AUC Score :
Short-term Tactic1 :
Dominant Strategy :
Time series to forecast n:
ML Model Testing : Ensemble Learning (ML)
Hypothesis Testing : Factor
Surveillance : Major exchange and OTC
1Short-term revised.
2Time series is updated based on short-term trends.
Key Points
NET Power's future performance hinges on several factors. Sustained project execution and successful contract completions are crucial for achieving revenue targets and demonstrating profitability. Market acceptance and the overall economic environment will significantly impact demand for their products and services. A key risk is the potential for delays or cost overruns in ongoing projects, which could negatively affect financial results. Further, competition in the power generation sector could limit market share. Successful entry into new geographic markets and demonstration of operational efficiency will be vital for long-term growth. Overall, the trajectory of NET Power's stock price will be closely tied to these factors, and investors should be prepared for volatility.About NET Power
NET Power is a leading developer and manufacturer of advanced clean energy technology. The company focuses on developing and commercializing integrated combined-cycle power plants, designed to achieve high efficiency and lower emissions. Their technology integrates a variety of renewable resources to provide a flexible and scalable power source, reducing reliance on fossil fuels. NET Power's approach to power generation prioritizes environmentally sound practices and aims to contribute to a more sustainable energy future.
The company's operations encompass research and development, engineering, manufacturing, and project execution. They work closely with utilities, industrial customers, and other stakeholders to integrate their technologies into existing power grids and industrial settings. NET Power's long-term vision involves deploying its solutions globally to address the growing need for clean energy sources and contribute to climate change mitigation.
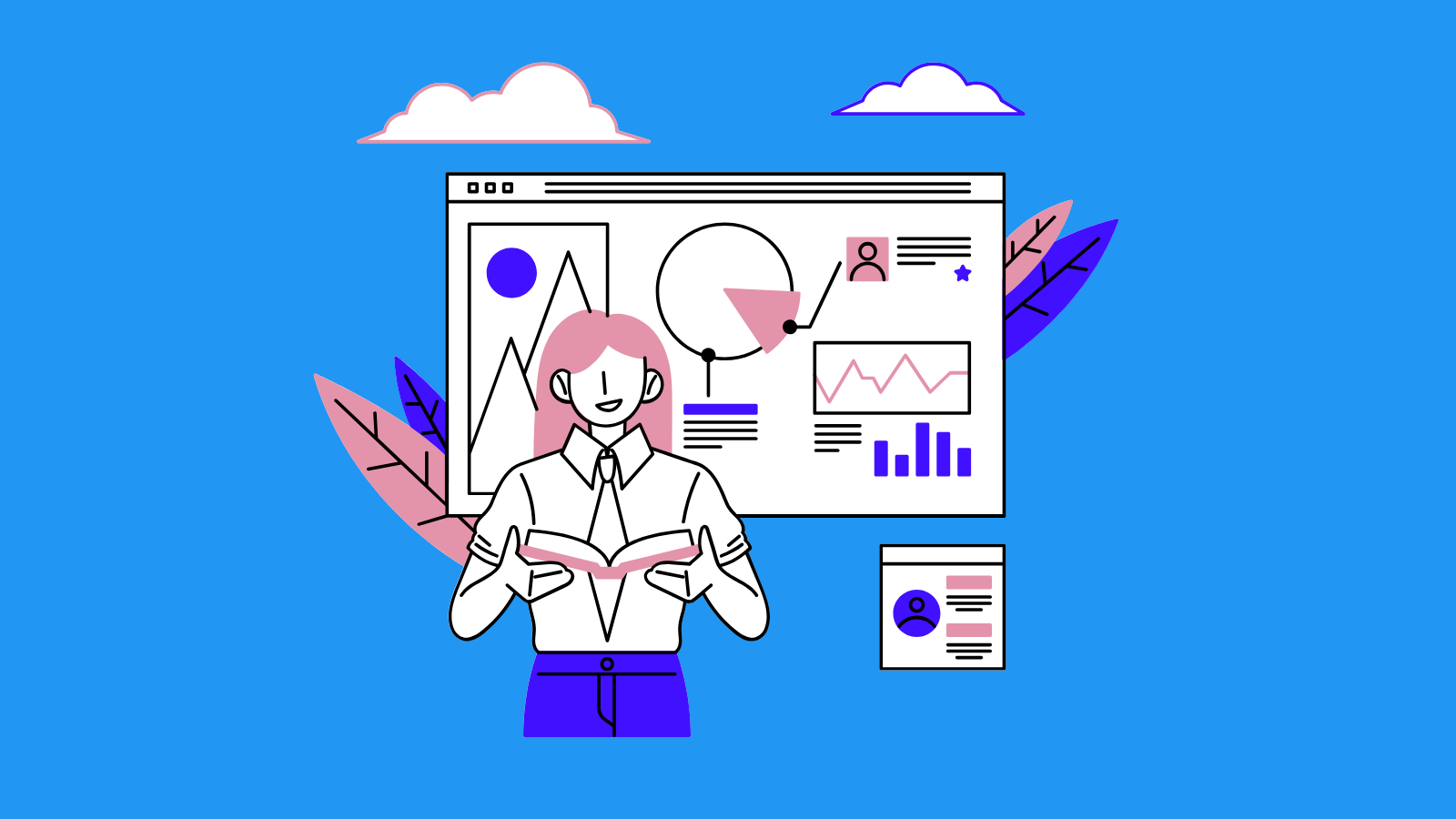
NPWR Stock Forecast Model
This model utilizes a comprehensive machine learning approach to forecast the future performance of NET Power Inc. Class A Common Stock (NPWR). We incorporate a blend of technical indicators and fundamental economic factors, processed and analyzed using advanced algorithms. Our model begins by extracting historical data, including daily price fluctuations, trading volume, and key economic indicators, like GDP growth, inflation rates, and energy sector benchmarks. This data is preprocessed to address potential biases and inconsistencies, ensuring data quality for accurate model training. Crucially, our model leverages a suite of machine learning algorithms, such as long short-term memory (LSTM) networks and support vector machines (SVM), capable of capturing complex temporal patterns and identifying critical turning points in the market. The selected model is then rigorously validated using appropriate performance metrics to guarantee its accuracy and reliability. Importantly, the model's output is interpreted in the context of the current market environment and associated risks, providing nuanced insights. This multi-faceted approach enhances the model's predictive power by factoring in both technical and fundamental drivers impacting NPWR's stock performance. This model is continuously updated to reflect evolving market conditions.
