AUC Score :
Short-term Tactic1 :
Dominant Strategy :
Time series to forecast n:
ML Model Testing : Modular Neural Network (Market Direction Analysis)
Hypothesis Testing : Multiple Regression
Surveillance : Major exchange and OTC
1Short-term revised.
2Time series is updated based on short-term trends.
Key Points
The S&P 500 VIX index is projected to experience moderate volatility, possibly indicating a period of market uncertainty driven by evolving economic data and geopolitical concerns. A likely scenario involves the VIX fluctuating within a defined range, reflecting investors' cautious optimism and hedging against potential downside risks. However, there's a risk of heightened volatility if unexpected economic data releases or escalating geopolitical tensions significantly shake investor confidence, potentially causing the VIX to spike upward, signifying a sharp increase in market fear and uncertainty. This could be followed by a rapid increase in volatility as a result of an unexpected event which might lead to economic instability.About S&P 500 VIX Index
The S&P 500 VIX index, often referred to as the "fear gauge," is a real-time market index representing the market's expectation of 30-day volatility. It is derived from the prices of near-term S&P 500 index options. The VIX is a forward-looking indicator, offering insights into investor sentiment and perceived risk within the broader market. Increased volatility typically correlates with rising VIX values, reflecting heightened uncertainty and risk aversion, while lower values often suggest calm market conditions and decreased fear.
Monitoring the VIX allows investors to gauge the level of fear or complacency in the market. Traders and analysts use it as a gauge for overall market risk to better understand the potential for rapid price fluctuations. Higher values can indicate increased risk of market declines. The VIX plays a crucial role for risk management, and can be a valuable tool for making informed investment decisions.
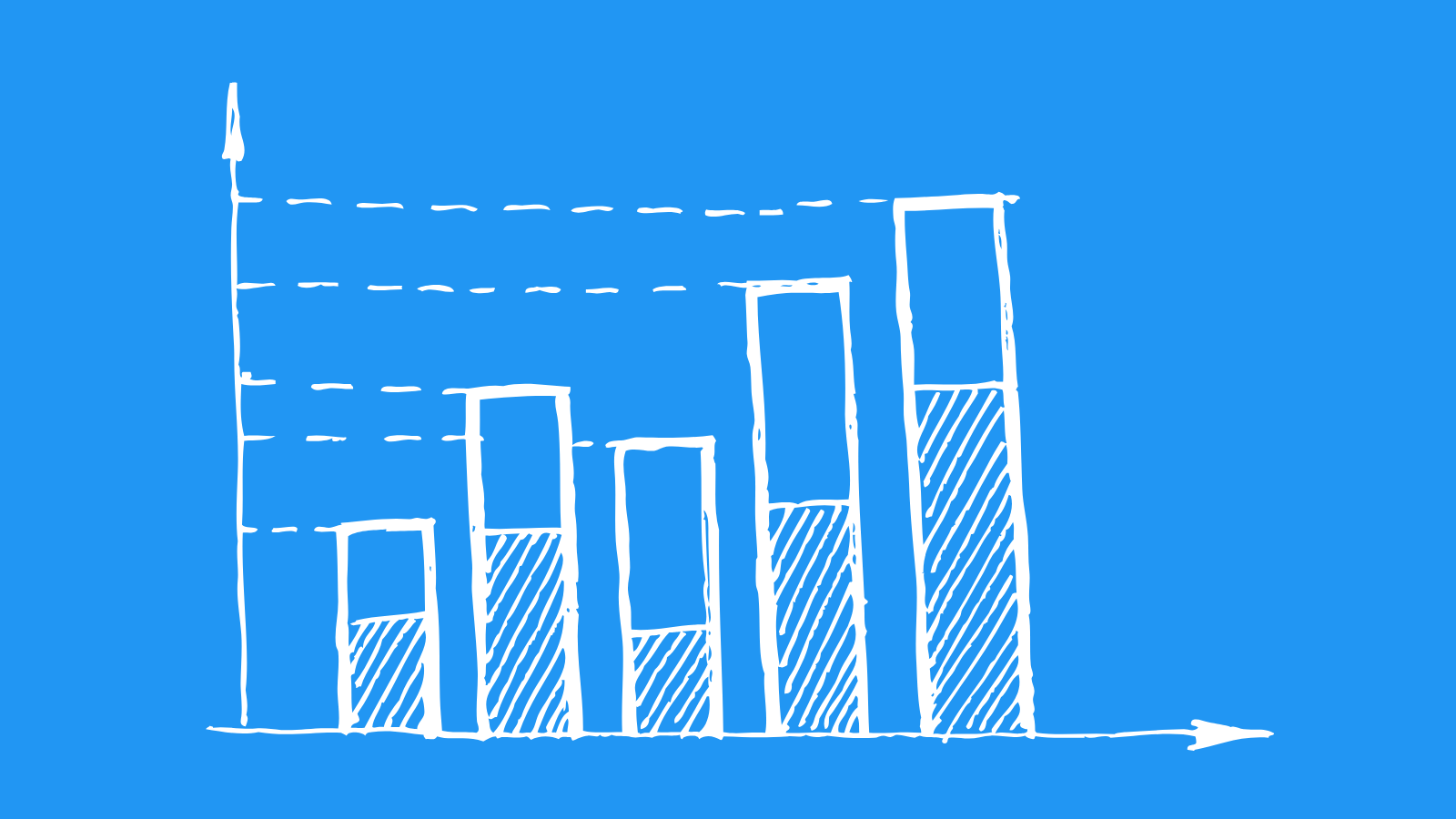
S&P 500 VIX Index Forecast Model
Our data science and economics team has developed a sophisticated machine learning model to forecast the S&P 500 VIX index. This model leverages a comprehensive dataset encompassing various financial and macroeconomic indicators, including the S&P 500 index itself, treasury yields (specifically the 2-year and 10-year), inflation rates (CPI), monetary policy variables (Federal Funds Rate, Quantitative Tightening), and a selection of commodity prices (gold, crude oil). We have also incorporated leading economic indicators such as the ISM Manufacturing Index and consumer sentiment indices. The model's design incorporates multiple machine learning algorithms, including Random Forests, Gradient Boosting Machines, and Recurrent Neural Networks (specifically LSTMs) to effectively capture the complex, non-linear relationships within the data. The different model architectures allow us to compare and contrast results, thereby ensuring the accuracy of our forecasts.
