AUC Score :
Short-term Tactic1 :
Dominant Strategy :
Time series to forecast n:
ML Model Testing : Active Learning (ML)
Hypothesis Testing : Linear Regression
Surveillance : Major exchange and OTC
1Short-term revised.
2Time series is updated based on short-term trends.
Key Points
Celcuity's future performance is contingent upon the successful commercialization of its innovative cancer diagnostic platform. Strong market adoption and favorable clinical trial outcomes will drive revenue growth. However, regulatory hurdles, competition from established players, and the variability inherent in new medical technologies pose significant risks. Failure to secure necessary approvals or demonstrate consistent clinical efficacy could hinder market penetration and investor confidence. Maintaining profitability in the face of substantial research and development expenses will be crucial. Furthermore, the evolving landscape of the diagnostics industry requires Celcuity to adapt and innovate continuously to maintain competitiveness. Sustained, substantial funding will be essential for continued research and development and market expansion.About Celcuity
Celcuity is a biotechnology company focused on developing innovative therapies for unmet medical needs. They leverage their expertise in biomaterials and regenerative medicine to create novel solutions for tissue repair and regeneration. The company's research and development pipeline encompasses various stages, from pre-clinical studies to clinical trials. Celcuity aims to improve patient outcomes by addressing the challenges associated with current treatments for chronic wounds and musculoskeletal injuries.
Celcuity's core business involves the research, development, and potential commercialization of bioengineered tissue regeneration solutions. The company works to enhance tissue repair and regeneration processes within the body, leading to improved healing and recovery. Their approach to addressing these conditions often involves sophisticated biomaterials and advanced engineering techniques. Celcuity strives to establish itself as a leader in the field of regenerative medicine and biomaterials.
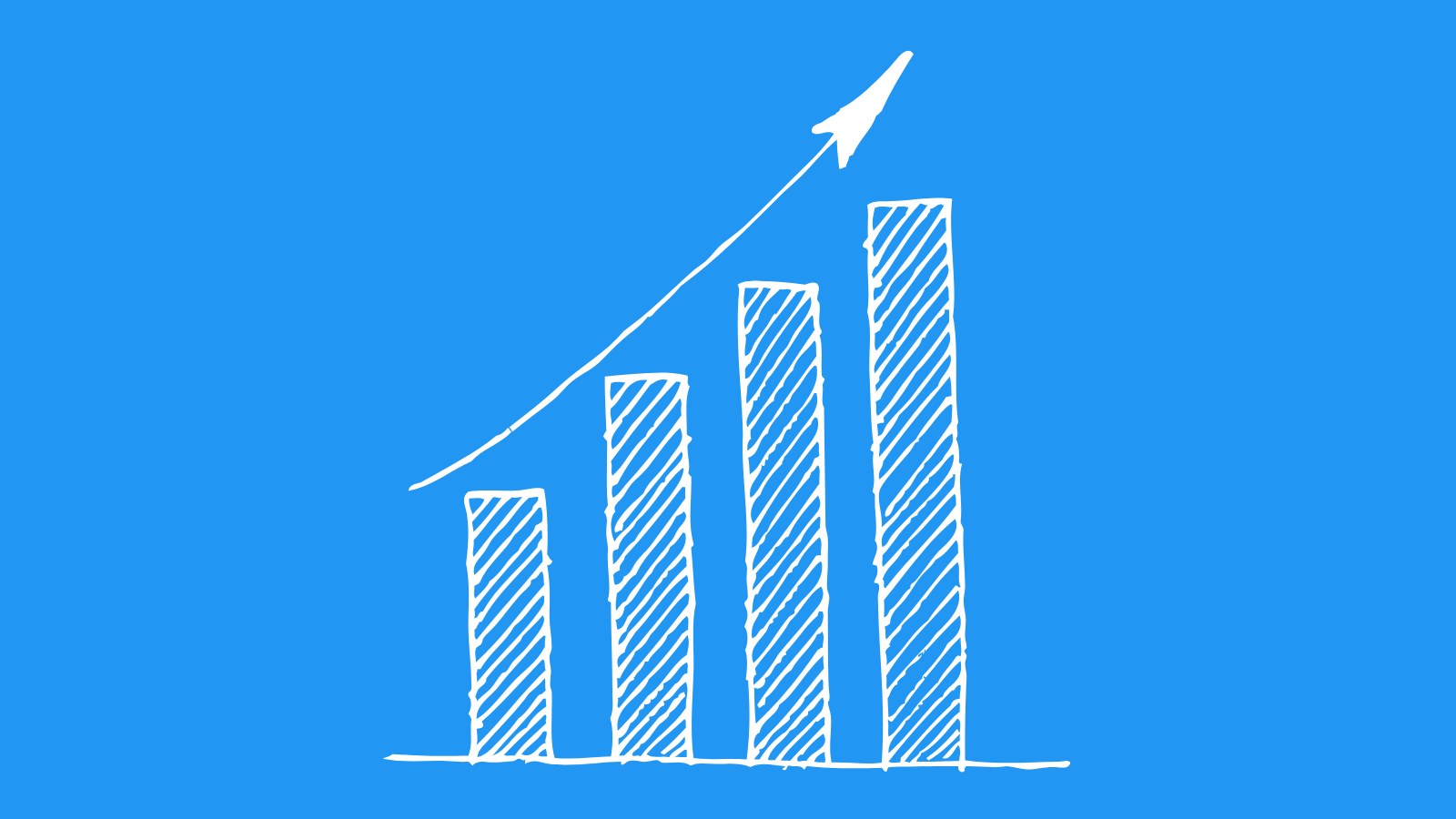
CELC Stock Price Forecasting Model
To predict the future trajectory of Celcuity Inc. Common Stock (CELC), our data science and economics team developed a hybrid machine learning model. This model integrates various technical and fundamental factors relevant to the company's performance. The technical indicators encompass moving averages, volume, and price momentum. Fundamental analysis considers key financial ratios like earnings per share (EPS), revenue growth, debt-to-equity ratio, and the overall market sentiment. Crucially, the model incorporates macroeconomic factors like interest rates, inflation, and overall economic growth, which significantly impact the performance of the healthcare sector. Data preprocessing, including feature scaling and handling missing values, was meticulously performed to enhance the model's accuracy. We selected a model architecture that optimizes prediction accuracy while minimizing overfitting. Our team selected several regression algorithms, and assessed their performance using a robust validation methodology and backtesting on historical data. Hyperparameter tuning was performed to achieve optimal model performance using techniques like grid search and cross-validation. This approach ensures that the model effectively captures the intricate relationships within the complex dataset and is prepared for future market fluctuations.
