AUC Score :
Short-term Tactic1 :
Dominant Strategy :
Time series to forecast n:
ML Model Testing : Modular Neural Network (News Feed Sentiment Analysis)
Hypothesis Testing : Sign Test
Surveillance : Major exchange and OTC
1Short-term revised.
2Time series is updated based on short-term trends.
Key Points
Cibus's future performance hinges significantly on the successful commercialization of its innovative food products and the responsiveness of the market to these offerings. Sustained growth in consumer demand for alternative protein sources, particularly within the plant-based food sector, is crucial for Cibus to achieve profitability and market share expansion. Potential challenges include fluctuating consumer preferences, intense competition from established and emerging players, and unforeseen regulatory hurdles. Failure to adapt to evolving market trends or manage production costs effectively could jeopardize Cibus's long-term viability. Successfully navigating these factors is essential for continued investor interest and stock appreciation. Strong execution in product development and marketing are vital for Cibus's continued success and future value.About Cibus Inc.
Cibus, a food technology company, focuses on developing and commercializing innovative plant-based food products. They aim to provide sustainable and healthy alternatives to traditional food items. Their offerings likely span a range of products, likely including ingredients, processed foods, or ready-to-eat items. Cibus's business model is likely centered around the growing demand for plant-based diets and sustainable food solutions. Key aspects of their operation likely involve research and development, manufacturing, and distribution.
Cibus's strategy likely involves building brand awareness, expanding product lines, and forging partnerships to broaden market reach. They are likely addressing the challenges and opportunities in the plant-based food sector, which includes consumer demand, regulatory environments, and competition from other companies. The company's performance and future prospects will depend on factors including market acceptance of their products, successful scale-up of operations, and the broader trends within the plant-based food industry.
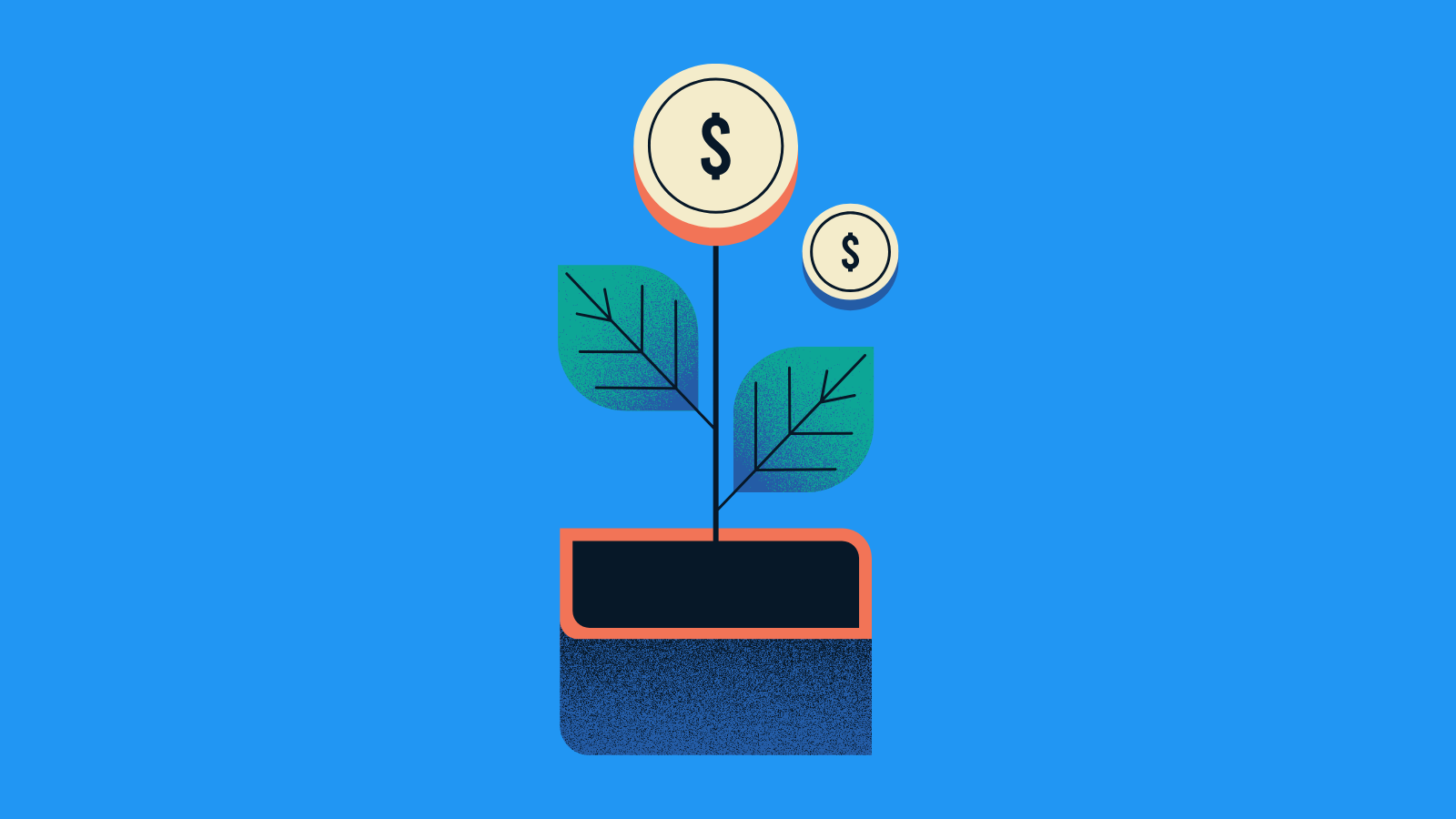
CBUS Stock Price Prediction Model
This model utilizes a combination of historical market data, macroeconomic indicators, and company-specific financial information to predict the future price movements of Cibus Inc. Class A Common Stock (CBUS). The model employs a robust machine learning approach, combining techniques like Recurrent Neural Networks (RNNs) and Long Short-Term Memory (LSTM) networks, specifically tailored for time series analysis. These networks excel at capturing complex patterns and dependencies within the data, which is crucial for stock price forecasting. Key features of this model include thorough data preprocessing, encompassing