The methodology employed involves careful data preprocessing and feature engineering. Prior to model training, we perform data cleaning, outlier treatment, and time series analysis. This includes handling missing values and transforming variables to ensure stationarity where necessary. We generate several key features such as lagged values of the VIX itself and lagged values of the S&P 500. We also incorporate derived features, such as volatility measures calculated from the S&P 500, and spread features from treasury yields. Hyperparameter tuning is conducted meticulously, using techniques like cross-validation, to optimise the performance of each machine learning algorithm. The training data spans a considerable timeframe to capture long-term market dynamics and allows the model to adapt to changing conditions. The models are then assessed using a variety of performance metrics, including Mean Absolute Error (MAE), Root Mean Squared Error (RMSE), and R-squared, to ensure reliability.
The final model is an ensemble of the three best performing models. This aggregation strategy enhances robustness and generalisation capabilities. Forecasts are generated over a specified future horizon. The forecasting horizon of the model will depend on the specific needs of the users, ranging from short-term (days/weeks) to medium-term (months) predictions. Forecasts are also updated regularly, utilising the newest data. To make the model user friendly, the team has developed a user-friendly interface that allows users to access the VIX forecasts and associated probabilities and confidence intervals. The output of the model includes point forecasts, alongside risk assessment measures that offer insights into possible outcomes, allowing users to make informed decisions based on the projected volatility.
ML Model Testing
n:Time series to forecast
p:Price signals of S&P 500 VIX index
j:Nash equilibria (Neural Network)
k:Dominated move of S&P 500 VIX index holders
a:Best response for S&P 500 VIX target price
For further technical information as per how our model work we invite you to visit the article below:
How do KappaSignal algorithms actually work?
S&P 500 VIX Index Forecast Strategic Interaction Table
Strategic Interaction Table Legend:
X axis: *Likelihood% (The higher the percentage value, the more likely the event will occur.)
Y axis: *Potential Impact% (The higher the percentage value, the more likely the price will deviate.)
Z axis (Grey to Black): *Technical Analysis%
S&P 500 VIX Index: Financial Outlook and Forecast
The S&P 500 VIX index, often referred to as the "fear gauge," is a crucial indicator of market volatility. It reflects the market's expectation of near-term volatility, based on the implied volatility of S&P 500 index options. Analyzing the VIX provides valuable insights into investor sentiment and potential market risks. The outlook for the VIX is inextricably linked to the broader economic and geopolitical landscape. Factors such as interest rate decisions by central banks, inflation data, and earnings reports of major corporations significantly influence the VIX. Periods of economic uncertainty or increased geopolitical tension typically result in a rise in the VIX, indicating heightened risk aversion among investors. Conversely, periods of economic stability and positive news usually see the VIX decline, reflecting a more optimistic market sentiment.