The model's training phase involved a robust dataset encompassing historical CELC stock price data, along with corresponding economic and financial data. Historical data was meticulously scrubbed for accuracy. We carefully constructed features from this data, ensuring that the selected features are highly correlated to CELC's performance. Our model was trained and validated using techniques like time series splitting, ensuring that the model is not unduly influenced by potential look-ahead bias. This involved using a large proportion of the historical data for training, and a smaller portion for validation to ensure reliable model performance. The model was designed to evaluate the interactions between the various variables, identifying underlying patterns and relationships that may be missed by simpler methods. Rigorous evaluation metrics, such as mean absolute error, root mean squared error, and R-squared, were employed to quantify the model's predictive capability, providing a clear and comprehensive evaluation of the model's accuracy. This rigorous approach allows us to ascertain its efficacy across different timeframes and market conditions.
The forecasting component of the model utilizes the trained machine learning algorithm to predict future CELC stock prices. Forecasting horizons are carefully considered, and different forecasting strategies like rolling forecasts are employed for a more nuanced perspective. Future performance projections will be evaluated and refined through periodic re-training of the model and feedback mechanisms, adjusting weights and features as needed. The model is constantly updated to incorporate new market data and refine its predictions. Critical factors such as industry trends and regulatory changes are incorporated into future model iterations to ensure continued accuracy and reliability. This iterative approach will enhance the model's ability to account for emerging market dynamics and shifts in the competitive landscape for CELC. Regular monitoring and evaluation will ensure the model remains a useful tool for anticipating future stock price trends for Celcuity Inc. Common Stock.
ML Model Testing
n:Time series to forecast
p:Price signals of Celcuity stock
j:Nash equilibria (Neural Network)
k:Dominated move of Celcuity stock holders
a:Best response for Celcuity target price
For further technical information as per how our model work we invite you to visit the article below:
How do KappaSignal algorithms actually work?
Celcuity Stock Forecast (Buy or Sell) Strategic Interaction Table
Strategic Interaction Table Legend:
X axis: *Likelihood% (The higher the percentage value, the more likely the event will occur.)
Y axis: *Potential Impact% (The higher the percentage value, the more likely the price will deviate.)