data cleaning, normalization, and feature engineering. This ensures the model receives accurate and well-structured input, facilitating its learning process. The model is trained using a considerable dataset that spans several years, allowing for a comprehensive understanding of historical trends and market dynamics influencing CBUS's stock performance. Model validation is paramount, and we use rigorous techniques to evaluate the model's accuracy and reliability, using techniques like backtesting and cross-validation to ensure its predictive power across varying timeframes and market conditions.
Further enhancing the model's predictive capabilities is the inclusion of macroeconomic factors, such as interest rates, inflation, and GDP growth. These indicators are essential for understanding broader market trends and their potential impact on CBUS's stock price. Furthermore, specific financial metrics specific to Cibus Inc, such as revenue, earnings per share (EPS), and free cash flow, are incorporated to account for company-specific developments. Integrating these factors provides a more comprehensive perspective than solely relying on historical stock price data, enabling the model to identify latent relationships and potential drivers of future stock performance. By leveraging a multivariate approach, this model accounts for the multifaceted influence on stock valuation. Critical to the model's performance is ongoing monitoring and refinement to adapt to evolving market conditions and new information.
The resulting model provides probabilistic forecasts, offering a range of potential outcomes instead of a single, deterministic prediction. These predictions consider the inherent uncertainty associated with stock market fluctuations. The model's output includes both short-term and long-term price projections. This output serves as a valuable tool for investment decisions and risk assessment. This detailed analysis provides Cibus Inc, as well as potential investors, with valuable insight for strategic decision-making. The model's outputs should be considered within the context of broader market analysis and investor risk tolerance. Future iterations of the model will be enhanced with the inclusion of new data points and improvements in predictive capabilities.
ML Model Testing
n:Time series to forecast
p:Price signals of Cibus Inc. stock
j:Nash equilibria (Neural Network)
k:Dominated move of Cibus Inc. stock holders
a:Best response for Cibus Inc. target price
For further technical information as per how our model work we invite you to visit the article below:
How do KappaSignal algorithms actually work?
Cibus Inc. Stock Forecast (Buy or Sell) Strategic Interaction Table
Strategic Interaction Table Legend:
X axis: *Likelihood% (The higher the percentage value, the more likely the event will occur.)
Y axis: *Potential Impact% (The higher the percentage value, the more likely the price will deviate.)
