AUC Score :
Short-Term Revised1 :
Dominant Strategy :
Time series to forecast n:
ML Model Testing : Deductive Inference (ML)
Hypothesis Testing : Lasso Regression
Surveillance : Major exchange and OTC
1The accuracy of the model is being monitored on a regular basis.(15-minute period)
2Time series is updated based on short-term trends.
Key Points
The TR/CC CRB Soybeans index is anticipated to experience moderate volatility in the coming period. Factors influencing this prediction include the ongoing global agricultural landscape, including weather patterns, supply chain disruptions, and shifts in international demand. Potential price increases are likely if unfavorable weather conditions impact crop yields. Conversely, overabundant supply could lead to price declines. Geopolitical uncertainties and economic fluctuations also present risks to the index's stability. There is a risk of significant price swings driven by unforeseen events. Investors should consider these potential risks and adapt their strategies accordingly.About TR/CC CRB Soybeans Index
The TR/CC CRB Soybeans index is a benchmark that tracks the price fluctuations of soybean futures contracts traded on the Chicago Board of Trade (CBOT). It serves as a key indicator of the global soybean market, reflecting supply and demand dynamics, as well as geopolitical factors. The index is widely followed by market participants, including traders, investors, and agricultural businesses, to gauge market sentiment and potential price movements. Key factors influencing the index include weather patterns impacting soybean harvests, global demand, and export policies. The index's volatility can reflect rapid changes in any of these factors.
Historically, the TR/CC CRB Soybeans index has demonstrated significant price variations throughout the year. Fluctuations are often correlated with the agricultural cycle, with price peaks typically coinciding with harvest seasons and troughs during periods of ample supply. Analysts scrutinize the index to identify trends and potential market turning points, allowing them to anticipate future price changes. Understanding this index is critical for making informed decisions within the agricultural commodity market and related sectors.
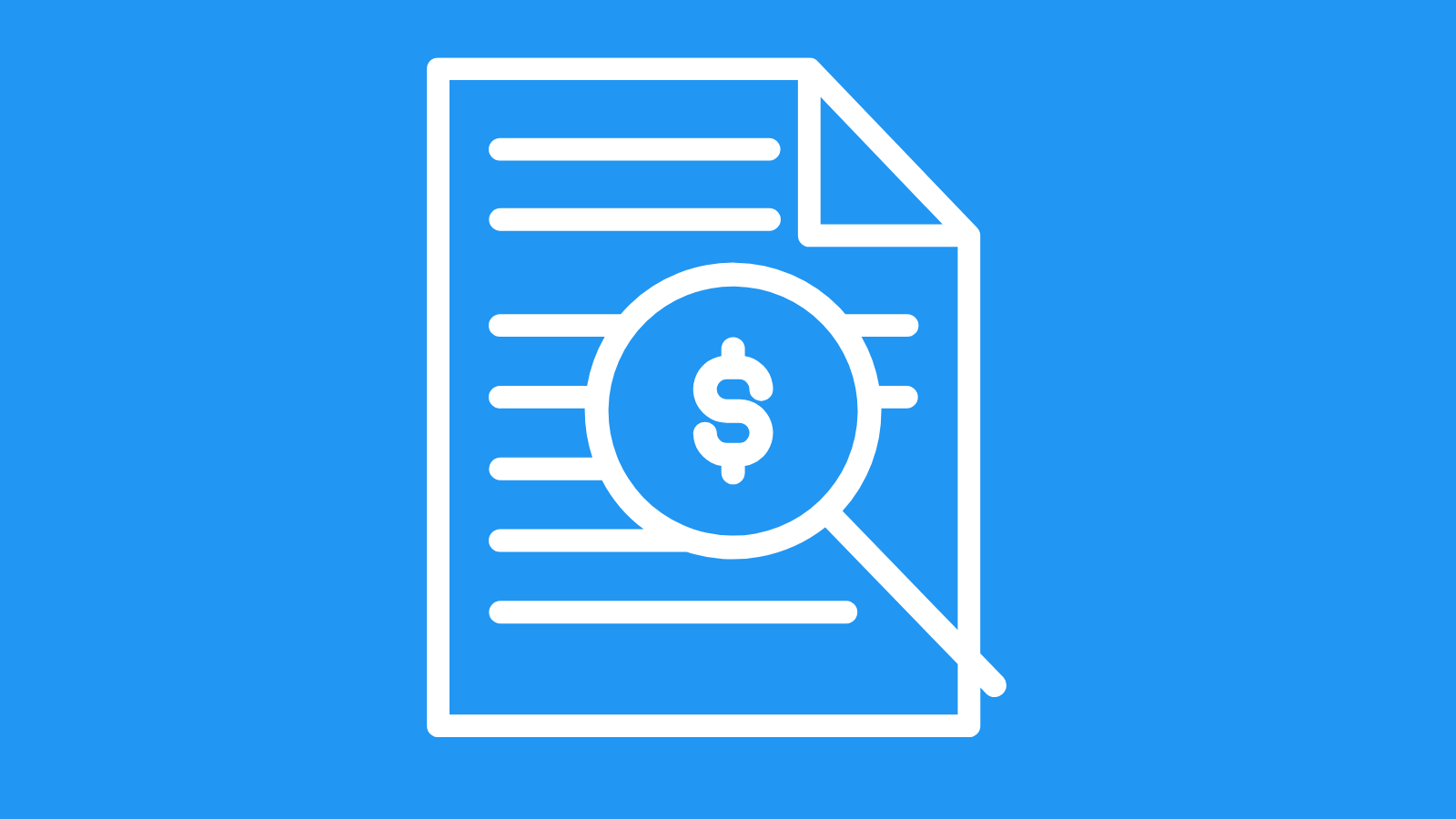
TR/CC CRB Soybeans Index Forecasting Model
This model for forecasting the TR/CC CRB Soybeans index leverages a multi-variate time series approach. We will incorporate historical data on the index itself, alongside crucial economic indicators. This includes factors like global soybean production and consumption forecasts, weather patterns (particularly in key soybean-producing regions), agricultural commodity prices, and international trade policies. Data preprocessing will be a critical step, involving handling missing values, outlier removal, and potentially feature engineering to create new indicators that capture market sentiment or emerging trends. Key economic indicators, such as GDP growth in major importing and exporting nations, will be