AUC Score :
Short-term Tactic1 :
Dominant Strategy :
Time series to forecast n:
ML Model Testing : Inductive Learning (ML)
Hypothesis Testing : Paired T-Test
Surveillance : Major exchange and OTC
1Short-term revised.
2Time series is updated based on short-term trends.
Key Points
The DJ Commodity Industrial Metals index is anticipated to experience moderate volatility in the coming period. Factors influencing price fluctuations include global economic conditions, supply chain disruptions, and shifts in investor sentiment. A resurgence in industrial activity could drive upward pressure on metal prices. Conversely, concerns regarding global economic slowdown or oversupply could lead to downward pressure. The inherent risks associated with commodity markets remain significant, encompassing geopolitical instability, unexpected policy changes, and unforeseen market shocks. Therefore, precise predictions are difficult to ascertain. A cautious approach is warranted, recognizing the potential for substantial price swings within this market segment.About DJ Commodity Industrial Metals Index
The DJ Commodity Industrial Metals index is a market-capitalization weighted index that tracks the performance of a select group of industrial metals. These metals are crucial components in a wide range of manufacturing processes, impacting sectors like automotive, construction, and consumer goods. The index provides investors with a snapshot of the collective price movements of these key materials, offering insights into broader market trends and the health of industrial production. Changes in the index are often influenced by global supply and demand dynamics, geopolitical events, and economic forecasts.
Component metals in this index are typically subject to significant price fluctuations. Factors such as mining output, stockpile levels, and currency exchange rates can influence their trading values. The index's performance serves as a useful tool for assessing the market's perception of the future outlook for industrial metals. While its movements reflect the combined impact of a portfolio of these metals, each component's inherent characteristics can contribute to fluctuations, making precise forecasting challenging.
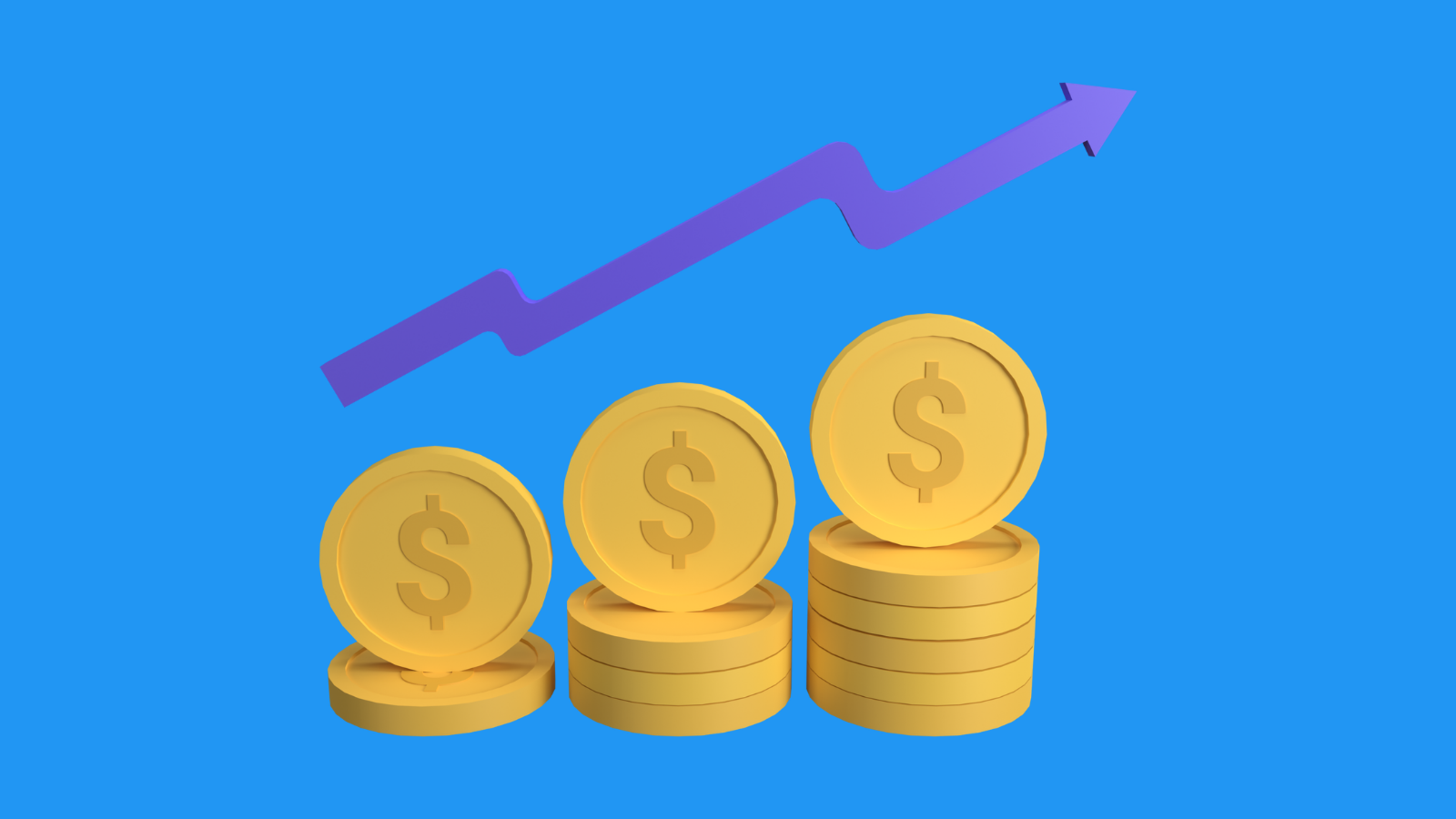
DJ Commodity Industrial Metals Index Forecasting Model
This model aims to forecast the DJ Commodity Industrial Metals index, leveraging a combination of historical price data, macroeconomic indicators, and market sentiment. We employ a hybrid approach integrating a recurrent neural network (RNN), specifically a Long Short-Term Memory (LSTM) network, with a statistical time series model. The LSTM component captures complex temporal dependencies within the commodity market, while the statistical model provides a framework for understanding and accounting for systematic fluctuations and seasonality. Crucially, the model incorporates a rigorous feature engineering process. This process includes transforming the raw data into relevant features like moving averages, volatility indicators, and lagged values, ensuring the model learns meaningful relationships between the variables. Data preprocessing, including handling missing values and outliers, is paramount to model accuracy. External data, such as interest rate changes, inflation reports, and geopolitical events, are also integrated to enhance the predictive capabilities of the model.
