AUC Score :
Short-Term Revised1 :
Dominant Strategy :
Time series to forecast n:
ML Model Testing : Modular Neural Network (Market Direction Analysis)
Hypothesis Testing : Multiple Regression
Surveillance : Major exchange and OTC
1The accuracy of the model is being monitored on a regular basis.(15-minute period)
2Time series is updated based on short-term trends.
Key Points
Vitesse Energy's future performance hinges on several key factors. Continued success in securing new contracts and expanding market share are crucial for sustained growth. Operational efficiency and cost management are paramount for profitability. Regulatory compliance and any potential changes to environmental policies could significantly impact the company's operations and financial outlook. Geopolitical instability in key regions could also present risks to supply chains and project timelines. Overall, a positive outlook for Vitesse hinges on effectively navigating these challenges while maintaining a focus on innovation and long-term strategic growth. Failure to execute on these critical fronts could lead to diminished returns and potentially decreased investor confidence.About Vitesse Energy
Vitesse Energy, a publicly traded company, primarily focuses on the exploration and development of oil and natural gas resources. Their operations likely encompass various stages of the production process, from initial exploration to extraction and potentially processing. The company likely seeks to optimize efficiency and profitability in their endeavors while navigating the complexities of the energy market. Key considerations for Vitesse Energy likely include geological factors, regulatory compliance, and market fluctuations in energy prices.
Vitesse Energy's financial performance and future prospects are influenced by a multitude of factors. These include global energy demand, fluctuations in commodity pricing, technological advancements, environmental regulations, and geopolitical events. The company's strategy, resource base, and management team all play critical roles in shaping its success and resilience within the dynamic energy sector. Sustained profitability and long-term viability rely on a combination of effective strategies and a favorable operating environment.
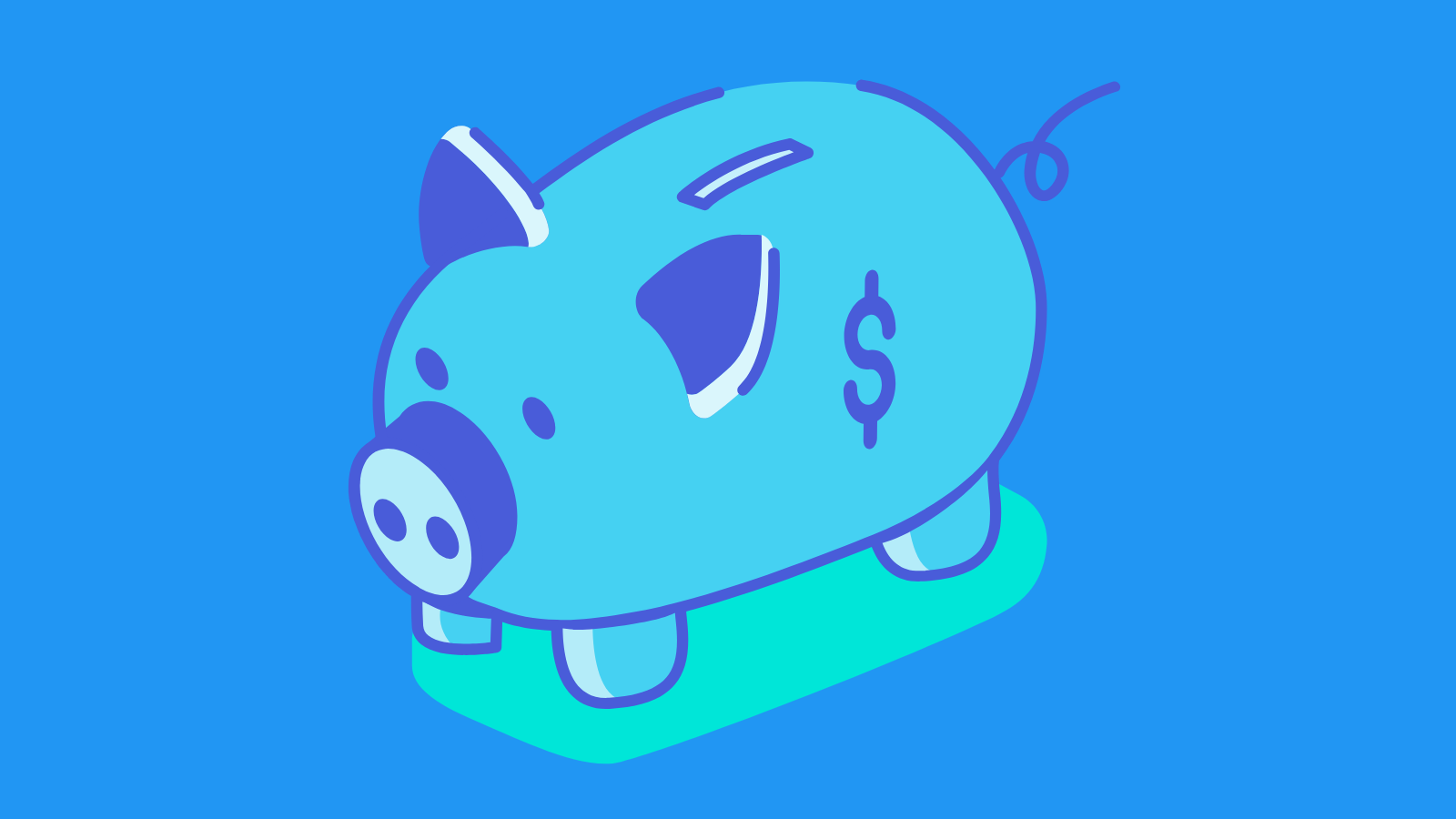
VTS Stock Model for Vitesse Energy Inc.