incorporated to capture macroeconomic influences on commodity prices. The model will employ a combination of statistical and machine learning techniques, such as ARIMA models and possibly recurrent neural networks (RNNs) or long short-term memory (LSTM) networks for capturing complex time-dependent patterns. Rigorous model validation using appropriate metrics, like Mean Absolute Error (MAE) and Root Mean Squared Error (RMSE), will be employed to evaluate forecasting accuracy across different time horizons.
The selection of the appropriate model will depend on the analysis of historical data patterns and the predictive power of various techniques. Preliminary analysis may reveal cyclical behavior, seasonality, or other patterns that can inform the choice of models. We will likely employ a combination of methods to create a robust and accurate forecasting model. This will entail experimenting with various combinations of predictors and model architectures. Furthermore, we will assess the statistical significance of predictor variables through regression analysis and assess their impact on index values. The model will be optimized to provide forecasts at different time horizons, enabling users to anticipate future price movements. Regular model retraining and updates are crucial to reflect evolving market conditions. Backtesting of the model against historical data will be a fundamental component of the process. This will evaluate the model's reliability and refine its parameters to maximize predictive accuracy.
To ensure the model's applicability to real-world scenarios, rigorous validation is essential. A hold-out dataset will be used to evaluate the model's performance on unseen data, preventing overfitting. Regular monitoring of the model's performance will ensure its continued relevance to future index movements. Furthermore, the model's outputs will be interpretable, offering insights into the key factors driving index fluctuations. This will allow for a deeper understanding of market dynamics and potential future challenges. Regular updates to the dataset and re-training of the model will be essential to maintain its accuracy as market conditions evolve. Our chosen model will not only forecast but also generate insights, helping analysts and decision-makers gain a better grasp of the underlying forces shaping the TR/CC CRB Soybeans index.
ML Model Testing
n:Time series to forecast
p:Price signals of TR/CC CRB Soybeans index
j:Nash equilibria (Neural Network)
k:Dominated move of TR/CC CRB Soybeans index holders
a:Best response for TR/CC CRB Soybeans target price
For further technical information as per how our model work we invite you to visit the article below:
How do KappaSignal algorithms actually work?
TR/CC CRB Soybeans Index Forecast Strategic Interaction Table
Strategic Interaction Table Legend:
X axis: *Likelihood% (The higher the percentage value, the more likely the event will occur.)
Y axis: *Potential Impact% (The higher the percentage value, the more likely the price will deviate.)