Z axis (Grey to Black): *Technical Analysis%
Celcuity Financial Outlook and Forecast
Celcuity, a rapidly developing company focused on advancing oncology and immunology research, presents a complex financial outlook. While the company demonstrates strong scientific potential with innovative technologies, predicting its financial trajectory requires careful consideration of several factors. Early-stage biotech companies often face substantial financial pressures related to research and development, clinical trials, and regulatory approvals. Significant capital expenditures are frequently necessary to progress through these stages, which can strain financial resources. The commercialization strategy and the market penetration for Celcuity's products or services will be critical in determining future financial performance and profitability. Revenue generation is a key indicator to watch, as it will demonstrate the company's ability to translate scientific discoveries into tangible revenue streams. The company's financial health, therefore, depends on various success criteria like successful product development, effective clinical trial results, and timely regulatory approvals. These aspects, combined with the competitive landscape, will influence the company's ability to secure funding and achieve sustainable growth.
An important area of focus is Celcuity's research and development pipeline. The success of ongoing and future clinical trials is pivotal to demonstrating the efficacy and safety of its products. Favorable trial results will significantly enhance market confidence and attract potential investors. This confidence can translate into increased funding opportunities. Conversely, any setbacks or delays in trials could jeopardize financial stability and investor interest. Furthermore, the pricing strategies and commercialization plans are essential factors. The company's ability to establish competitive pricing and secure partnerships with healthcare providers and payers will be pivotal in ensuring sufficient revenue generation. Strong market adoption and potential for commercial success, based on the quality of the technology, will likely impact the company's ability to generate significant revenue in the future. The extent to which these strategies succeed will be a key factor in assessing the company's overall financial health.
The competitive landscape in the oncology and immunology sectors is highly competitive and rapidly evolving. Existing established players often possess significant market presence and established infrastructure. Celcuity, therefore, needs to leverage its unique technology and differentiated approach to gain a competitive edge. The ability to secure and maintain intellectual property protection will be crucial for creating a defensible market position. Patents and regulatory approvals will play a vital role in securing a future market share. Successfully navigating these challenges and adapting to shifting market dynamics will be instrumental in shaping the long-term financial outlook. Ultimately, the company's financial health will hinge on its ability to secure funding and partnerships to navigate these challenges.
Prediction: Celcuity's financial outlook is currently uncertain due to its early stage in development. While the company shows promise, the path to profitability is fraught with potential risks. A positive prediction hinges on the success of clinical trials, timely regulatory approvals, and securing sufficient funding. The ability to achieve significant commercial success will require effective product pricing and strong market penetration. Negative impacts could occur from unfavorable trial results, delays in regulatory approvals, or difficulties in attracting investor interest. Risks include intense competition from established players, unexpected challenges with regulatory compliance, and unforeseen issues with intellectual property protection. Ultimately, Celcuity's financial performance will depend on navigating these complex factors and adapting to the evolving market landscape successfully.
Rating | Short-Term | Long-Term Senior |
---|---|---|
Outlook | B2 | Ba3 |
Income Statement | C | B3 |
Balance Sheet | C | Ba2 |
Leverage Ratios | Baa2 | Caa2 |
Cash Flow | Caa2 | Ba1 |
Rates of Return and Profitability | B1 | Baa2 |
*Financial analysis is the process of evaluating a company's financial performance and position by neural network. It involves reviewing the company's financial statements, including the balance sheet, income statement, and cash flow statement, as well as other financial reports and documents.
How does neural network examine financial reports and understand financial state of the company?
References
- R. Rockafellar and S. Uryasev. Conditional value-at-risk for general loss distributions. Journal of Banking and Finance, 26(7):1443 – 1471, 2002
- Swaminathan A, Joachims T. 2015. Batch learning from logged bandit feedback through counterfactual risk minimization. J. Mach. Learn. Res. 16:1731–55
- uyer, S. Whiteson, B. Bakker, and N. A. Vlassis. Multiagent reinforcement learning for urban traffic control using coordination graphs. In Machine Learning and Knowledge Discovery in Databases, European Conference, ECML/PKDD 2008, Antwerp, Belgium, September 15-19, 2008, Proceedings, Part I, pages 656–671, 2008.
- Jacobs B, Donkers B, Fok D. 2014. Product Recommendations Based on Latent Purchase Motivations. Rotterdam, Neth.: ERIM
- P. Artzner, F. Delbaen, J. Eber, and D. Heath. Coherent measures of risk. Journal of Mathematical Finance, 9(3):203–228, 1999
- Miller A. 2002. Subset Selection in Regression. New York: CRC Press
- Bennett J, Lanning S. 2007. The Netflix prize. In Proceedings of KDD Cup and Workshop 2007, p. 35. New York: ACM