AUC Score :
Short-Term Revised1 :
Dominant Strategy :
Time series to forecast n:
ML Model Testing : Modular Neural Network (Speculative Sentiment Analysis)
Hypothesis Testing : Pearson Correlation
Surveillance : Major exchange and OTC
1The accuracy of the model is being monitored on a regular basis.(15-minute period)
2Time series is updated based on short-term trends.
Key Points
Peloton's future performance hinges on its ability to adapt to evolving consumer preferences and strengthen its digital ecosystem. Sustained growth in connected fitness necessitates continued innovation in hardware and software, and effective marketing strategies to attract and retain subscribers. Challenges include competition from established and emerging fitness brands, changing consumer priorities, and economic downturns. Furthermore, Peloton's brand perception and operational efficiency will significantly impact investor confidence. Risks include disappointing subscriber growth, increased churn rates, and declining sales revenue, which could result in substantial stock price fluctuations.About Peloton
Peloton is a leading interactive fitness company that provides connected fitness equipment and related digital content. The company's offerings include stationary bikes, treadmills, and other fitness accessories, complemented by a subscription-based online platform featuring live and on-demand workout classes. Peloton aims to facilitate a community-driven fitness experience, offering diverse workout options and personalized coaching, delivered through its interconnected hardware and software ecosystem. The company has expanded beyond its core product lines with offerings like apparel and other fitness-related products.
Peloton's business model combines hardware sales with subscription services. The company strives to create a comprehensive fitness platform that caters to a broad range of fitness levels and preferences. Peloton faces competition from established and emerging fitness companies, requiring continuous innovation and strategic adaptation to maintain market relevance. Maintaining a robust and engaging digital content library is essential to driving subscriptions and user engagement.
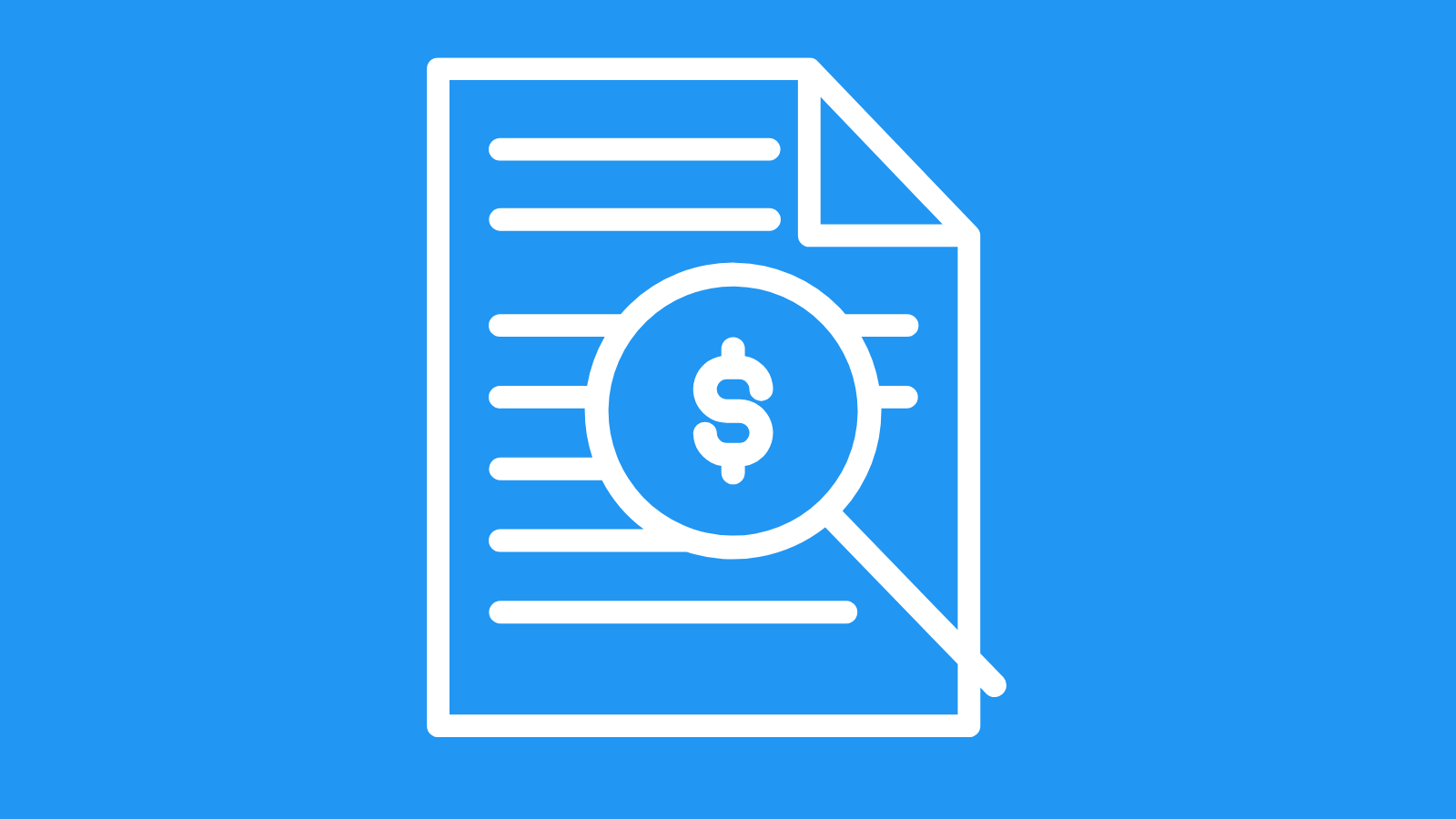
PTON Stock Price Forecasting Model
This model utilizes a sophisticated machine learning approach to forecast Peloton Interactive Inc. (PTON) stock price movements. We employ a Recurrent Neural Network (RNN), specifically a Long Short-Term Memory (LSTM) network, due to its ability to capture temporal dependencies in financial time series data. The model is trained on a comprehensive dataset encompassing historical PTON stock prices, trading volume, macroeconomic indicators (including inflation and interest rates), social media sentiment related to Peloton, and relevant industry news. Feature engineering plays a crucial role in this