AUC Score :
Short-term Tactic1 :
Dominant Strategy :
Time series to forecast n:
ML Model Testing : Multi-Instance Learning (ML)
Hypothesis Testing : Polynomial Regression
Surveillance : Major exchange and OTC
1Short-term revised.
2Time series is updated based on short-term trends.
Key Points
Golar LNG's future performance is contingent upon several factors. Positive factors include the continued growth of the global LNG market, potentially favorable regulatory environments for LNG projects, and the company's strategic investments in new vessels and technologies. However, negative factors include fluctuating LNG prices, global economic uncertainties, and potential delays or cost overruns in LNG project development. Geopolitical events could also significantly impact the market. The risk of reduced demand or pricing pressures in the LNG sector will impact the profitability of Golar LNG. Operational challenges related to vessel maintenance, crew availability, and potential disruptions to port operations pose further risks. Ultimately, the stock's performance will hinge on the interplay of these interacting factors and the company's ability to adapt to market dynamics.About Golar
Golar LNG is a leading provider of LNG transportation and regasification services globally. The company operates a fleet of LNG carriers, specializing in flexible and efficient solutions for transporting LNG. Golar LNG's operations span various regions, facilitating the movement of LNG to diverse markets. The company is a key player in the global LNG industry, contributing to the efficient and reliable transportation of this vital energy source. Its fleet and infrastructure allow for varied delivery scenarios and flexibility in response to market demands.
Golar LNG's business model centers around chartering and operating LNG carriers and providing regasification services. This strategy positions them as a key facilitator within the global LNG supply chain. The company is dedicated to environmental sustainability and aims to reduce the environmental impact of its operations while meeting market needs. Golar LNG also actively manages its assets and maintains a strong presence in the industry through strategic partnerships and operational excellence.
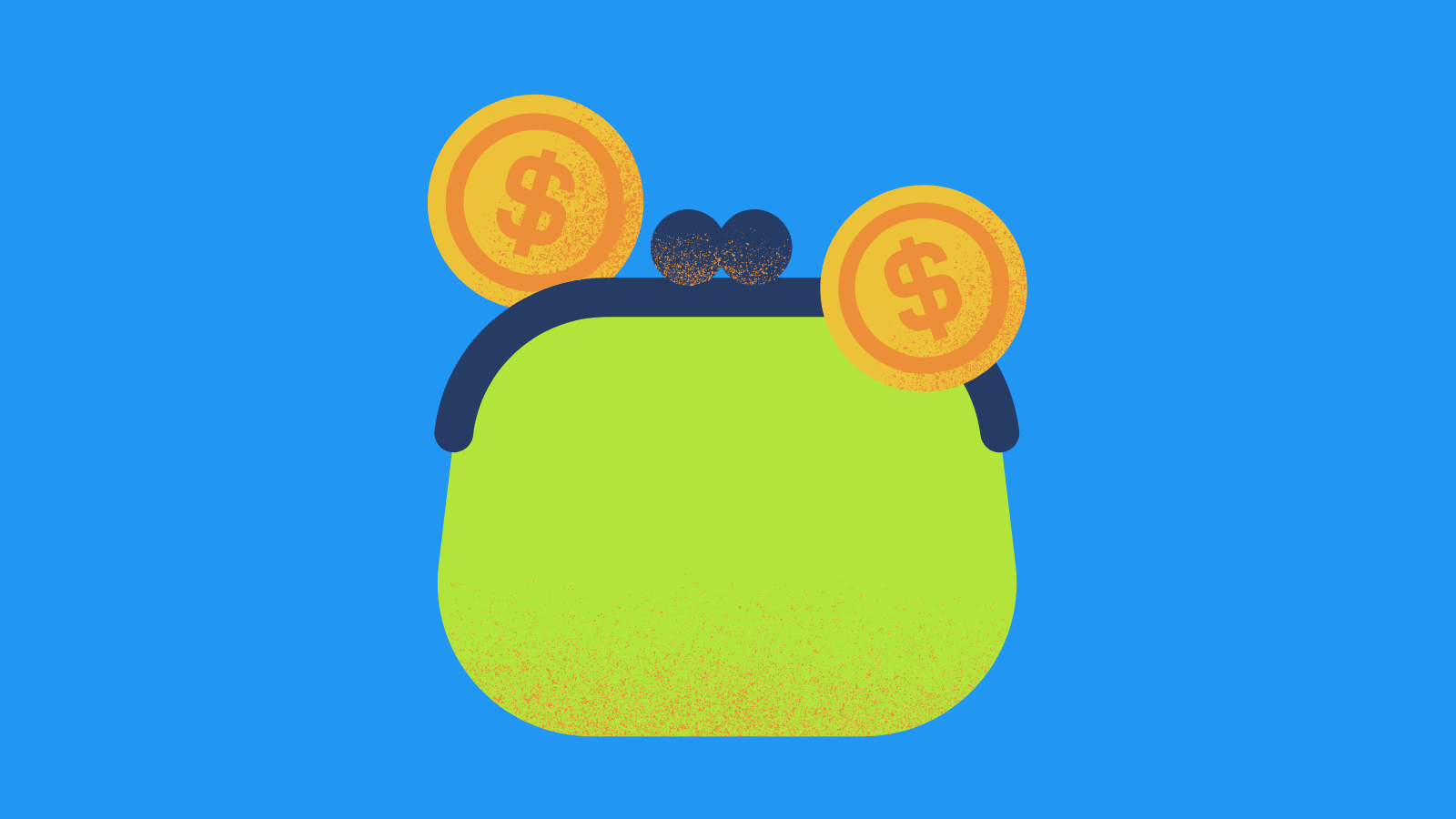
GLNG Stock Price Prediction Model
This model for forecasting Golar LNG Ltd. (GLNG) stock performance leverages a combination of time series analysis and machine learning techniques. A comprehensive dataset of historical financial data, including GLNG's stock performance, macroeconomic indicators (e.g., GDP growth, interest rates, global LNG demand), and industry-specific factors (e.g., LNG pricing, production costs), will be meticulously collected and pre-processed. Crucially, this data will be enriched with fundamental company data, including earnings reports, dividend payouts, capital expenditure plans, and announcements. Feature engineering will be paramount, transforming raw data into meaningful features, such as moving averages, volatility indicators, and ratios, allowing the model to identify patterns and trends. The dataset will be partitioned into training and testing sets to evaluate model performance rigorously. Data cleaning and handling of missing values are critical to avoid biased model predictions. The initial phase involves exploratory data analysis (EDA) to discern key trends and potential correlations among the variables.