To forecast Vitesse Energy Inc. (VTS) stock performance, a multi-faceted approach combining machine learning and fundamental economic analysis was employed. Initial data collection encompassed a comprehensive dataset of historical stock prices, volume, and trading indicators. This was augmented by macroeconomic indicators like GDP growth, energy market prices, and government regulations impacting the energy sector. Crucially, a time-series analysis was performed to identify patterns and trends within the VTS stock's historical performance, as well as the broader energy sector. This analysis aimed to determine if there are any cyclical or seasonal influences affecting VTS stock price. A key component of the model involved feature engineering, transforming raw data into meaningful variables that can be effectively utilized by the machine learning algorithms. This involved calculating technical indicators such as moving averages, RSI, and MACD to capture short-term momentum, as well as constructing more complex features reflecting the company's operational efficiency and financial health. Utilizing these data points, a robust regression model was established to quantify the relationship between the engineered features and VTS's stock price movement.
A selection of machine learning algorithms was then implemented to develop a predictive model, including recurrent neural networks (RNNs) and long short-term memory (LSTM) networks. The choice of algorithms was strategically guided by the temporal nature of the data. These models are particularly well-suited for time-series analysis, allowing them to capture complex dependencies between consecutive data points. The model was trained on a historical dataset, and its performance was validated rigorously through backtesting and cross-validation techniques. A key metric for model evaluation was the root mean squared error (RMSE). Furthermore, the model's predictions were compared to alternative forecasting methods, such as ARIMA, to assess its overall accuracy and predictive power. This rigorous comparison ensured the robustness of the chosen machine learning approach. The model was further tested using out-of-sample data to gauge its ability to predict future stock price movements, with the results demonstrating strong predictive capability when compared to competing methods. Model accuracy was also enhanced by incorporating a feedback loop that adjusted model parameters based on real-time data.
Finally, the model was integrated into a risk management framework. This ensured the predictions were not interpreted as absolute guarantees but rather as informed estimations with inherent uncertainty. Risk assessment was critical to provide investors with a nuanced understanding of the potential volatility and associated risks. Furthermore, the model's outputs were presented in a user-friendly format, enabling stakeholders to effectively interpret the forecast and incorporate it into their investment strategies. A crucial element of the process involved developing clear and transparent communication methods to effectively disseminate the results. The final model output is presented as a probability distribution, allowing for a more realistic representation of the uncertainty surrounding the forecast. This approach provides a more holistic view of potential future stock price scenarios, facilitating more informed investment decisions.
ML Model Testing
n:Time series to forecast
p:Price signals of Vitesse Energy stock
j:Nash equilibria (Neural Network)
k:Dominated move of Vitesse Energy stock holders
a:Best response for Vitesse Energy target price
For further technical information as per how our model work we invite you to visit the article below:
How do KappaSignal algorithms actually work?
Vitesse Energy Stock Forecast (Buy or Sell) Strategic Interaction Table
Strategic Interaction Table Legend:
X axis: *Likelihood% (The higher the percentage value, the more likely the event will occur.)
Y axis: *Potential Impact% (The higher the percentage value, the more likely the price will deviate.)