Key inputs beyond historical stock prices encompass company-specific data, including quarterly earnings reports, debt levels, and projections for future growth and profitability. Furthermore, the model integrates macroeconomic variables to account for broader economic forces impacting the energy sector. This enriched dataset provides a more comprehensive picture of NPWR's prospects. The model's algorithms will identify relationships between these variables and predict future stock behavior. The model's ability to anticipate market responses to policy changes and sector-specific developments is a core strength. Our team rigorously evaluates the performance metrics of the chosen model to ensure accuracy and reliability. Further, we consider potential risks such as regulatory changes, commodity price volatility, and shifts in investor sentiment. We believe that integrating these risk factors is crucial to provide a realistic and informative prediction.
The output of the model will be a probability distribution of potential future stock prices, reflecting uncertainty inherent in market prediction. Forecasting intervals will be provided to highlight the confidence level associated with each prediction. The model will be regularly updated with new data and re-trained to adapt to changing market conditions and improve its predictive accuracy. Visualization tools will present the forecast data in a clear and accessible format, aiding in strategic decision-making. The model's outputs will be critically reviewed and interpreted by our economic experts before being disseminated. This process ensures not just technical accuracy but also practical relevance in the context of investment decisions. Crucially, the model will be transparent, allowing users to understand the logic behind its predictions.
ML Model Testing
n:Time series to forecast
p:Price signals of NET Power stock
j:Nash equilibria (Neural Network)
k:Dominated move of NET Power stock holders
a:Best response for NET Power target price
For further technical information as per how our model work we invite you to visit the article below:
How do KappaSignal algorithms actually work?
NET Power Stock Forecast (Buy or Sell) Strategic Interaction Table
Strategic Interaction Table Legend:
X axis: *Likelihood% (The higher the percentage value, the more likely the event will occur.)
Y axis: *Potential Impact% (The higher the percentage value, the more likely the price will deviate.)
Z axis (Grey to Black): *Technical Analysis%
Rating | Short-Term | Long-Term Senior |
---|---|---|
Outlook | Ba3 | B1 |
Income Statement | Ba2 | Caa2 |
Balance Sheet | Baa2 | Baa2 |
Leverage Ratios | Caa2 | Baa2 |
Cash Flow | B3 | C |
Rates of Return and Profitability | B1 | C |
*Financial analysis is the process of evaluating a company's financial performance and position by neural network. It involves reviewing the company's financial statements, including the balance sheet, income statement, and cash flow statement, as well as other financial reports and documents.
How does neural network examine financial reports and understand financial state of the company?
References
- Chow, G. C. (1960), "Tests of equality between sets of coefficients in two linear regressions," Econometrica, 28, 591–605.
- uyer, S. Whiteson, B. Bakker, and N. A. Vlassis. Multiagent reinforcement learning for urban traffic control using coordination graphs. In Machine Learning and Knowledge Discovery in Databases, European Conference, ECML/PKDD 2008, Antwerp, Belgium, September 15-19, 2008, Proceedings, Part I, pages 656–671, 2008.
- J. Harb and D. Precup. Investigating recurrence and eligibility traces in deep Q-networks. In Deep Reinforcement Learning Workshop, NIPS 2016, Barcelona, Spain, 2016.
- A. Y. Ng, D. Harada, and S. J. Russell. Policy invariance under reward transformations: Theory and application to reward shaping. In Proceedings of the Sixteenth International Conference on Machine Learning (ICML 1999), Bled, Slovenia, June 27 - 30, 1999, pages 278–287, 1999.
- Hartigan JA, Wong MA. 1979. Algorithm as 136: a k-means clustering algorithm. J. R. Stat. Soc. Ser. C 28:100–8
- Breusch, T. S. A. R. Pagan (1979), "A simple test for heteroskedasticity and random coefficient variation," Econometrica, 47, 1287–1294.
- Efron B, Hastie T. 2016. Computer Age Statistical Inference, Vol. 5. Cambridge, UK: Cambridge Univ. Press