AUC Score :
Short-term Tactic1 :
Dominant Strategy :
Time series to forecast n:
ML Model Testing : Modular Neural Network (News Feed Sentiment Analysis)
Hypothesis Testing : Paired T-Test
Surveillance : Major exchange and OTC
1Short-term revised.
2Time series is updated based on short-term trends.
Key Points
The DJ Commodity Industrial Metals index is anticipated to experience moderate volatility in the coming period. Several factors suggest a potential upward trend, including increased global industrial activity and robust demand for raw materials. However, price fluctuations are likely to be influenced by macroeconomic uncertainties, such as geopolitical tensions, supply chain disruptions, and potential shifts in interest rates. A key risk is the potential for unexpected supply shocks, which could drive prices higher than anticipated. Conversely, overproduction or a sudden decline in demand could depress prices, introducing another significant risk. Overall, while a positive outlook is possible, the index's trajectory will remain contingent on various external factors, requiring careful monitoring and ongoing analysis.About DJ Commodity Industrial Metals Index
The DJ Commodity Industrial Metals Index is a benchmark that tracks the performance of a diversified portfolio of industrial metals. It encompasses a range of crucial metals vital for manufacturing, construction, and various industrial applications. This index provides a key indicator of market sentiment and price trends for these metals, often used as a gauge of broader economic activity and industrial demand. The constituents of the index represent a selection of important metallic elements, reflecting their significance across various sectors.
The index's composition and methodologies are designed to give investors and analysts a comprehensive view of the industrial metals market. The constituents and weighting schemes are adjusted periodically to maintain relevance and reflect evolving market dynamics and supply chain factors. Fluctuations in the index are frequently tied to factors such as global supply and demand, geopolitical events, and investor sentiment regarding the future of industrial production and consumption.
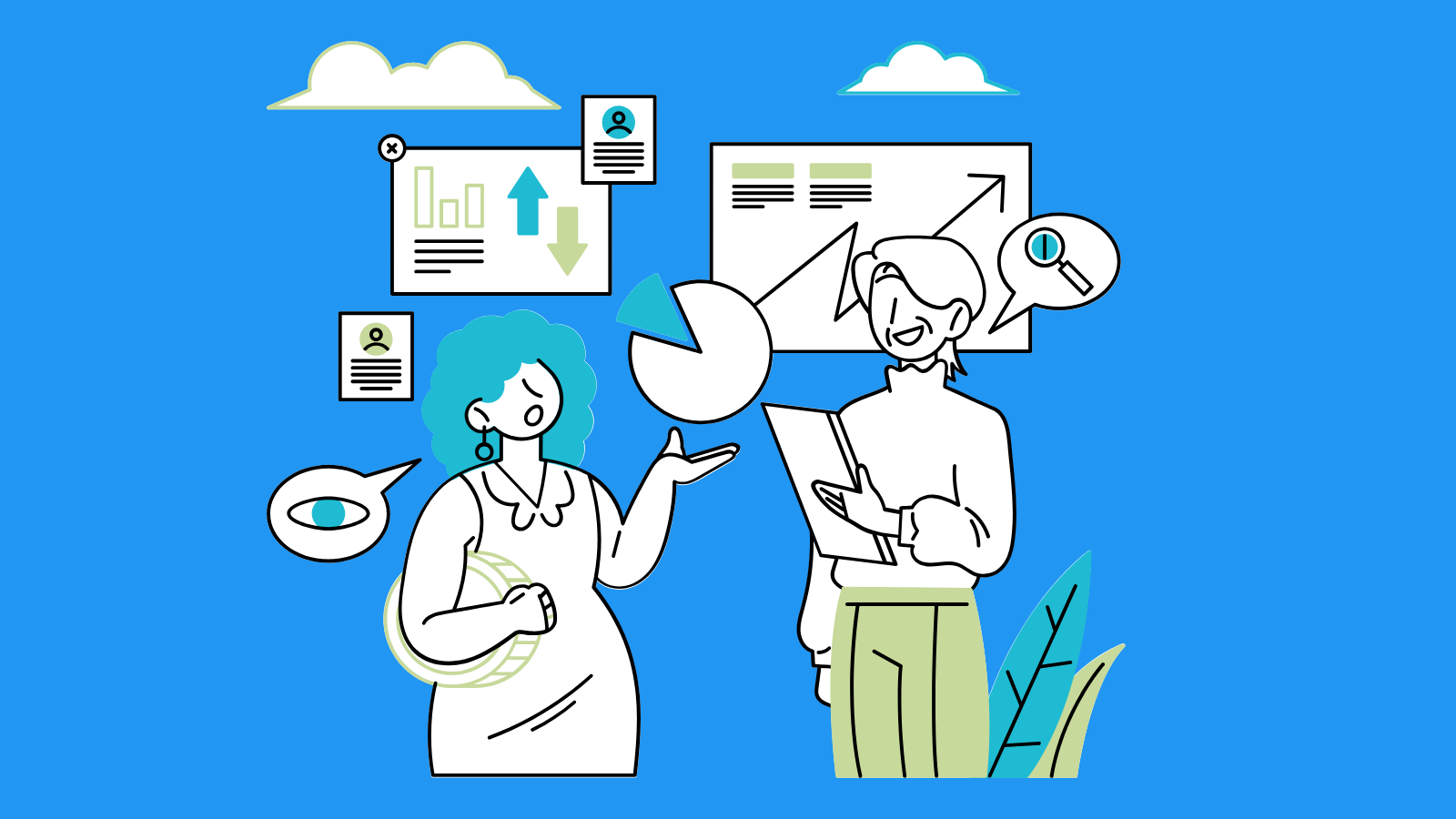
DJ Commodity Industrial Metals Index Forecasting Model
This model utilizes a hybrid approach combining time series analysis and machine learning techniques to forecast the DJ Commodity Industrial Metals index. Initial data preprocessing involves cleaning the dataset, handling missing values, and potentially transforming features to ensure data quality and suitability for modeling. A crucial step is feature engineering, where we derive new variables from existing ones, such as lagged values, moving averages, and seasonality indicators. These engineered features aim to capture trends, seasonality, and other complex patterns potentially influencing the index. Furthermore, external economic indicators like inflation rates, interest rates, and global GDP growth are incorporated as additional features, enriching the model's understanding of the broader economic context impacting the metals market. Data from historical commodity prices, global macroeconomic indicators, and geopolitical events are crucial for the model's training and evaluation. Initial experimentation will explore various time series models like ARIMA, SARIMA, and exponential smoothing to establish a baseline for prediction performance.
