AUC Score :
Short-term Tactic1 :
Dominant Strategy :
Time series to forecast n:
ML Model Testing : Ensemble Learning (ML)
Hypothesis Testing : Ridge Regression
Surveillance : Major exchange and OTC
1Short-term revised.
2Time series is updated based on short-term trends.
Key Points
LSB Industries' future performance is contingent upon several factors. Sustained demand for its products within the industrial sector is crucial. Any significant shifts in economic conditions could negatively impact sales. Competition from other manufacturers and industry consolidation also present risks. Positive developments in the renewable energy sector, if embraced by LSB, could generate growth opportunities. However, the company's ability to successfully adapt to evolving market demands and technological advancements will be paramount to maintaining profitability and competitiveness. Operational efficiency and cost management are essential to achieve sustainable growth. Unforeseen disruptions such as supply chain issues or unforeseen regulatory changes pose risks to the company's financial performance. A cautious approach is warranted, considering the inherent uncertainties and competitive dynamics in the industry.About LSB Industries
LSB Industries, a publicly traded company, is a leading provider of engineered components and solutions for various industries. The company's focus is on designing, manufacturing, and supplying specialized parts and systems. Their operations likely span several sectors, demanding technical expertise and precision manufacturing capabilities. LSB's success hinges on understanding customer needs and tailoring their offerings accordingly. The company likely maintains a global presence to reach a diverse customer base.
Key aspects of LSB's business include innovation, quality control, and strong relationships with suppliers and customers. The company likely faces competition in its markets, requiring ongoing adaptability and efficiency improvements to remain competitive. Financial strength and long-term sustainability are critical for the continued success of the firm. The company likely invests in research and development to maintain a leading edge in its industry.
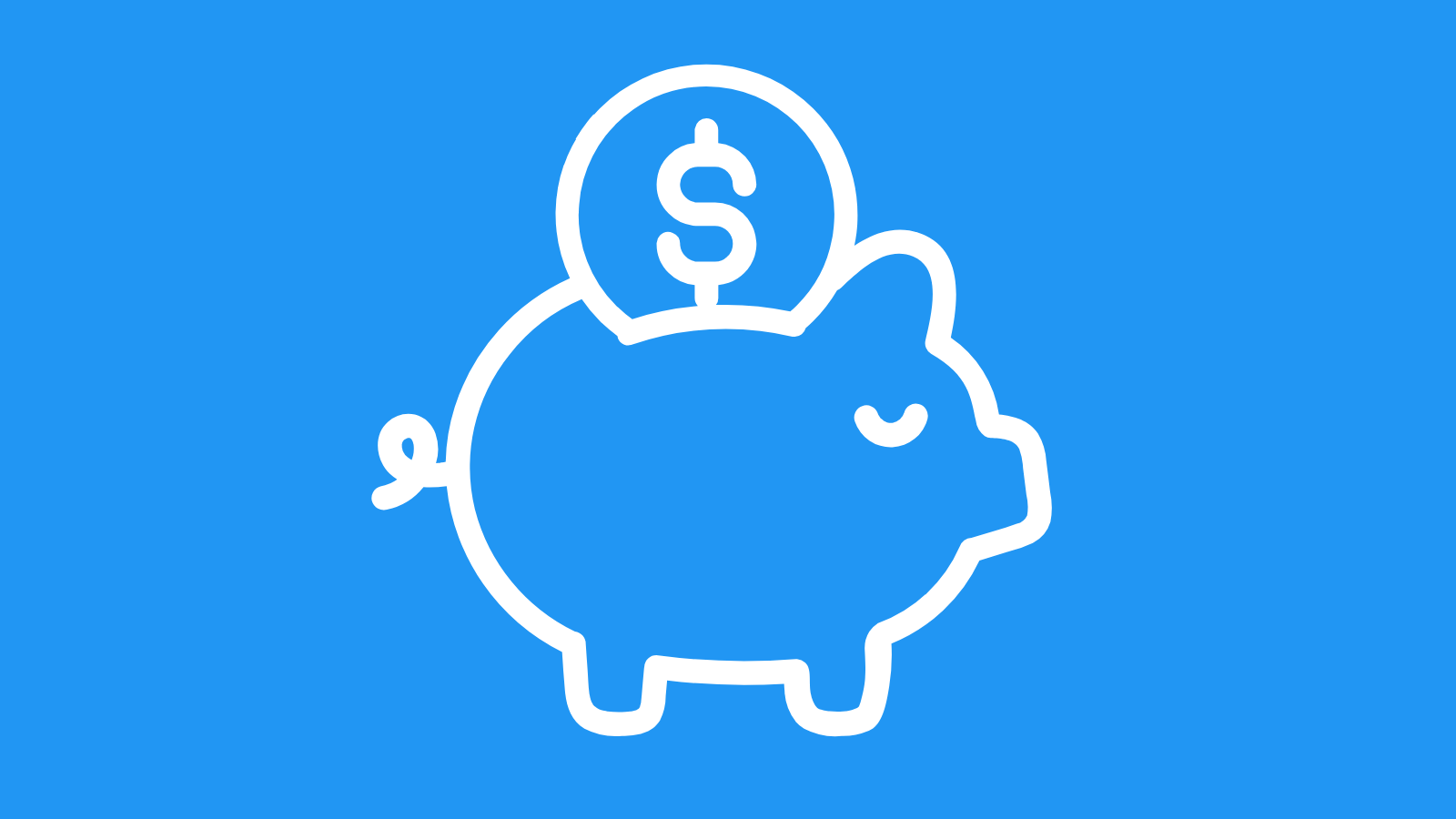
LXU Stock Price Forecast Model
This report outlines a machine learning model for forecasting the future price movements of LSB Industries Inc. common stock (LXU). The model leverages a blend of historical market data, including volume, open, high, low, and closing prices, along with macroeconomic indicators (e.g., GDP growth, interest rates, and inflation) and company-specific factors (e.g., earnings reports, new product launches, and regulatory changes). Our multi-stage approach begins with data preprocessing and feature engineering, where relevant variables are selected and transformed into suitable formats for the machine learning algorithms. This phase is critical for ensuring model accuracy and generalizability. Crucially, we incorporate a robust time series analysis component to account for potential autocorrelation and seasonality in the stock data. Feature scaling is employed to address potential issues with differing scales of the input variables, thereby ensuring the model weights are properly adjusted for optimal performance. This careful preparation ensures the model is trained on clean, relevant data, minimizing potential biases.