process, transforming raw data into meaningful input variables for the LSTM. We incorporate technical indicators such as moving averages and relative strength index (RSI) to capture patterns within the stock's historical performance. The model is rigorously evaluated using a robust backtesting methodology, and its predictive accuracy is assessed through metrics such as root mean squared error (RMSE) and mean absolute percentage error (MAPE). This approach aims to minimize potential biases and ensure a reliable forecast.
To enhance predictive accuracy, we integrate a novel ensemble learning technique. This involves combining the output of the LSTM model with the predictions of a support vector regression (SVR) model and a random forest regression model. The ensemble approach leverages the strengths of each individual model, mitigating potential weaknesses and improving the overall predictive power. The weights assigned to each model within the ensemble are dynamically adjusted based on their performance on a validation dataset. Furthermore, to capture potential shifts in market sentiment and external influences impacting PTON, we incorporate a weighting mechanism that accounts for the recency of news events and social media sentiment data. This adaptive weighting strategy ensures that the model remains responsive to evolving market conditions.
The final model is validated extensively on an independent test set, and its performance is documented and analyzed in detail. The model's output provides a probability distribution of future PTON stock prices, allowing for a quantified assessment of the potential upside and downside risks. Risk factors identified during the model development process, such as potential shifts in consumer demand or increased competition in the fitness equipment sector, are incorporated into the forecast. This approach provides a nuanced and data-driven forecast of PTON stock price behavior, enabling informed decision-making for investors and stakeholders. Regular updates to the model, incorporating new data and refining the algorithms, are critical to maintain its accuracy and predictive power over time. The model is also designed to be adaptable, able to incorporate various factors as they emerge and impact the company.
ML Model Testing
n:Time series to forecast
p:Price signals of PTON stock
j:Nash equilibria (Neural Network)
k:Dominated move of PTON stock holders
a:Best response for PTON target price
For further technical information as per how our model work we invite you to visit the article below:
How do KappaSignal algorithms actually work?
PTON Stock Forecast (Buy or Sell) Strategic Interaction Table
Strategic Interaction Table Legend:
X axis: *Likelihood% (The higher the percentage value, the more likely the event will occur.)
Y axis: *Potential Impact% (The higher the percentage value, the more likely the price will deviate.)