The machine learning component leverages ensemble methods, specifically gradient boosting, to improve predictive accuracy. Gradient boosting algorithms, like XGBoost or LightGBM, excel at handling complex relationships and non-linear patterns within the data. These algorithms iteratively learn from mistakes made by previous models and combine multiple simpler models into a more powerful one. Hyperparameter tuning is essential to optimize the model's performance through techniques like grid search or random search. Evaluation metrics like root mean squared error (RMSE) and mean absolute error (MAE) will be used to quantify the predictive ability of different models and compare their performance across various time horizons. Model selection and validation techniques, such as time series cross-validation, will be critical to ensure the model generalizes effectively to unseen data and avoids overfitting to the training set. Careful consideration of model interpretability will be applied to understand the influence of different factors on the forecasted commodity prices.
The final model will be a robust and comprehensive forecasting tool capable of incorporating both the inherent time series patterns and external economic influences. It will be regularly updated and re-evaluated to maintain its predictive accuracy and adapt to evolving market conditions. Continuous monitoring of market trends and economic data will be essential to identifying shifts in the underlying relationships that drive future price movements. Rigorous backtesting on historical data will be performed to assess the model's reliability and establish confidence intervals around the predicted values. The model will be deployed for practical applications after demonstrating adequate performance and robustness on various test datasets, with a careful analysis of the assumptions and limitations of the model and predictions. Deployment will include regular monitoring and updating of the model with new data, ensuring it retains optimal predictive power.
ML Model Testing
n:Time series to forecast
p:Price signals of DJ Commodity Industrial Metals index
j:Nash equilibria (Neural Network)
k:Dominated move of DJ Commodity Industrial Metals index holders
a:Best response for DJ Commodity Industrial Metals target price
For further technical information as per how our model work we invite you to visit the article below:
How do KappaSignal algorithms actually work?
DJ Commodity Industrial Metals Index Forecast Strategic Interaction Table
Strategic Interaction Table Legend:
X axis: *Likelihood% (The higher the percentage value, the more likely the event will occur.)
Y axis: *Potential Impact% (The higher the percentage value, the more likely the price will deviate.)