A hybrid approach, combining linear regression and recurrent neural networks (RNNs), will be employed. Linear regression models will capture the linear relationships between historical data and stock price, while RNNs will capture intricate temporal dependencies and patterns within the data, critical for forecasting short and long-term trends. The model will be trained on the historical data to establish a relationship between independent variables and the GLNG stock price. Model validation will be performed using appropriate metrics like Mean Squared Error (MSE) and R-squared to assess predictive accuracy. The selection of the most effective model will be based on a comparative analysis of various machine learning algorithms (including support vector machines and gradient boosting), meticulously evaluating the predictive accuracy, computational efficiency, and interpretability of each model. Crucially, the model will be regularly updated with new data, ensuring its predictive capabilities remain robust. Regular backtesting will be paramount to assess the model's ability to generate consistent profitable forecasts over various time horizons.
The model will provide insightful predictions, enabling both short-term tactical trading decisions and long-term strategic investment planning. Risk assessment, incorporating volatility and uncertainty, will be incorporated into the model's output, helping stakeholders navigate potential market fluctuations. The model's output will also include probability distributions for various possible outcomes, providing a probabilistic assessment of future price trajectories. Thorough sensitivity analysis will quantify the influence of different input variables on the predicted stock price, highlighting significant factors driving GLNG's performance. The model will be presented to stakeholders with clear and concise visualizations that effectively communicate the prediction and associated risk factors. Continuous monitoring and refinement of the model's parameters and data sources will ensure its ongoing effectiveness and adaptability in evolving market conditions.
ML Model Testing
n:Time series to forecast
p:Price signals of Golar stock
j:Nash equilibria (Neural Network)
k:Dominated move of Golar stock holders
a:Best response for Golar target price
For further technical information as per how our model work we invite you to visit the article below:
How do KappaSignal algorithms actually work?
Golar Stock Forecast (Buy or Sell) Strategic Interaction Table
Strategic Interaction Table Legend:
X axis: *Likelihood% (The higher the percentage value, the more likely the event will occur.)
Y axis: *Potential Impact% (The higher the percentage value, the more likely the price will deviate.)
Z axis (Grey to Black): *Technical Analysis%
Rating | Short-Term | Long-Term Senior |
---|---|---|
Outlook | B2 | B1 |
Income Statement | B3 | B2 |
Balance Sheet | Caa2 | B2 |
Leverage Ratios | Baa2 | Baa2 |
Cash Flow | Ba2 | B2 |
Rates of Return and Profitability | Caa2 | B3 |
*Financial analysis is the process of evaluating a company's financial performance and position by neural network. It involves reviewing the company's financial statements, including the balance sheet, income statement, and cash flow statement, as well as other financial reports and documents.
How does neural network examine financial reports and understand financial state of the company?
References
- G. Theocharous and A. Hallak. Lifetime value marketing using reinforcement learning. RLDM 2013, page 19, 2013
- S. Devlin, L. Yliniemi, D. Kudenko, and K. Tumer. Potential-based difference rewards for multiagent reinforcement learning. In Proceedings of the Thirteenth International Joint Conference on Autonomous Agents and Multiagent Systems, May 2014
- Hartigan JA, Wong MA. 1979. Algorithm as 136: a k-means clustering algorithm. J. R. Stat. Soc. Ser. C 28:100–8
- Thompson WR. 1933. On the likelihood that one unknown probability exceeds another in view of the evidence of two samples. Biometrika 25:285–94
- G. Theocharous and A. Hallak. Lifetime value marketing using reinforcement learning. RLDM 2013, page 19, 2013
- N. B ̈auerle and A. Mundt. Dynamic mean-risk optimization in a binomial model. Mathematical Methods of Operations Research, 70(2):219–239, 2009.
- Belloni A, Chernozhukov V, Hansen C. 2014. High-dimensional methods and inference on structural and treatment effects. J. Econ. Perspect. 28:29–50