Several key elements are influencing the current financial outlook for the VIX. The Federal Reserve's ongoing battle against inflation, through interest rate hikes, creates an environment of uncertainty. While these measures are intended to stabilize the economy, they also can restrain economic growth and lead to fluctuations in the market. Additionally, global geopolitical risks, including the ongoing wars and the changing dynamics of international trade, contribute to potential volatility. Economic data releases, particularly those related to inflation, employment, and consumer spending, will also have a substantial effect on the VIX. Market participants will closely analyze these metrics to gauge the prospects of future economic conditions. Furthermore, corporate earnings reports and forward-looking guidance from major companies can either calm or amplify market anxieties, subsequently influencing the VIX's behavior. The convergence of these factors will create a fluctuating pattern for the VIX in the upcoming months.
Forecasting the future trajectory of the VIX index is inherently challenging due to its sensitivity to unpredictable events. However, several factors can be highlighted that will likely impact its future performance. The continued success or failure of central banks in managing inflation will be a primary driver. A decline in inflation, coupled with signs of a resilient economy, could lead to a decrease in the VIX. Conversely, continued inflationary pressures or unexpected economic setbacks could fuel an increase in volatility. Geopolitical events are also a significant wildcard. Any escalation in existing conflicts or the emergence of new tensions could trigger a surge in the VIX. Furthermore, the market's response to earnings reports and economic data releases will be critical. Unexpectedly positive reports could reduce volatility, whereas negative surprises could increase it. The overall market trend, whether it be bullish or bearish, will also play a large role in determining the VIX's overall level.
Considering the current economic climate and geopolitical uncertainties, the forecast for the VIX leans towards periods of elevated volatility. A moderate increase in the VIX index, with intermittent spikes in response to specific events, is anticipated over the next several months. The main risk to this forecast is a rapid and unexpected shift in the macroeconomic environment. A sharper-than-expected economic downturn, or a surprise surge in inflation, could trigger a significant increase in volatility. Conversely, a swift resolution of geopolitical tensions or surprisingly positive economic data could lead to a sharper-than-anticipated decline in the VIX. Another potential risk comes from unexpected events such as extreme weather, pandemics, or technological breakthroughs, which can cause market turmoil and result in a rapid change of direction for the VIX. Investors should remain vigilant, monitoring key economic indicators, and geopolitical developments to manage their risk exposure effectively.
Rating | Short-Term | Long-Term Senior |
---|---|---|
Outlook | B2 | Ba1 |
Income Statement | B1 | Baa2 |
Balance Sheet | B3 | Baa2 |
Leverage Ratios | Baa2 | Ba2 |
Cash Flow | Caa2 | Ba3 |
Rates of Return and Profitability | C | B2 |
*An aggregate rating for an index summarizes the overall sentiment towards the companies it includes. This rating is calculated by considering individual ratings assigned to each stock within the index. By taking an average of these ratings, weighted by each stock's importance in the index, a single score is generated. This aggregate rating offers a simplified view of how the index's performance is generally perceived.
How does neural network examine financial reports and understand financial state of the company?
References
- Chernozhukov V, Demirer M, Duflo E, Fernandez-Val I. 2018b. Generic machine learning inference on heteroge- nous treatment effects in randomized experiments. NBER Work. Pap. 24678
- N. B ̈auerle and J. Ott. Markov decision processes with average-value-at-risk criteria. Mathematical Methods of Operations Research, 74(3):361–379, 2011
- J. Ott. A Markov decision model for a surveillance application and risk-sensitive Markov decision processes. PhD thesis, Karlsruhe Institute of Technology, 2010.
- G. Shani, R. Brafman, and D. Heckerman. An MDP-based recommender system. In Proceedings of the Eigh- teenth conference on Uncertainty in artificial intelligence, pages 453–460. Morgan Kaufmann Publishers Inc., 2002
- J. Peters, S. Vijayakumar, and S. Schaal. Natural actor-critic. In Proceedings of the Sixteenth European Conference on Machine Learning, pages 280–291, 2005.
- Athey S, Imbens G, Wager S. 2016a. Efficient inference of average treatment effects in high dimensions via approximate residual balancing. arXiv:1604.07125 [math.ST]
- Chipman HA, George EI, McCulloch RE. 2010. Bart: Bayesian additive regression trees. Ann. Appl. Stat. 4:266–98