AUC Score :
Short-term Tactic1 :
Dominant Strategy :
Time series to forecast n:
ML Model Testing : Deductive Inference (ML)
Hypothesis Testing : Stepwise Regression
Surveillance : Major exchange and OTC
1Short-term revised.
2Time series is updated based on short-term trends.
Key Points
The DJ Commodity Lead index is anticipated to experience moderate fluctuations, likely driven by global economic conditions and supply chain dynamics. Potential upward pressure may be seen from rising demand in key sectors. However, risks include heightened geopolitical uncertainty impacting raw material availability and fluctuating energy prices, potentially leading to volatile price swings. Overall, a cautious outlook is warranted, with the need to closely monitor developments in these interconnected factors to accurately assess future performance.About DJ Commodity Lead Index
The DJ Commodity Index is a benchmark for tracking the performance of various commodities. It's designed to measure the overall movement of commodity prices, encompassing a range of raw materials. The index is widely followed by investors and analysts to assess market sentiment and potential investment opportunities within the commodity sector. Different commodities, such as agricultural products, energy sources, and metals, each contribute to the overall index value, reflecting their relative significance in the global market.
The DJ Commodity Index provides a comprehensive view of commodity market dynamics. It's influenced by numerous factors, including supply and demand fluctuations, geopolitical events, economic growth, and inflation. This multifaceted influence makes the index sensitive to shifts in global conditions and serves as a crucial tool for understanding trends across the diverse commodity landscape.
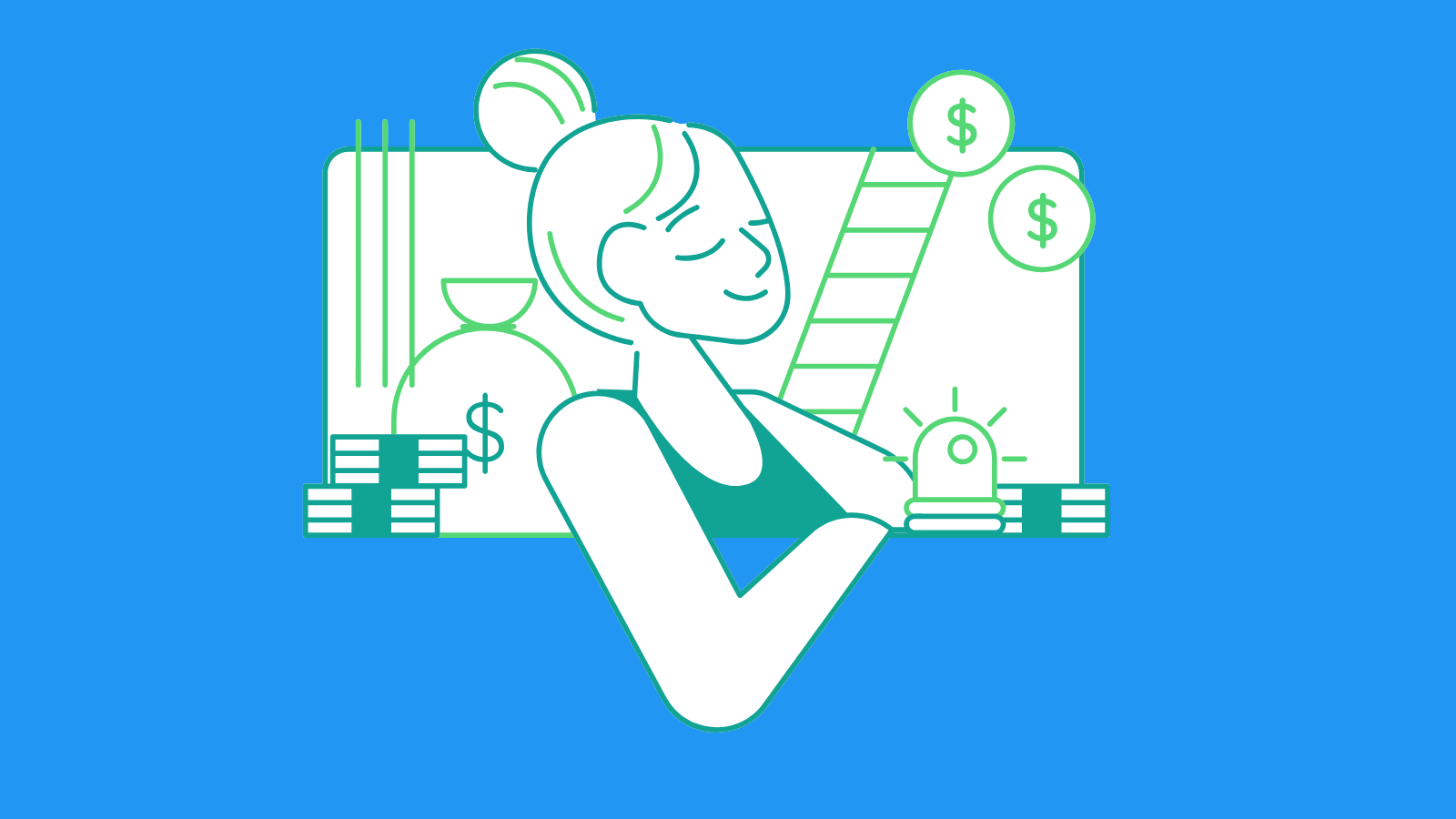
DJ Commodity Lead Index Forecast Model
To predict the future trajectory of the DJ Commodity Lead Index, a machine learning model incorporating diverse economic and market indicators is essential. Our proposed model leverages a multi-layered neural network architecture, specifically a long short-term memory (LSTM) network. This choice is predicated on the time-dependent nature of commodity prices, reflecting trends and patterns often obscured by traditional regression models. The LSTM model's capacity for handling sequential data proves crucial for capturing intricate relationships within the commodity market. Feature engineering plays a vital role. Historical commodity prices, macroeconomic indicators (e.g., GDP growth, inflation rates, interest rates), geopolitical events, and supply/demand dynamics are carefully selected and preprocessed to ensure optimal model performance. Data preprocessing involves handling missing values, scaling numerical features, and one-hot encoding categorical variables to avoid bias and ensure consistency in data representation.
