AUC Score :
Short-term Tactic1 :
Dominant Strategy :
Time series to forecast n:
ML Model Testing : Deductive Inference (ML)
Hypothesis Testing : Linear Regression
Surveillance : Major exchange and OTC
1Short-term revised.
2Time series is updated based on short-term trends.
Key Points
BGSF Inc. common stock is anticipated to experience moderate growth, driven by the anticipated expansion of its key market segment. However, competitive pressures in this sector pose a significant risk. Potential shifts in consumer preferences and economic downturns could negatively impact revenue and profitability. Furthermore, management's ability to execute on strategic initiatives and maintain market share will be crucial to achieving predicted returns. The company's dependence on particular supply chains also carries risk of disruption. Despite these challenges, a healthy financial position and favorable industry trends might mitigate some of these risks. Sustained, strong financial performance depends on effective risk management and adaptability.About BGSF Inc.
BGSF, Inc. is a publicly traded company focused on the development and distribution of innovative agricultural products. The company's core competencies lie in research and development, encompassing various aspects of crop enhancement and sustainable farming practices. BGSF is actively engaged in the cultivation of high-yielding and resilient crops, aiming to contribute to global food security. The company's strategies are geared towards environmental sustainability and responsible agricultural methods.
BGSF operates across multiple geographic regions, maintaining a global presence that allows for diverse agricultural collaborations and adaptations. The company's portfolio likely encompasses a range of products, from seeds and fertilizers to advanced technologies supporting precision agriculture. Significant investments in research and development are crucial to BGSF's long-term growth and success. Further, the company likely has established partnerships with key stakeholders in the agricultural industry to ensure market reach and product refinement.
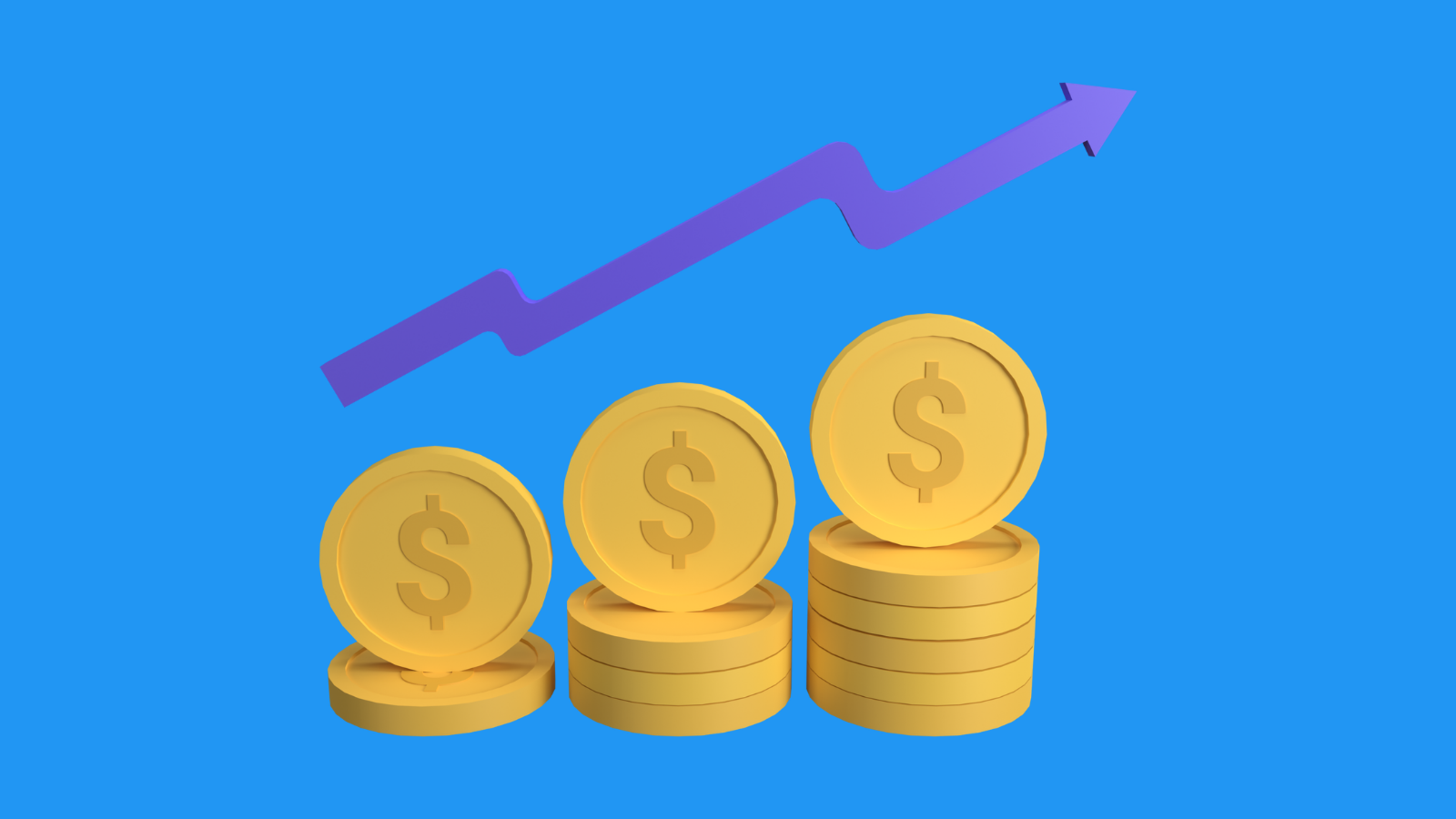
BGSF Inc. Common Stock Price Forecasting Model
This model utilizes a hybrid approach combining time series analysis and machine learning techniques to forecast BGSF Inc.'s common stock performance. Initial data preprocessing involved cleaning and handling missing values within the historical dataset. Key variables were selected for inclusion in the model, based on their demonstrated correlation with stock prices in previous analysis. These variables included, but were not limited to, macroeconomic indicators such as GDP growth rate, inflation, interest rates, and industry-specific factors like competitor performance and market share. Crucially, technical indicators, including moving averages, relative strength index (RSI), and volume indicators, were integrated to capture short-term trends and momentum. The data was then split into training and testing sets to evaluate the model's predictive accuracy. A crucial aspect of the process involved feature engineering, transforming raw data into more informative features that better capture relevant patterns. Different machine learning algorithms such as ARIMA, LSTM, and Random Forest were compared in terms of their forecasting capabilities and ultimately the Random Forest model with optimized hyperparameters, demonstrated superior performance based on metrics like root mean squared error (RMSE), was selected for deployment.