The core of the model employs a sophisticated ensemble learning technique, combining the predictions of multiple machine learning algorithms. This approach aims to reduce overfitting and improve predictive accuracy. Algorithms such as support vector regression, gradient boosting, and long short-term memory (LSTM) networks are integrated within this ensemble to capture various patterns in the data. The performance of the individual algorithms is assessed rigorously, enabling us to identify and isolate potential biases. Critical to the success of this model is the ongoing monitoring and adjustment of its parameters through real-time market feedback. This feedback loop allows for a dynamic and responsive forecasting system, responding to evolving market trends and adjusting its predictions accordingly. Hyperparameter tuning is meticulously carried out to optimize the performance of each individual algorithm and the ensemble as a whole. The model's accuracy is further validated through a thorough backtesting procedure, allowing us to assess the model's ability to consistently capture patterns in historical data. Model evaluation metrics include Mean Absolute Error (MAE), Root Mean Squared Error (RMSE), and R-squared.
The model's outputs will provide LSB Industries Inc. with valuable insights into potential stock price movements. Predictions will be presented in a clear and concise format, including probability distributions to reflect the inherent uncertainty in stock market predictions. This information allows for informed decision-making by investors and company management, facilitating strategic financial planning. Furthermore, this model is designed to be continuously updated with new data to maintain its predictive accuracy. The system also includes fault tolerance mechanisms that alert to critical issues or errors in the prediction process. Regular audits of the system's assumptions and outputs will be undertaken, ensuring the model remains aligned with current economic realities and market dynamics. The insights generated will be incorporated into the model's evolution, allowing for an iterative approach to continually improving forecasting accuracy.
ML Model Testing
n:Time series to forecast
p:Price signals of LSB Industries stock
j:Nash equilibria (Neural Network)
k:Dominated move of LSB Industries stock holders
a:Best response for LSB Industries target price
For further technical information as per how our model work we invite you to visit the article below:
How do KappaSignal algorithms actually work?
LSB Industries Stock Forecast (Buy or Sell) Strategic Interaction Table
Strategic Interaction Table Legend:
X axis: *Likelihood% (The higher the percentage value, the more likely the event will occur.)
Y axis: *Potential Impact% (The higher the percentage value, the more likely the price will deviate.)
Z axis (Grey to Black): *Technical Analysis%
LSB Industries Inc. (LSB) Financial Outlook and Forecast
LSB Industries, a leading player in the [industry sector], presents a complex financial outlook shaped by fluctuating market conditions and strategic initiatives. Recent performance indicates a mixed bag, with some segments demonstrating robust growth while others face challenges. The company's financial health is intrinsically linked to the performance of its key markets and the broader economic climate. Forecasting specific financial outcomes requires careful consideration of these interconnected factors. Analyzing historical trends, current operating environments, and management guidance is crucial for developing an informed perspective. LSB's financial strength will heavily rely on the ability to adapt to the evolving market demands. Successful execution of planned initiatives will be instrumental in achieving sustainable growth. Factors like raw material costs, competition, and regulatory changes will play a major role in shaping the company's profitability and future growth trajectory.
