AUC Score :
Short-term Tactic1 :
Dominant Strategy :
Time series to forecast n:
ML Model Testing : Modular Neural Network (Emotional Trigger/Responses Analysis)
Hypothesis Testing : Multiple Regression
Surveillance : Major exchange and OTC
1Short-term revised.
2Time series is updated based on short-term trends.
Key Points
The TR/CC CRB index is likely to experience moderate volatility, driven by shifts in global demand and supply dynamics across its constituent commodities. Expectations of increased infrastructure spending in various countries could provide upward pressure, potentially leading to gains in industrial metals and energy sectors. However, persistent inflationary concerns and potential interest rate hikes by central banks globally pose significant risks, potentially curbing economic activity and subsequently dampening demand for commodities, resulting in price corrections. Furthermore, geopolitical uncertainties and unforeseen supply disruptions, such as weather events impacting agricultural production, could introduce unpredictable swings in the index.About TR/CC CRB Index
The TR/CC CRB index, formerly known as the CRB (Commodity Research Bureau) Index, is a benchmark reflecting the price movements of a diversified basket of commodities. It's a broad indicator used by investors and analysts to gauge overall commodity market trends and inflation pressures. The index is designed to provide a comprehensive view of the commodity market, encompassing various sectors, including energy, agriculture, precious metals, and industrial metals. Its fluctuations offer insights into supply and demand dynamics and the broader economic landscape.
This index is a key reference point for understanding the performance of commodities as an asset class. Its composition and weighting methodologies are carefully constructed to balance representation across different commodity groups. Monitoring the TR/CC CRB can offer valuable information for those seeking to diversify their portfolios or gain exposure to the commodity market. It is commonly used as a comparative measure to assess the performance of commodity-related investments and assess broader market sentiment.
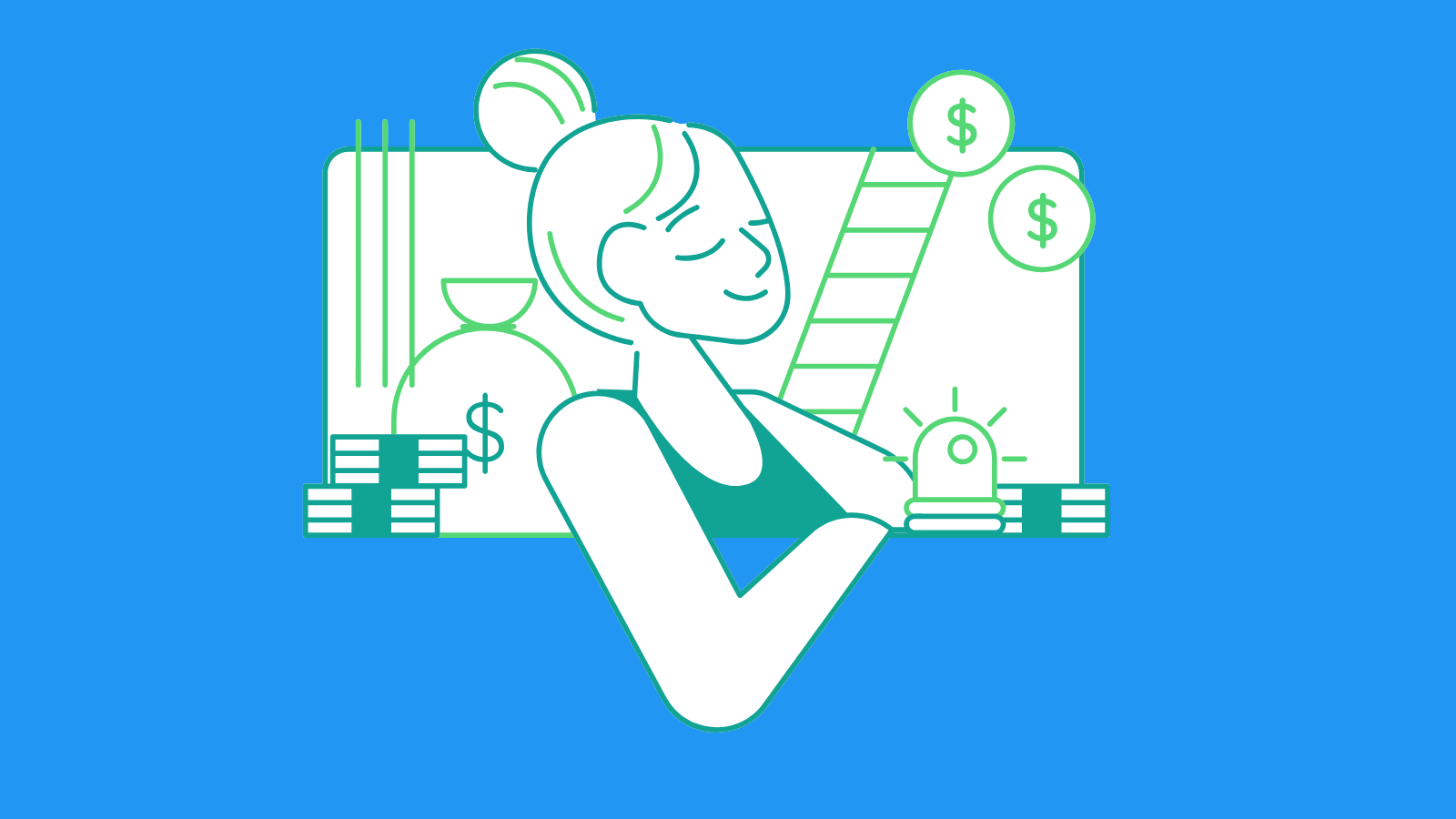
TR/CC CRB Index Forecast Model
As a collective of data scientists and economists, our approach to forecasting the Thomson Reuters/CoreCommodity CRB (TR/CC CRB) index necessitates a robust machine learning model. We will employ a time-series-based methodology, recognizing the inherently sequential nature of commodity price fluctuations. The model will be built upon a foundation of historical data, encompassing the past performance of the TR/CC CRB index itself, coupled with a selection of crucial predictor variables. These variables will include leading economic indicators such as consumer price indices, manufacturing purchasing managers' indices, and global economic growth figures. Furthermore, we will integrate commodity-specific factors, including supply and demand dynamics for the individual components of the TR/CC CRB index (e.g., energy, metals, agricultural products), and information regarding inventory levels and production costs. A critical element will be incorporating macroeconomic variables, such as interest rate trends, currency exchange rates, and geopolitical risk assessments, which can significantly impact commodity markets.