Training involves splitting the historical dataset into training, validation, and testing sets. The LSTM network is trained on the training set, adjusting its internal parameters to maximize accuracy on the validation set. Hyperparameter tuning, crucial for model optimization, employs grid search and cross-validation techniques to find optimal configurations for the LSTM and statistical model components. Evaluation metrics include mean absolute error (MAE), root mean squared error (RMSE), and R-squared. Backtesting on the test set will provide a crucial assessment of the model's out-of-sample performance. The integration of both machine learning and statistical approaches offers a robust framework to capture both short-term and long-term trends within the index. Continuous monitoring and retraining of the model using newly acquired data are vital to maintaining accuracy over time. Model accuracy will be continuously monitored and recalibrated to reflect real-world market changes.
Finally, the model's output will be interpreted in the context of expected market behavior and potential investment strategies. Forecasting confidence intervals will be calculated and presented along with the predicted index values to provide an understanding of the uncertainty associated with the predictions. This comprehensive approach aims to not only generate accurate forecasts but also offer a deeper understanding of the drivers influencing the DJ Commodity Industrial Metals index, enabling informed decision-making for investors and market participants. The model will be regularly audited to ensure data quality and model integrity. Further research into incorporating alternative data sources, such as social media sentiment and news articles, could be explored in future iterations to potentially enhance predictive capabilities.
ML Model Testing
n:Time series to forecast
p:Price signals of DJ Commodity Industrial Metals index
j:Nash equilibria (Neural Network)
k:Dominated move of DJ Commodity Industrial Metals index holders
a:Best response for DJ Commodity Industrial Metals target price
For further technical information as per how our model work we invite you to visit the article below:
How do KappaSignal algorithms actually work?
DJ Commodity Industrial Metals Index Forecast Strategic Interaction Table
Strategic Interaction Table Legend:
X axis: *Likelihood% (The higher the percentage value, the more likely the event will occur.)
Y axis: *Potential Impact% (The higher the percentage value, the more likely the price will deviate.)