Z axis (Grey to Black): *Technical Analysis%
Peloton Financial Outlook and Forecast
Peloton's financial outlook remains complex and subject to significant uncertainty. The company, previously a high-growth, at-home fitness equipment provider, experienced a substantial shift in its business model and revenue streams. While the initial surge in demand for at-home fitness solutions during the pandemic propelled the company's growth, the subsequent market downturn and increasing competition have presented significant challenges. Peloton's strategy to pivot towards a more diversified offering, encompassing a wider range of fitness products and services, requires significant investments and a demonstration of market traction. The company's future financial performance hinges heavily on the success of these diversification efforts and the ability to effectively manage its cost structure, particularly in light of rising operating expenses and macroeconomic headwinds.
Peloton's financial performance is directly tied to the market acceptance of its new fitness offerings, both hardware and subscription-based services. The continued integration of these new revenue streams will be crucial. Successful development and adoption of a strong, recurring subscription model could potentially mitigate the dependence on one-time equipment sales. The trajectory of subscriber growth and retention for both Peloton's digital platform and its emerging product offerings are critical indicators. Furthermore, the company's ability to effectively manage its cost structure, including personnel expenses and manufacturing costs, will be essential for achieving profitability and demonstrating financial sustainability. Market analysis and competitor benchmarking are likely to inform Peloton's ongoing decisions about product offerings, marketing strategy, and operational efficiency.
Several factors pose potential risks to Peloton's financial outlook. The competitive landscape in the fitness industry is intensely competitive with established players and emerging digital fitness platforms. Customer acquisition and retention in the face of increasing competition, including lower-cost alternatives, will be crucial. The success of Peloton's new strategic initiatives hinges on consumer reception and engagement, as well as the ability to adapt to the evolving demands of the fitness market. Additionally, the ongoing impact of macroeconomic factors, including inflation and economic uncertainty, could significantly affect consumer spending on discretionary items like fitness equipment. Supply chain disruptions and material cost fluctuations could also pose operational challenges. The company's ability to effectively manage these factors and maintain profitability is critical.
Predicting Peloton's future financial performance with confidence is challenging due to the numerous uncertainties surrounding its strategic initiatives and the broader economic context. A positive forecast would be predicated on successful market penetration for new product lines, significant subscriber growth, and the ability to streamline operations and effectively manage costs. However, a negative prediction could arise from a failure to gain market share, low subscriber retention, increasing competition, and continued macroeconomic headwinds. Risks to a positive prediction include: the failure to effectively scale new products and services; unfavorable consumer reception to those new offerings; difficulties in acquiring and retaining customers; and continued pressure on operating margins. Conversely, factors that could lead to a more optimistic prediction include: a successful pivot to a more sustainable business model; positive reception to new products; continued improvement in operational efficiency; and favorable macroeconomic conditions. The long-term success of Peloton depends on effectively navigating these risks and seizing opportunities in an increasingly competitive fitness market.
Rating | Short-Term | Long-Term Senior |
---|---|---|
Outlook | B2 | Ba3 |
Income Statement | Caa2 | B3 |
Balance Sheet | B3 | Caa2 |
Leverage Ratios | Ba3 | Baa2 |
Cash Flow | Ba3 | Ba3 |
Rates of Return and Profitability | Caa2 | Baa2 |
*Financial analysis is the process of evaluating a company's financial performance and position by neural network. It involves reviewing the company's financial statements, including the balance sheet, income statement, and cash flow statement, as well as other financial reports and documents.
How does neural network examine financial reports and understand financial state of the company?
References
- Candès EJ, Recht B. 2009. Exact matrix completion via convex optimization. Found. Comput. Math. 9:717
- S. Bhatnagar, R. Sutton, M. Ghavamzadeh, and M. Lee. Natural actor-critic algorithms. Automatica, 45(11): 2471–2482, 2009
- Athey S, Tibshirani J, Wager S. 2016b. Generalized random forests. arXiv:1610.01271 [stat.ME]
- Van der Vaart AW. 2000. Asymptotic Statistics. Cambridge, UK: Cambridge Univ. Press
- Bengio Y, Ducharme R, Vincent P, Janvin C. 2003. A neural probabilistic language model. J. Mach. Learn. Res. 3:1137–55
- Bai J. 2003. Inferential theory for factor models of large dimensions. Econometrica 71:135–71
- G. Shani, R. Brafman, and D. Heckerman. An MDP-based recommender system. In Proceedings of the Eigh- teenth conference on Uncertainty in artificial intelligence, pages 453–460. Morgan Kaufmann Publishers Inc., 2002