Z axis (Grey to Black): *Technical Analysis%
DJ Commodity Industrial Metals Index Financial Outlook and Forecast
The DJ Commodity Industrial Metals Index, a benchmark for the performance of industrial metal commodities, presents a complex financial outlook. Current market conditions are influenced by a multitude of interconnected factors. Global economic growth projections, alongside anticipated changes in manufacturing activity and industrial production, play a pivotal role. A robust global economy, characterized by increased demand for industrial metals used in manufacturing processes, typically correlates with higher index values. Conversely, a weakening economic outlook often leads to decreased demand and lower index valuations. The ongoing geopolitical landscape, with its inherent uncertainties, also exerts a substantial influence. Trade tensions, political instability, or conflicts in key producing regions can significantly impact supply chains, commodity prices, and consequently, the index's performance. Furthermore, fluctuations in raw material prices and input costs affect the profitability of various industrial sectors, indirectly impacting the demand for industrial metals.
Supply chain disruptions and the evolving energy landscape are critical considerations. The increasing global reliance on industrial metals, particularly for renewable energy technologies, points to a potential long-term upward trend. However, supply chain inefficiencies, resulting from logistics bottlenecks, labor shortages, or raw material sourcing issues, can lead to price volatility and uncertainties. The transition to a low-carbon economy, with its growing demand for metals used in electric vehicles, solar panels, and wind turbines, may further increase demand in the long run. Simultaneously, inflationary pressures, and the resultant impact on interest rates, also exert a significant influence on market sentiment and commodity prices. The interaction of these factors shapes the index's trajectory and necessitates a nuanced understanding of the overall economic climate to form any reliable projection.
Analyzing historical trends reveals periods of significant volatility within the industrial metals market. Factors like fluctuating raw material costs, shifts in global manufacturing activity, and changes in geopolitical tensions have frequently influenced the index's performance. Therefore, any predictive model must incorporate an understanding of these historical dynamics. The current economic environment, however, introduces an added layer of complexity with elements such as inflation and rising interest rates. These trends affect investment strategies and the overall outlook for the index, rendering precise forecasts challenging. It is essential to assess the interplay between these factors, recognizing their dynamic interaction as a critical element in comprehending the market's direction.
Predicting the future trajectory of the DJ Commodity Industrial Metals Index necessitates careful consideration of potential outcomes. A positive prediction anticipates continued robust global economic growth, leading to a surge in industrial production and metal demand. Favorable geopolitical conditions, stable energy markets, and efficient supply chains would further bolster this positive outlook. However, the prediction carries risks such as sharp economic downturns, escalating geopolitical tensions, significant supply chain disruptions, and unforeseen inflation or interest rate hikes. These risks could lead to a downturn in the index and necessitate a cautious investment approach. Alternatively, a negative prediction anticipates reduced global economic activity, which can severely curtail industrial metal demand. Geopolitical tensions, supply chain disruptions, and energy price spikes can intensify this negative scenario. Therefore, investors must carefully assess these potential risks and uncertainties before making any investment decisions concerning the index.
Rating | Short-Term | Long-Term Senior |
---|---|---|
Outlook | Ba3 | B2 |
Income Statement | Ba2 | B2 |
Balance Sheet | C | Ba3 |
Leverage Ratios | B1 | B3 |
Cash Flow | Baa2 | Caa2 |
Rates of Return and Profitability | Baa2 | Caa2 |
*An aggregate rating for an index summarizes the overall sentiment towards the companies it includes. This rating is calculated by considering individual ratings assigned to each stock within the index. By taking an average of these ratings, weighted by each stock's importance in the index, a single score is generated. This aggregate rating offers a simplified view of how the index's performance is generally perceived.
How does neural network examine financial reports and understand financial state of the company?
References
- Imai K, Ratkovic M. 2013. Estimating treatment effect heterogeneity in randomized program evaluation. Ann. Appl. Stat. 7:443–70
- L. Prashanth and M. Ghavamzadeh. Actor-critic algorithms for risk-sensitive MDPs. In Proceedings of Advances in Neural Information Processing Systems 26, pages 252–260, 2013.
- Breusch, T. S. (1978), "Testing for autocorrelation in dynamic linear models," Australian Economic Papers, 17, 334–355.
- Mikolov T, Chen K, Corrado GS, Dean J. 2013a. Efficient estimation of word representations in vector space. arXiv:1301.3781 [cs.CL]
- Bera, A. M. L. Higgins (1997), "ARCH and bilinearity as competing models for nonlinear dependence," Journal of Business Economic Statistics, 15, 43–50.
- A. Shapiro, W. Tekaya, J. da Costa, and M. Soares. Risk neutral and risk averse stochastic dual dynamic programming method. European journal of operational research, 224(2):375–391, 2013
- Mnih A, Hinton GE. 2007. Three new graphical models for statistical language modelling. In International Conference on Machine Learning, pp. 641–48. La Jolla, CA: Int. Mach. Learn. Soc.