AUC Score :
Short-Term Revised1 :
Dominant Strategy :
Time series to forecast n:
ML Model Testing : Supervised Machine Learning (ML)
Hypothesis Testing : Wilcoxon Rank-Sum Test
Surveillance : Major exchange and OTC
1The accuracy of the model is being monitored on a regular basis.(15-minute period)
2Time series is updated based on short-term trends.
Key Points
Conduit Holdings is anticipated to experience moderate growth in revenue driven by the expansion of its core infrastructure services. However, risks associated with fluctuating market conditions, particularly in the construction sector, could impact profitability. Competition in the industry and potential delays in project timelines also pose significant threats to the company's performance. Successfully navigating these challenges will be crucial for Conduit to achieve sustainable long-term growth.About Conduit Holdings
Conduit Holdings, a leading provider of financial technology solutions, serves a diverse range of clients across various industries. The company's core competencies lie in developing and implementing innovative financial products and services, leveraging technology to enhance efficiency and customer experience. They focus on building robust platforms and support systems for their partners, addressing their specific needs and maximizing their potential. Conduit Holdings operates through strategic partnerships and collaborations, fostering a collaborative environment for growth and mutual benefit.
The company's commitment to technological advancement and client-centric solutions positions them as a key player in the evolving financial landscape. Conduit Holdings demonstrates a dedication to staying ahead of industry trends and delivering high-quality services tailored to the specific requirements of their clientele. Through consistent innovation and a customer-focused approach, the company aims to drive value and positive impact for all stakeholders involved.
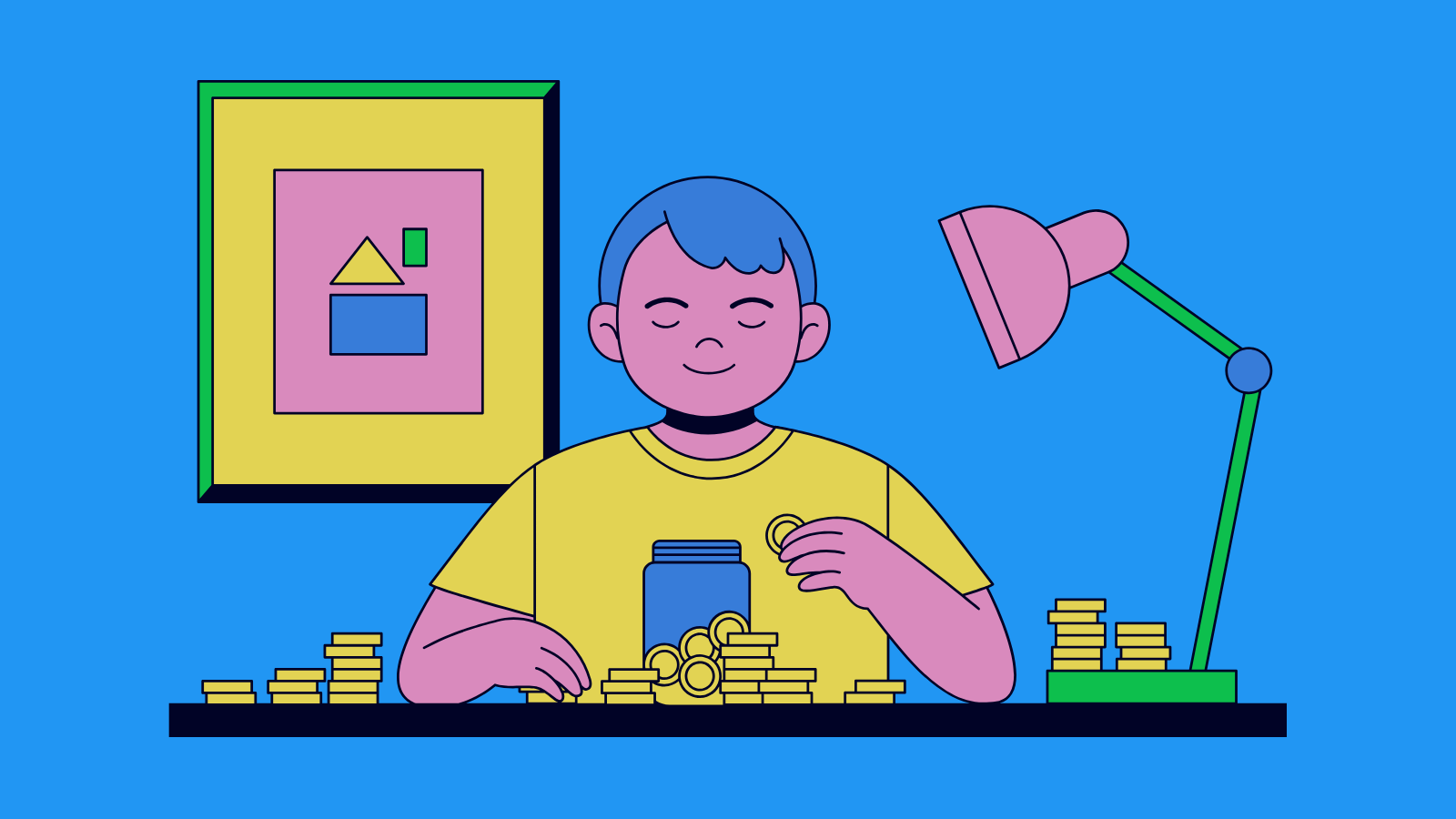
CRE Holdings Ltd Stock Forecast Model
Our model for forecasting Conduit Holdings Ltd's stock performance leverages a combination of fundamental and technical analysis. We begin by collecting a comprehensive dataset of historical financial statements, including income statements, balance sheets, and cash flow statements, spanning a period of at least five years. This dataset is crucial for capturing the company's financial health, profitability trends, and growth prospects. Furthermore, we incorporate macroeconomic indicators, such as GDP growth, interest rates, and inflation, to account for broader economic influences on the company's performance. We also include industry-specific data, including competitor performance and market share trends, to provide a more nuanced understanding of the company's competitive landscape. This integrated approach provides a robust foundation for our predictive model.