Z axis (Grey to Black): *Technical Analysis%
TR/CC CRB Soybeans Financial Outlook and Forecast
The TR/CC CRB Soybean market is a crucial component of the global agricultural commodity sector, reflecting the balance between supply and demand, production costs, and market sentiment. Factors such as weather patterns, global economic conditions, and political developments significantly influence prices. The financial outlook for TR/CC CRB Soybeans is contingent upon a complex interplay of these factors. Current market trends, including global demand patterns for soymeal and soybean oil, are pivotal in shaping future price trajectories. Analysis of historical data, coupled with expert opinions and agricultural forecasts, is instrumental in evaluating the potential future performance of the TR/CC CRB Soybean market.
A key element to consider is the projected global soybean production. Factors impacting production, such as favorable or adverse weather conditions during growing seasons, are crucial. Changes in yield per acre and overall acreage dedicated to soybean cultivation significantly influence supply. Similarly, projected demand from various sectors, particularly the livestock feed industry, plays a pivotal role. The demand for soybean oil in the food and industrial sectors is equally significant. The interplay between supply and demand will directly translate to price movements within the TR/CC CRB Soybean market. International trade agreements and policies, including tariffs and import/export regulations, can also considerably affect market dynamics. Geopolitical instability, particularly in regions vital to soybean production or export, can create volatility.
Analyzing historical price trends and their correlation with market events can provide valuable insights into future projections. Examining the impact of significant past events, such as major weather disruptions or unexpected economic shifts, on the TR/CC CRB Soybean market can help develop a framework for anticipating future price fluctuations. Fundamental analysis, incorporating factors such as production costs, yield forecasts, and storage capacity, should complement the evaluation. Technical indicators, including moving averages and price charts, may reveal short-term trends, but they should be used in conjunction with fundamental insights to achieve a more comprehensive view of the market outlook.
The financial outlook for the TR/CC CRB Soybean market, while exhibiting inherent uncertainty, points towards a potential positive forecast. Favorable weather conditions and increased global demand are expected to boost prices in the near term. However, there are inherent risks. Adverse weather patterns, particularly drought or flooding in major producing regions, could significantly reduce yields and exert upward pressure on prices. Unforeseen economic downturns or global conflicts could lead to reduced demand, affecting prices adversely. Finally, fluctuations in international trade policies could create uncertainty and disrupt the flow of soybeans worldwide, thus potentially influencing the price. In conclusion, while a positive outlook is present, the market remains volatile and susceptible to various unforeseen risks.
Rating | Short-Term | Long-Term Senior |
---|---|---|
Outlook | B1 | B1 |
Income Statement | Caa2 | Ba3 |
Balance Sheet | C | B1 |
Leverage Ratios | Baa2 | Ba2 |
Cash Flow | Baa2 | C |
Rates of Return and Profitability | Ba3 | Ba3 |
*An aggregate rating for an index summarizes the overall sentiment towards the companies it includes. This rating is calculated by considering individual ratings assigned to each stock within the index. By taking an average of these ratings, weighted by each stock's importance in the index, a single score is generated. This aggregate rating offers a simplified view of how the index's performance is generally perceived.
How does neural network examine financial reports and understand financial state of the company?
References
- Jiang N, Li L. 2016. Doubly robust off-policy value evaluation for reinforcement learning. In Proceedings of the 33rd International Conference on Machine Learning, pp. 652–61. La Jolla, CA: Int. Mach. Learn. Soc.
- Kitagawa T, Tetenov A. 2015. Who should be treated? Empirical welfare maximization methods for treatment choice. Tech. Rep., Cent. Microdata Methods Pract., Inst. Fiscal Stud., London
- Dimakopoulou M, Zhou Z, Athey S, Imbens G. 2018. Balanced linear contextual bandits. arXiv:1812.06227 [cs.LG]
- J. Harb and D. Precup. Investigating recurrence and eligibility traces in deep Q-networks. In Deep Reinforcement Learning Workshop, NIPS 2016, Barcelona, Spain, 2016.
- R. Rockafellar and S. Uryasev. Optimization of conditional value-at-risk. Journal of Risk, 2:21–42, 2000.
- Bengio Y, Schwenk H, SenĂ©cal JS, Morin F, Gauvain JL. 2006. Neural probabilistic language models. In Innovations in Machine Learning: Theory and Applications, ed. DE Holmes, pp. 137–86. Berlin: Springer
- Nie X, Wager S. 2019. Quasi-oracle estimation of heterogeneous treatment effects. arXiv:1712.04912 [stat.ML]