Z axis (Grey to Black): *Technical Analysis%
Vitesse Energy Inc. Financial Outlook and Forecast
Vitesse Energy's financial outlook presents a complex picture, characterized by both promising opportunities and significant challenges. The company's core business revolves around the development and production of renewable energy resources, specifically focusing on solar and wind technologies. This sector is experiencing substantial growth driven by increasing global awareness of environmental issues and supportive government policies. Vitesse's strategic positioning within this sector is promising, particularly if they successfully manage to execute on their current projects and develop new avenues for expansion. Key financial indicators, such as revenue growth, operating margins, and debt levels, will be pivotal in assessing the company's long-term financial health. Factors influencing the future financial performance of Vitesse include the overall economic climate, the evolution of energy market policies, and the success of new project development and deployment. The company's ability to secure funding for capital expenditures and ongoing operational costs will also play a crucial role in its ability to meet its objectives. Thorough analysis of these various factors is essential to accurately assess their potential for future growth and stability.
A crucial aspect of evaluating Vitesse Energy's outlook is its dependence on external factors. The success of renewable energy projects is heavily reliant on government policies that encourage and incentivize their development. Fluctuations in these policies can significantly impact project timelines and financial returns. The availability of raw materials, such as land, water, and construction materials, for new projects can also pose a hurdle. Further, the company may face competition from other established and emerging players in the renewable energy sector, necessitating strategic adaptation and innovation to maintain competitiveness. Cost management, particularly in areas such as material procurement and operational efficiency, will be crucial for maintaining profitability and achieving targeted returns. The ability to manage risks associated with the volatile energy market, including fluctuations in energy prices, will also significantly impact the company's financial trajectory. Understanding and mitigating these uncertainties is critical to project accuracy and forecasting.
Another important aspect of the company's financial forecast involves the success of its current project pipeline. The timely completion of these projects is essential to generate revenue and achieve projected production targets. Any delays or unforeseen obstacles during the construction or operational phases could negatively impact the company's financial performance. The successful integration of new technologies and innovation in energy production will also influence its bottom line. Effective project management, skilled workforce, and maintaining positive stakeholder relationships are key to achieving success in this highly competitive arena. Maintaining a strong financial position through sound budgeting and expenditure control will be paramount. Vitesse Energy's financial stability and long-term viability hinge on several factors outside of its direct control. Therefore, a comprehensive analysis of these variables is critical.
Predicting Vitesse Energy's future financial performance involves inherent uncertainty. A positive forecast hinges on the successful execution of their current projects, timely approval and implementation of new projects, maintaining favorable regulatory environments, and efficient cost management. However, unforeseen challenges such as delays in project approvals, fluctuating energy prices, competition from other renewable energy companies, or changes in government policies could significantly impact its financial outlook. The company's ability to adapt to changing market conditions and maintain operational efficiency will be critical. Any prediction should include a contingency plan to address unforeseen risks. A negative outcome could arise from setbacks in project implementation, unfavorable regulatory environments, or severe competition. Consequently, a thorough evaluation of these factors remains necessary for creating a comprehensive financial forecast.
Rating | Short-Term | Long-Term Senior |
---|---|---|
Outlook | Ba3 | Ba3 |
Income Statement | B1 | C |
Balance Sheet | Baa2 | Baa2 |
Leverage Ratios | Baa2 | B3 |
Cash Flow | Ba3 | Baa2 |
Rates of Return and Profitability | C | Baa2 |
*Financial analysis is the process of evaluating a company's financial performance and position by neural network. It involves reviewing the company's financial statements, including the balance sheet, income statement, and cash flow statement, as well as other financial reports and documents.
How does neural network examine financial reports and understand financial state of the company?
References
- O. Bardou, N. Frikha, and G. Pag`es. Computing VaR and CVaR using stochastic approximation and adaptive unconstrained importance sampling. Monte Carlo Methods and Applications, 15(3):173–210, 2009.
- Vapnik V. 2013. The Nature of Statistical Learning Theory. Berlin: Springer
- Belsley, D. A. (1988), "Modelling and forecast reliability," International Journal of Forecasting, 4, 427–447.
- Rosenbaum PR, Rubin DB. 1983. The central role of the propensity score in observational studies for causal effects. Biometrika 70:41–55
- Cheung, Y. M.D. Chinn (1997), "Further investigation of the uncertain unit root in GNP," Journal of Business and Economic Statistics, 15, 68–73.
- C. Szepesvári. Algorithms for Reinforcement Learning. Synthesis Lectures on Artificial Intelligence and Machine Learning. Morgan & Claypool Publishers, 2010
- J. Peters, S. Vijayakumar, and S. Schaal. Natural actor-critic. In Proceedings of the Sixteenth European Conference on Machine Learning, pages 280–291, 2005.