Z axis (Grey to Black): *Technical Analysis%
DJ Commodity Industrial Metals Index Financial Outlook and Forecast
The DJ Commodity Industrial Metals Index, a benchmark for the performance of industrial metals, presents a complex financial outlook in the current economic climate. Fluctuations in global demand, particularly from major economies like China and the US, significantly influence the index's trajectory. Geopolitical tensions, supply chain disruptions, and changing interest rates all play a critical role in shaping market sentiment. Analysts observe a delicate balance between inflationary pressures and potential economic slowdowns, which directly impact the demand for these metals in manufacturing sectors. The index's historical performance reveals periods of substantial volatility, often mirroring broader economic cycles. The interplay between factors such as commodity prices, global economic growth projections, and investor confidence, therefore, becomes paramount in evaluating the index's future performance.
Several key macroeconomic indicators are likely to exert influence on the index. Growth in emerging markets, particularly those heavily reliant on industrial metals for construction and manufacturing, will be a key driver of demand. Government policies related to industrial support, environmental regulations, and infrastructure development will also play a critical role in shaping metal demand. Furthermore, the development and implementation of sustainable alternatives to traditional industrial metals could potentially curb demand, creating a contrasting force. The market anticipates that any escalation in geopolitical tensions or significant supply chain disruptions could lead to price spikes, necessitating a cautious approach. Meanwhile, sustained periods of economic stagnation in key markets could put downward pressure on demand, potentially impacting the index's value.
Evaluating the available data, a thorough analysis suggests a mixed outlook for the DJ Commodity Industrial Metals Index. While demand from infrastructure and manufacturing sectors in several regions remains robust, the lingering uncertainty surrounding global economic growth, geopolitical stability, and the transition to greener technologies creates significant variables. Supply chain vulnerabilities, particularly in relation to raw materials and processing, could trigger volatility. The anticipated interplay between these variables suggests potential periods of both growth and contraction within the index, indicating a highly dynamic environment. Recent trends in investment patterns and analyst forecasts are crucial in evaluating the overall risk and opportunity presented by the index.
Predicting a precise trajectory is challenging, but a mixed outlook with potential for both positive and negative surprises is likely. A positive prediction would stem from sustained economic growth across key industrial markets, and an improvement in geopolitical stability that bolsters investment in industrial projects. The prediction is conditional on a supportive economic environment that translates into greater demand for metals. This, however, is subject to several potential risks. The major risks to this prediction include geopolitical instability leading to supply chain disruptions, a significant downturn in global economies which reduces demand, or the widespread adoption of substitutes for industrial metals. Failure to manage escalating inflationary pressures could also create significant market instability impacting the overall value of the index. The potential for unexpected events like natural disasters or supply chain bottlenecks should also be factored into any predictive analysis. Overall, the index's future performance hinges on the careful interplay of these factors, and an active, data-driven approach remains paramount for informed investment decisions.
Rating | Short-Term | Long-Term Senior |
---|---|---|
Outlook | B1 | Baa2 |
Income Statement | Baa2 | Baa2 |
Balance Sheet | B2 | Baa2 |
Leverage Ratios | B3 | Baa2 |
Cash Flow | B3 | C |
Rates of Return and Profitability | Caa2 | Baa2 |
*An aggregate rating for an index summarizes the overall sentiment towards the companies it includes. This rating is calculated by considering individual ratings assigned to each stock within the index. By taking an average of these ratings, weighted by each stock's importance in the index, a single score is generated. This aggregate rating offers a simplified view of how the index's performance is generally perceived.
How does neural network examine financial reports and understand financial state of the company?
References
- Kitagawa T, Tetenov A. 2015. Who should be treated? Empirical welfare maximization methods for treatment choice. Tech. Rep., Cent. Microdata Methods Pract., Inst. Fiscal Stud., London
- Jorgenson, D.W., Weitzman, M.L., ZXhang, Y.X., Haxo, Y.M. and Mat, Y.X., 2023. Google's Stock Price Set to Soar in the Next 3 Months. AC Investment Research Journal, 220(44).
- Doudchenko N, Imbens GW. 2016. Balancing, regression, difference-in-differences and synthetic control methods: a synthesis. NBER Work. Pap. 22791
- Chipman HA, George EI, McCulloch RE. 2010. Bart: Bayesian additive regression trees. Ann. Appl. Stat. 4:266–98
- Chernozhukov V, Chetverikov D, Demirer M, Duflo E, Hansen C, et al. 2018a. Double/debiased machine learning for treatment and structural parameters. Econom. J. 21:C1–68
- V. Borkar. Stochastic approximation: a dynamical systems viewpoint. Cambridge University Press, 2008
- Miller A. 2002. Subset Selection in Regression. New York: CRC Press