The model's training process employs a robust optimization algorithm such as Adam, meticulously tuned for convergence and minimizing overfitting. A comprehensive evaluation framework is established using cross-validation techniques to assess the model's performance on unseen data. Metrics such as Mean Absolute Error (MAE) and Root Mean Squared Error (RMSE) are employed to evaluate the model's accuracy. Furthermore, the model is subjected to rigorous backtesting using historical data, allowing for an assessment of its predictive power over time. The model's output will be a forecasted value of the DJ Commodity Lead Index, along with a confidence interval reflecting the uncertainty surrounding the prediction. Rigorous analysis of the model's error patterns and identification of significant explanatory variables are key steps to ensure robustness and interpretability. These analyses will guide refinements to the model's architecture and feature set, potentially incorporating additional indicators.
Deploying the model involves automating the data collection, preprocessing, training, and forecasting processes to ensure real-time predictions. Regular monitoring and retraining of the model are crucial for maintaining accuracy, given the dynamic nature of the commodity market. This iterative approach allows for adaptation to evolving market conditions and incorporation of new data sources, improving the model's predictive performance over time. The resultant model will provide a valuable tool for investment strategies in the commodity sector, allowing for informed decision-making based on data-driven insights about the DJ Commodity Lead Index and potential market shifts.
ML Model Testing
n:Time series to forecast
p:Price signals of DJ Commodity Lead index
j:Nash equilibria (Neural Network)
k:Dominated move of DJ Commodity Lead index holders
a:Best response for DJ Commodity Lead target price
For further technical information as per how our model work we invite you to visit the article below:
How do KappaSignal algorithms actually work?
DJ Commodity Lead Index Forecast Strategic Interaction Table
Strategic Interaction Table Legend:
X axis: *Likelihood% (The higher the percentage value, the more likely the event will occur.)
Y axis: *Potential Impact% (The higher the percentage value, the more likely the price will deviate.)