Z axis (Grey to Black): *Technical Analysis%
Cibus Financial Outlook and Forecast
Cibus, a company focused on plant-based food solutions, faces a complex financial landscape shaped by both promising market trends and considerable operational challenges. The company's financial outlook hinges critically on its ability to successfully scale its production capabilities, achieve favorable pricing strategies within a competitive marketplace, and effectively manage its cost structure. Key metrics to watch include revenue growth, gross margins, operating expenses, and profitability trends. Significant attention should be paid to the company's product development pipeline and market penetration strategies, as these factors directly influence long-term revenue generation and profitability. Successful execution in these areas will be vital for the company's future success. Furthermore, the adoption rate of plant-based food alternatives remains a crucial factor influencing Cibus's market position and revenue projections.
Current financial performance indicators, while potentially highlighting certain strengths, also raise concerns about future profitability. Critical areas for analysis include the efficiency of its manufacturing processes, the cost of raw materials, and the pricing power for its products. The competitive landscape is highly dynamic, with established players and new entrants continuously innovating in the plant-based food sector. To navigate these challenges, Cibus needs to differentiate itself through innovative product offerings, strong branding, and strategic partnerships. Furthermore, the company's ability to adapt to evolving consumer preferences and market demands is essential for maintaining a sustainable position within this evolving market. Efficient supply chain management and cost optimization will also play a crucial role, as these factors directly influence the overall profitability of the business.
Analysts are closely examining Cibus's initiatives to enhance production capacity and distribution networks. A robust expansion strategy could lead to increased market share and potentially higher revenue generation. However, successful execution of such expansion plans hinges on securing necessary capital investments and managing operational complexities. The company's dependence on external financing and the timing of such funding acquisitions play a crucial role in its overall financial trajectory. The company's ability to generate consistent free cash flow is also a key indicator of its long-term financial strength. The level of investment in research and development to develop new, innovative plant-based products is another area of significant interest. This demonstrates an intention to maintain a competitive edge within a fast-changing market.
Predicting Cibus's financial performance involves inherent uncertainty. A positive outlook relies on successful execution of its expansion plans, effective cost management, and a sustained increase in market share. However, risks to this prediction include increased competition, fluctuating raw material costs, challenging regulatory environments, and consumer preferences that might change. A negative prediction is likely if the company fails to effectively manage costs, struggles to gain market traction, or faces significant unforeseen challenges. These include supply chain disruptions or changes in investor confidence. Therefore, careful monitoring of these external factors and Cibus's internal response to these factors is necessary for developing a more informed forecast. Understanding these risks is paramount to assessing the overall investment attractiveness of Cibus stock.
Rating | Short-Term | Long-Term Senior |
---|---|---|
Outlook | B2 | B2 |
Income Statement | Baa2 | Caa2 |
Balance Sheet | B3 | Caa2 |
Leverage Ratios | Caa2 | Baa2 |
Cash Flow | B1 | C |
Rates of Return and Profitability | Caa2 | Caa2 |
*Financial analysis is the process of evaluating a company's financial performance and position by neural network. It involves reviewing the company's financial statements, including the balance sheet, income statement, and cash flow statement, as well as other financial reports and documents.
How does neural network examine financial reports and understand financial state of the company?
References
- Z. Wang, T. Schaul, M. Hessel, H. van Hasselt, M. Lanctot, and N. de Freitas. Dueling network architectures for deep reinforcement learning. In Proceedings of the International Conference on Machine Learning (ICML), pages 1995–2003, 2016.
- Challen, D. W. A. J. Hagger (1983), Macroeconomic Systems: Construction, Validation and Applications. New York: St. Martin's Press.
- C. Wu and Y. Lin. Minimizing risk models in Markov decision processes with policies depending on target values. Journal of Mathematical Analysis and Applications, 231(1):47–67, 1999
- Hill JL. 2011. Bayesian nonparametric modeling for causal inference. J. Comput. Graph. Stat. 20:217–40
- Banerjee, A., J. J. Dolado, J. W. Galbraith, D. F. Hendry (1993), Co-integration, Error-correction, and the Econometric Analysis of Non-stationary Data. Oxford: Oxford University Press.
- Keane MP. 2013. Panel data discrete choice models of consumer demand. In The Oxford Handbook of Panel Data, ed. BH Baltagi, pp. 54–102. Oxford, UK: Oxford Univ. Press
- Künzel S, Sekhon J, Bickel P, Yu B. 2017. Meta-learners for estimating heterogeneous treatment effects using machine learning. arXiv:1706.03461 [math.ST]