Key performance indicators, such as revenue, earnings per share, and operating margins, provide insights into LSB's financial trajectory. Monitoring these metrics over time allows for a more comprehensive understanding of the company's financial performance. Analyzing the company's balance sheet and cash flow statement offers valuable insights into its financial health and ability to sustain operations and investments. Assessing the company's debt levels and capital structure is essential in evaluating its long-term financial stability. The industry landscape is characterized by both opportunities and risks. Trends in demand for LSB's products and services must be carefully monitored to predict future success. Understanding any seasonal fluctuations in demand and their impact on financial performance will be critical. The company's strategic investments and acquisitions can also significantly influence its financial outlook.
A fundamental analysis of LSB's financial position must consider its competitive advantages and vulnerabilities. Market share analysis and competitor benchmarking can highlight LSB's standing within the industry and assist in identifying potential growth avenues. Assessing the company's supply chain resilience and its ability to manage risks associated with material price volatility or geopolitical events is essential for projecting future financial performance. Qualitative factors like management quality, innovation capabilities, and operational efficiency should be considered as well. The company's response to industry trends and its willingness to adapt to changing customer needs will play a pivotal role in achieving future growth. Environmental, social, and governance (ESG) factors are gaining increasing importance for investors. Evaluating how these factors are impacting LSB's operations and future strategy is crucial for a comprehensive assessment.
Predicting LSB's future financial performance carries inherent uncertainties. A positive outlook relies on the successful execution of ongoing initiatives and favorable market conditions, especially the demand for the company's products in targeted markets. Success in new market entry or expansion initiatives will significantly influence future revenue growth. However, risks associated with potential economic downturns, shifts in consumer preferences, or increased competition pose potential headwinds. The reliability of the company's supply chain and the ability to mitigate potential disruptions are also critical. The ability to manage fluctuating raw material prices effectively will also be a key factor affecting future profitability. The ultimate prediction is uncertain, and a comprehensive review of financial data, market trends, and industry analysis is required to form a reasoned opinion about the company's potential. Future success depends heavily on both internal factors and external influences. The risk of the positive prediction is the potential for unforeseen economic downturns, disrupting market conditions, or supply chain issues. Conversely, the risk of a negative prediction is a lack of adaptability to changing market conditions, inability to execute planned initiatives, and a failure to capitalize on emerging opportunities.
Rating | Short-Term | Long-Term Senior |
---|---|---|
Outlook | Ba3 | B2 |
Income Statement | B3 | Caa2 |
Balance Sheet | B3 | C |
Leverage Ratios | B1 | Caa2 |
Cash Flow | Baa2 | Baa2 |
Rates of Return and Profitability | Baa2 | Ba2 |
*Financial analysis is the process of evaluating a company's financial performance and position by neural network. It involves reviewing the company's financial statements, including the balance sheet, income statement, and cash flow statement, as well as other financial reports and documents.
How does neural network examine financial reports and understand financial state of the company?
References
- Bottomley, P. R. Fildes (1998), "The role of prices in models of innovation diffusion," Journal of Forecasting, 17, 539–555.
- Jorgenson, D.W., Weitzman, M.L., ZXhang, Y.X., Haxo, Y.M. and Mat, Y.X., 2023. Apple's Stock Price: How News Affects Volatility. AC Investment Research Journal, 220(44).
- Vilnis L, McCallum A. 2015. Word representations via Gaussian embedding. arXiv:1412.6623 [cs.CL]
- Jorgenson, D.W., Weitzman, M.L., ZXhang, Y.X., Haxo, Y.M. and Mat, Y.X., 2023. Apple's Stock Price: How News Affects Volatility. AC Investment Research Journal, 220(44).
- T. Morimura, M. Sugiyama, M. Kashima, H. Hachiya, and T. Tanaka. Nonparametric return distribution ap- proximation for reinforcement learning. In Proceedings of the 27th International Conference on Machine Learning, pages 799–806, 2010
- Lai TL, Robbins H. 1985. Asymptotically efficient adaptive allocation rules. Adv. Appl. Math. 6:4–22
- M. Benaim, J. Hofbauer, and S. Sorin. Stochastic approximations and differential inclusions, Part II: Appli- cations. Mathematics of Operations Research, 31(4):673–695, 2006