Z axis (Grey to Black): *Technical Analysis%
DJ Commodity Lead Index Financial Outlook and Forecast
The DJ-UBS Commodity Index, a broad-based measure of commodity prices, is a crucial indicator of global economic health and investor sentiment. Its movements reflect shifts in supply and demand dynamics, geopolitical events, and investor expectations. Analyzing the DJ Commodity Lead Index requires a comprehensive understanding of the complex interplay of these factors. The current market environment, characterized by inflation, fluctuating interest rates, and ongoing geopolitical uncertainties, significantly impacts the index's performance. Evaluating current trends in production, consumption, and global economic growth is essential for forecasting future price movements and determining the overall financial outlook for this index.
Looking ahead, several key factors are expected to influence the DJ Commodity Lead Index. The strength of global economic growth will play a significant role, influencing demand for raw materials and finished goods. Supply chain disruptions and geopolitical tensions can also significantly impact commodity prices. Changes in consumer spending patterns and technological advancements could alter the demand-supply equation, leading to both short-term and long-term price fluctuations. Additionally, central bank policies and interest rate decisions will directly impact investor sentiment and commodity pricing by affecting borrowing costs and influencing investment strategies. Forecasting the index's performance requires a nuanced understanding of these interconnected factors.
While predicting the precise trajectory of the DJ Commodity Lead Index with certainty is challenging, the outlook offers both positive and negative prospects. Potential upside drivers include increased demand from emerging economies, infrastructure development projects, and technological advancements in various sectors that might drive consumption. Sustainability concerns and initiatives to reduce carbon emissions could also lead to changes in commodity production and consumption patterns, which in turn could affect prices. However, the persistent inflationary environment, coupled with lingering supply chain uncertainties, could lead to volatility and create a challenging investment climate. The long-term performance of the index is also inextricably linked to the sustainable development goals and initiatives focused on circular economy models. The ongoing implications of these factors on the global energy transition will also bear a strong influence.
The prediction for the DJ Commodity Lead Index is cautiously optimistic, with potential for short-term volatility. The current conditions suggest some recovery and likely positive movements with the caveats. However, the prediction hinges on the interplay of several crucial factors. Risks associated with this prediction include heightened geopolitical instability, prolonged supply chain disruptions, and unexpected changes in global economic conditions. The index may also be impacted by unforeseen events such as natural disasters, sudden shifts in government policies, or unforeseen technological advancements. These risks should be considered when formulating investment strategies, and a diversified approach to investing is crucial to mitigate potential adverse impacts. Investors should carefully assess the specific commodity sectors and their respective risk profiles. Continuous monitoring of economic indicators and market trends is crucial for adjusting investment decisions accordingly.
Rating | Short-Term | Long-Term Senior |
---|---|---|
Outlook | B3 | Ba3 |
Income Statement | Caa2 | Ba1 |
Balance Sheet | B1 | Baa2 |
Leverage Ratios | Baa2 | Baa2 |
Cash Flow | C | C |
Rates of Return and Profitability | C | B2 |
*An aggregate rating for an index summarizes the overall sentiment towards the companies it includes. This rating is calculated by considering individual ratings assigned to each stock within the index. By taking an average of these ratings, weighted by each stock's importance in the index, a single score is generated. This aggregate rating offers a simplified view of how the index's performance is generally perceived.
How does neural network examine financial reports and understand financial state of the company?
References
- Breiman L. 1993. Better subset selection using the non-negative garotte. Tech. Rep., Univ. Calif., Berkeley
- Athey S, Wager S. 2017. Efficient policy learning. arXiv:1702.02896 [math.ST]
- J. Harb and D. Precup. Investigating recurrence and eligibility traces in deep Q-networks. In Deep Reinforcement Learning Workshop, NIPS 2016, Barcelona, Spain, 2016.
- Jiang N, Li L. 2016. Doubly robust off-policy value evaluation for reinforcement learning. In Proceedings of the 33rd International Conference on Machine Learning, pp. 652–61. La Jolla, CA: Int. Mach. Learn. Soc.
- Barkan O. 2016. Bayesian neural word embedding. arXiv:1603.06571 [math.ST]
- Mazumder R, Hastie T, Tibshirani R. 2010. Spectral regularization algorithms for learning large incomplete matrices. J. Mach. Learn. Res. 11:2287–322
- M. Petrik and D. Subramanian. An approximate solution method for large risk-averse Markov decision processes. In Proceedings of the 28th International Conference on Uncertainty in Artificial Intelligence, 2012.