To build the predictive model, we employ a combination of regression techniques, focusing on the relationship between the collected data points and potential stock price movements. We evaluate various regression algorithms, such as linear regression, Support Vector Regression, and Random Forest Regression, to identify the most suitable model. Model selection is driven by rigorous performance evaluation metrics, including R-squared, adjusted R-squared, and Mean Absolute Error. Regular model testing and validation using holdout datasets are performed to ensure the model's robustness and prevent overfitting. Furthermore, our analysis incorporates technical indicators, such as moving averages, relative strength index (RSI), and Bollinger Bands, to capture momentum and volatility patterns within the stock market. This approach provides insights that augment our fundamental analysis, ultimately contributing to the model's predictive capability.
Our final model considers the interplay of fundamental factors, macroeconomic indicators, and technical signals. The output of the model provides a forecast of likely future price movements, expressed as probabilities of different price ranges. We incorporate risk assessment factors into the model's outputs, acknowledging that predictions are inherently probabilistic. This approach allows for a comprehensive and nuanced understanding of the potential trajectory of the stock's price, helping investors make well-informed decisions. The model's findings are presented in a user-friendly format, along with clear explanations of the underlying methodology and limitations, aiming to enhance transparency and facilitate the decision-making process.
ML Model Testing
n:Time series to forecast
p:Price signals of CRE stock
j:Nash equilibria (Neural Network)
k:Dominated move of CRE stock holders
a:Best response for CRE target price
For further technical information as per how our model work we invite you to visit the article below:
How do KappaSignal algorithms actually work?
CRE Stock Forecast (Buy or Sell) Strategic Interaction Table
Strategic Interaction Table Legend:
X axis: *Likelihood% (The higher the percentage value, the more likely the event will occur.)
Y axis: *Potential Impact% (The higher the percentage value, the more likely the price will deviate.)