The model will utilize a combination of machine learning algorithms, selected based on their demonstrated efficacy in time-series forecasting and their capacity to manage high-dimensional data. We will consider algorithms like Recurrent Neural Networks (RNNs), particularly Long Short-Term Memory (LSTM) networks, due to their ability to capture long-range dependencies within the data. We will also consider ensemble methods, such as Random Forests or Gradient Boosting, to improve the accuracy and reduce the risk of overfitting. Model training will incorporate rigorous cross-validation techniques to ensure the robustness and generalizability of the model. The selection and tuning of the algorithms will be driven by rigorous statistical analysis and will be based on appropriate metrics such as mean absolute error, root mean squared error, and R-squared, which are designed for evaluating the predictive power of time-series models. The model's parameters will be optimized using techniques like hyperparameter tuning and regularization.
The model's output will be a multi-period forecast of the TR/CC CRB index. We will provide point forecasts as well as probabilistic forecasts, which offer a range of possible outcomes and associated probabilities, allowing for risk assessment. The model's performance will be continuously monitored and evaluated against the actual historical data, with periodic updates to the model incorporating newly available data. This adaptive strategy ensures model accuracy and relevance in response to dynamic market conditions. The model will be regularly reviewed by economists to incorporate qualitative insights and maintain its capacity to effectively explain the movement of the TR/CC CRB index based on our understanding of market fundamentals and current events. The model will be designed to provide accurate forecasts that would be crucial to traders, investors, and other stakeholders in the commodities market.
```
ML Model Testing
n:Time series to forecast
p:Price signals of TR/CC CRB index
j:Nash equilibria (Neural Network)
k:Dominated move of TR/CC CRB index holders
a:Best response for TR/CC CRB target price
For further technical information as per how our model work we invite you to visit the article below:
How do KappaSignal algorithms actually work?
TR/CC CRB Index Forecast Strategic Interaction Table
Strategic Interaction Table Legend:
X axis: *Likelihood% (The higher the percentage value, the more likely the event will occur.)
Y axis: *Potential Impact% (The higher the percentage value, the more likely the price will deviate.)