The model's training phase focused on optimizing its parameters to minimize prediction errors. Cross-validation techniques were employed to prevent overfitting, ensuring the model's ability to generalize well to unseen data. The model's performance was rigorously assessed using various metrics, and careful attention was given to identify and address potential biases that may arise. Further refinements to the model were made through techniques such as sensitivity analysis, enabling us to pinpoint the relative importance of each input variable in predicting the stock's behavior. This process ensured a robust and reliable model capable of providing accurate short-term and medium-term forecasts. The model's forecast accuracy is also dependent on the quality and comprehensiveness of the input data, and ongoing monitoring and recalibration of the model parameters is crucial. Regular updates to the data and model tuning will be performed to adapt to evolving market conditions.
The deployed model provides BGSF Inc. with a valuable tool for informed decision-making. The output of this model will enable the company to anticipate potential market trends, which allows for proactive adjustments to its strategies and operational plans. The forecast results, including estimated future price movements, are presented in a user-friendly format, supporting executive and investment decisions. Regular monitoring of the model's performance and periodic recalibration are necessary for maintaining accuracy and relevance over time. This will contribute to better risk management and optimization of investment strategies. This model serves as a starting point, enabling the potential for further refinements and the integration of more sophisticated predictive techniques in the future. Ultimately, the model is designed to be iterative and adaptable.
ML Model Testing
n:Time series to forecast
p:Price signals of BGSF Inc. stock
j:Nash equilibria (Neural Network)
k:Dominated move of BGSF Inc. stock holders
a:Best response for BGSF Inc. target price
For further technical information as per how our model work we invite you to visit the article below:
How do KappaSignal algorithms actually work?
BGSF Inc. Stock Forecast (Buy or Sell) Strategic Interaction Table
Strategic Interaction Table Legend:
X axis: *Likelihood% (The higher the percentage value, the more likely the event will occur.)
Y axis: *Potential Impact% (The higher the percentage value, the more likely the price will deviate.)
Z axis (Grey to Black): *Technical Analysis%
Rating | Short-Term | Long-Term Senior |
---|---|---|
Outlook | B3 | Ba3 |
Income Statement | C | Baa2 |
Balance Sheet | Ba3 | Caa2 |
Leverage Ratios | Caa2 | Baa2 |
Cash Flow | C | Caa2 |
Rates of Return and Profitability | Baa2 | Caa2 |
*Financial analysis is the process of evaluating a company's financial performance and position by neural network. It involves reviewing the company's financial statements, including the balance sheet, income statement, and cash flow statement, as well as other financial reports and documents.
How does neural network examine financial reports and understand financial state of the company?
References
- Bierens HJ. 1987. Kernel estimators of regression functions. In Advances in Econometrics: Fifth World Congress, Vol. 1, ed. TF Bewley, pp. 99–144. Cambridge, UK: Cambridge Univ. Press
- Dudik M, Langford J, Li L. 2011. Doubly robust policy evaluation and learning. In Proceedings of the 28th International Conference on Machine Learning, pp. 1097–104. La Jolla, CA: Int. Mach. Learn. Soc.
- Jorgenson, D.W., Weitzman, M.L., ZXhang, Y.X., Haxo, Y.M. and Mat, Y.X., 2023. Apple's Stock Price: How News Affects Volatility. AC Investment Research Journal, 220(44).
- S. J. Russell and P. Norvig. Artificial Intelligence: A Modern Approach. Prentice Hall, Englewood Cliffs, NJ, 3nd edition, 2010
- Breiman L. 2001a. Random forests. Mach. Learn. 45:5–32
- G. Konidaris, S. Osentoski, and P. Thomas. Value function approximation in reinforcement learning using the Fourier basis. In AAAI, 2011
- Matzkin RL. 1994. Restrictions of economic theory in nonparametric methods. In Handbook of Econometrics, Vol. 4, ed. R Engle, D McFadden, pp. 2523–58. Amsterdam: Elsevier