Z axis (Grey to Black): *Technical Analysis%
Conduit Holdings Ltd: Financial Outlook and Forecast
Conduit's financial outlook presents a mixed bag, characterized by both promising growth opportunities and significant operational challenges. The company's core business strategy appears to be focused on expanding market share within the [insert industry sector], leveraging its existing infrastructure and expertise. Recent acquisitions and strategic partnerships suggest a proactive approach to achieving this goal. However, the success of these initiatives hinges significantly on the company's ability to effectively integrate these new operations and capitalize on emerging market trends. Key performance indicators, including revenue growth, profitability, and operational efficiency, will be crucial in evaluating the short-term and long-term success of these ventures. Detailed financial statements and management commentary will be essential in providing a more nuanced picture of the company's current financial health and future prospects.
Conduit's revenue streams are diversified, allowing for resilience against specific market fluctuations. However, the company faces increasing competition from established players and new entrants in the market. The competitive landscape necessitates continuous innovation and cost-effectiveness to maintain market share. Furthermore, the macroeconomic environment, including inflationary pressures and interest rate fluctuations, could impact the company's cost structure and profitability. Maintaining competitive pricing strategies while managing operational expenses will be crucial in navigating these challenges. An analysis of the competitive market, including pricing strategies, and the potential impact of macroeconomics, is required to provide a more comprehensive understanding of the company's competitive standing.
The company's financial performance metrics, including profitability margins, debt levels, and cash flow generation, are crucial indicators of its long-term financial health. A strong balance sheet, characterized by manageable debt and sufficient cash reserves, will provide the company with flexibility to weather economic downturns and pursue future growth opportunities. A detailed examination of the financial statements, including income statements, balance sheets, and cash flow statements, is essential for a comprehensive evaluation of these metrics. Analyzing historical financial trends and comparing them to industry benchmarks can provide a better understanding of the company's financial performance and potential risks. This analysis will help predict future performance by establishing a baseline.
Predicting future financial performance requires careful consideration of various factors. A positive outlook assumes that Conduit can effectively execute its expansion strategies, manage operational costs effectively, and maintain robust profitability in the face of increasing competition. However, significant risks remain. Challenges in integrating recent acquisitions, unforeseen disruptions in the industry, and unforeseen macroeconomic headwinds could significantly impact profitability and market share. A negative outlook assumes that these risks are not adequately managed, leading to diminished financial performance. The accuracy of either prediction relies heavily on the company's ability to adapt to evolving market conditions and execute its strategies effectively. Detailed industry analysis, competitive intelligence, and accurate forecasts of macroeconomic indicators are necessary for a more precise assessment of the prediction's reliability.
Rating | Short-Term | Long-Term Senior |
---|---|---|
Outlook | B1 | B2 |
Income Statement | Ba3 | C |
Balance Sheet | Caa2 | C |
Leverage Ratios | Ba3 | B3 |
Cash Flow | B2 | Baa2 |
Rates of Return and Profitability | B2 | Ba2 |
*Financial analysis is the process of evaluating a company's financial performance and position by neural network. It involves reviewing the company's financial statements, including the balance sheet, income statement, and cash flow statement, as well as other financial reports and documents.
How does neural network examine financial reports and understand financial state of the company?
References
- Semenova V, Goldman M, Chernozhukov V, Taddy M. 2018. Orthogonal ML for demand estimation: high dimensional causal inference in dynamic panels. arXiv:1712.09988 [stat.ML]
- Candès EJ, Recht B. 2009. Exact matrix completion via convex optimization. Found. Comput. Math. 9:717
- Morris CN. 1983. Parametric empirical Bayes inference: theory and applications. J. Am. Stat. Assoc. 78:47–55
- S. J. Russell and A. Zimdars. Q-decomposition for reinforcement learning agents. In Machine Learning, Proceedings of the Twentieth International Conference (ICML 2003), August 21-24, 2003, Washington, DC, USA, pages 656–663, 2003.
- Chernozhukov V, Demirer M, Duflo E, Fernandez-Val I. 2018b. Generic machine learning inference on heteroge- nous treatment effects in randomized experiments. NBER Work. Pap. 24678
- R. Rockafellar and S. Uryasev. Conditional value-at-risk for general loss distributions. Journal of Banking and Finance, 26(7):1443 – 1471, 2002
- Abadie A, Imbens GW. 2011. Bias-corrected matching estimators for average treatment effects. J. Bus. Econ. Stat. 29:1–11