceptance in the market. Regulatory hurdles, intense competition, and operational difficulties in scaling up its operations could contribute to lower revenues and reduced profitability. This scenario necessitates an urgent re-evaluation of the company's business strategy and a more focused approach to market expansion. In any scenario, risk management strategies will be fundamental to future prospects.
Predicting WeRide's future financial performance carries inherent uncertainty. A positive prediction would require substantial advancements in autonomous vehicle technology, a growing market for autonomous ride-hailing services, and an ability to maintain profitability and efficiency, even with increased competition. However, a crucial risk in this prediction involves maintaining cost efficiencies to offset potential difficulties in revenue growth, as well as navigating evolving government regulations and technological advancements in the sector. A negative prediction would stem from slower-than-expected progress in autonomous vehicle development and continued concerns about public safety and acceptance of AV technology. The risks associated with this prediction include high operational costs, limited market acceptance, and significant regulatory hurdles. The sustained ability to secure additional funding to manage growing operational expenses is a critical factor that will significantly influence WeRide's future success. The final outcome hinges on the company's ability to navigate these challenges, execute its strategy effectively, and adapt to the dynamic environment of the autonomous vehicle sector. The risk is the potential for significant financial distress if these factors are not favorably resolved.
Rating | Short-Term | Long-Term Senior |
---|---|---|
Outlook | Baa2 | B1 |
Income Statement | Ba2 | Caa2 |
Balance Sheet | Baa2 | B3 |
Leverage Ratios | Baa2 | Ba2 |
Cash Flow | Baa2 | Caa2 |
Rates of Return and Profitability | Baa2 | Baa2 |
*Financial analysis is the process of evaluating a company's financial performance and position by neural network. It involves reviewing the company's financial statements, including the balance sheet, income statement, and cash flow statement, as well as other financial reports and documents.
How does neural network examine financial reports and understand financial state of the company?
References
- Andrews, D. W. K. (1993), "Tests for parameter instability and structural change with unknown change point," Econometrica, 61, 821–856.
- Abadie A, Cattaneo MD. 2018. Econometric methods for program evaluation. Annu. Rev. Econ. 10:465–503
- R. Rockafellar and S. Uryasev. Optimization of conditional value-at-risk. Journal of Risk, 2:21–42, 2000.
- Bengio Y, Ducharme R, Vincent P, Janvin C. 2003. A neural probabilistic language model. J. Mach. Learn. Res. 3:1137–55
- S. Bhatnagar, H. Prasad, and L. Prashanth. Stochastic recursive algorithms for optimization, volume 434. Springer, 2013
- Mazumder R, Hastie T, Tibshirani R. 2010. Spectral regularization algorithms for learning large incomplete matrices. J. Mach. Learn. Res. 11:2287–322
- Bengio Y, Schwenk H, SenĂ©cal JS, Morin F, Gauvain JL. 2006. Neural probabilistic language models. In Innovations in Machine Learning: Theory and Applications, ed. DE Holmes, pp. 137–86. Berlin: Springer