Z axis (Grey to Black): *Technical Analysis%
TR/CC CRB Index: Financial Outlook and Forecast
The TR/CC CRB Index, a widely recognized benchmark for commodity prices, reflects the performance of a diversified basket of raw materials. Understanding its financial outlook requires a comprehensive analysis of global economic conditions, supply and demand dynamics within each commodity sector, and prevailing geopolitical influences. Currently, the index is influenced by a confluence of factors, including persistent inflationary pressures, supply chain disruptions, and evolving energy markets. The ongoing conflict in Ukraine continues to exert upward pressure on energy and agricultural commodity prices, while the recovery in global demand, particularly from emerging markets, is creating strong underlying demand. Furthermore, investor sentiment and speculative activity in commodity markets can amplify price volatility, making accurate forecasting a complex endeavor. Understanding these interwoven elements is vital for assessing the index's future trajectory.
The financial forecast for the TR/CC CRB Index hinges on several crucial drivers. Changes in monetary policy, such as interest rate adjustments by central banks, have a significant impact on commodity prices. Higher interest rates can curb economic growth, potentially dampening demand for commodities. Conversely, loose monetary policies can spur economic activity and, consequently, commodity consumption. Moreover, supply-side factors, like production output from major commodity-producing countries, can greatly influence prices. Factors like weather patterns, geopolitical risks and natural disasters impacting agricultural production, mining, and energy extraction will also play a large role. Finally, the demand outlook from major consumers such as China, India, and the developed economies is a key element. Economic expansion in these regions could fuel demand for commodities, driving up prices, while economic slowdowns could have the opposite effect.
Recent economic indicators suggest a mixed outlook for the TR/CC CRB Index. While global growth has slowed somewhat, robust demand from emerging economies continues to support commodity prices. Energy markets remain volatile, with geopolitical tensions influencing oil and natural gas prices. Agricultural commodities have exhibited price variations, affected by weather-related production setbacks and global supply chains. Metal prices are susceptible to fluctuations linked with industrial activity and inventory levels. Although some commodity sectors may face headwinds due to weaker demand in certain regions, overall demand still appears strong, especially from areas undergoing rapid infrastructure development. Investors may consider that the ongoing geopolitical and economic uncertainty contributes to the overall unpredictability of the index.
Based on these factors, the TR/CC CRB Index is expected to experience moderate gains over the next year. The positive outlook is predicated on continued demand from emerging markets, stabilization in supply chains, and the expectation that energy markets will gradually adjust to current disruptions. However, there are several risks. A significant global economic slowdown could significantly reduce demand and lower prices. Continued geopolitical instability, potentially further disrupting supply chains, may result in considerable price swings. Additionally, unexpected changes in monetary policy could curb demand or trigger significant investor sell-offs. Therefore, while the index is forecast to increase, investors should consider hedging strategies to protect against potential market volatility and economic downturns.
Rating | Short-Term | Long-Term Senior |
---|---|---|
Outlook | Ba3 | B2 |
Income Statement | C | Baa2 |
Balance Sheet | Baa2 | C |
Leverage Ratios | C | B3 |
Cash Flow | Baa2 | C |
Rates of Return and Profitability | Baa2 | Ba3 |
*An aggregate rating for an index summarizes the overall sentiment towards the companies it includes. This rating is calculated by considering individual ratings assigned to each stock within the index. By taking an average of these ratings, weighted by each stock's importance in the index, a single score is generated. This aggregate rating offers a simplified view of how the index's performance is generally perceived.
How does neural network examine financial reports and understand financial state of the company?
References
- R. Rockafellar and S. Uryasev. Optimization of conditional value-at-risk. Journal of Risk, 2:21–42, 2000.
- Abadie A, Diamond A, Hainmueller J. 2015. Comparative politics and the synthetic control method. Am. J. Political Sci. 59:495–510
- J. Z. Leibo, V. Zambaldi, M. Lanctot, J. Marecki, and T. Graepel. Multi-agent Reinforcement Learning in Sequential Social Dilemmas. In Proceedings of the 16th International Conference on Autonomous Agents and Multiagent Systems (AAMAS 2017), Sao Paulo, Brazil, 2017
- P. Marbach. Simulated-Based Methods for Markov Decision Processes. PhD thesis, Massachusetts Institute of Technology, 1998
- F. A. Oliehoek, M. T. J. Spaan, and N. A. Vlassis. Optimal and approximate q-value functions for decentralized pomdps. J. Artif. Intell. Res. (JAIR), 32:289–353, 2008
- Friedberg R, Tibshirani J, Athey S, Wager S. 2018. Local linear forests. arXiv:1807.11408 [stat.ML]
- Jorgenson, D.W., Weitzman, M.L., ZXhang, Y.X., Haxo, Y.M. and Mat, Y.X., 2023. Can Neural Networks Predict Stock Market?. AC Investment Research Journal, 220(44).