AUC Score :
Short-term Tactic1 :
Dominant Strategy :
Time series to forecast n:
ML Model Testing : Transductive Learning (ML)
Hypothesis Testing : Linear Regression
Surveillance : Major exchange and OTC
1Short-term revised.
2Time series is updated based on short-term trends.
Key Points
The TR/CC CRB index is projected to experience moderate volatility in the near term. A potential increase in demand from emerging markets could drive prices higher, particularly for industrial commodities. However, geopolitical uncertainties and potential supply chain disruptions pose a significant risk, which may trigger downward pressure on the index. Furthermore, an economic slowdown in major economies could also curb demand and dampen the overall performance.About TR/CC CRB Index
The Thomson Reuters/CoreCommodity CRB Index (TR/CC CRB) is a widely recognized benchmark reflecting the overall price movements of a basket of commodities. Established to gauge broad commodity market trends, the index comprises futures contracts across various sectors, including energy, agriculture, precious metals, and industrial metals. Its construction is based on the relative economic significance and trading liquidity of each commodity, ensuring a representative reflection of global commodity markets. The index is rebalanced periodically to maintain its accuracy and relevance.
Investors and analysts employ the TR/CC CRB Index as a key indicator of inflationary pressures, economic health, and market sentiment. It provides a valuable tool for diversifying investment portfolios and managing risk exposure in commodity markets. Moreover, the index acts as a reference point for derivative products, such as Exchange-Traded Funds (ETFs), allowing participants to gain broad exposure to the commodities market without directly trading individual futures contracts. Overall, the TR/CC CRB serves as a critical measure for understanding and analyzing the dynamics of the global commodity landscape.
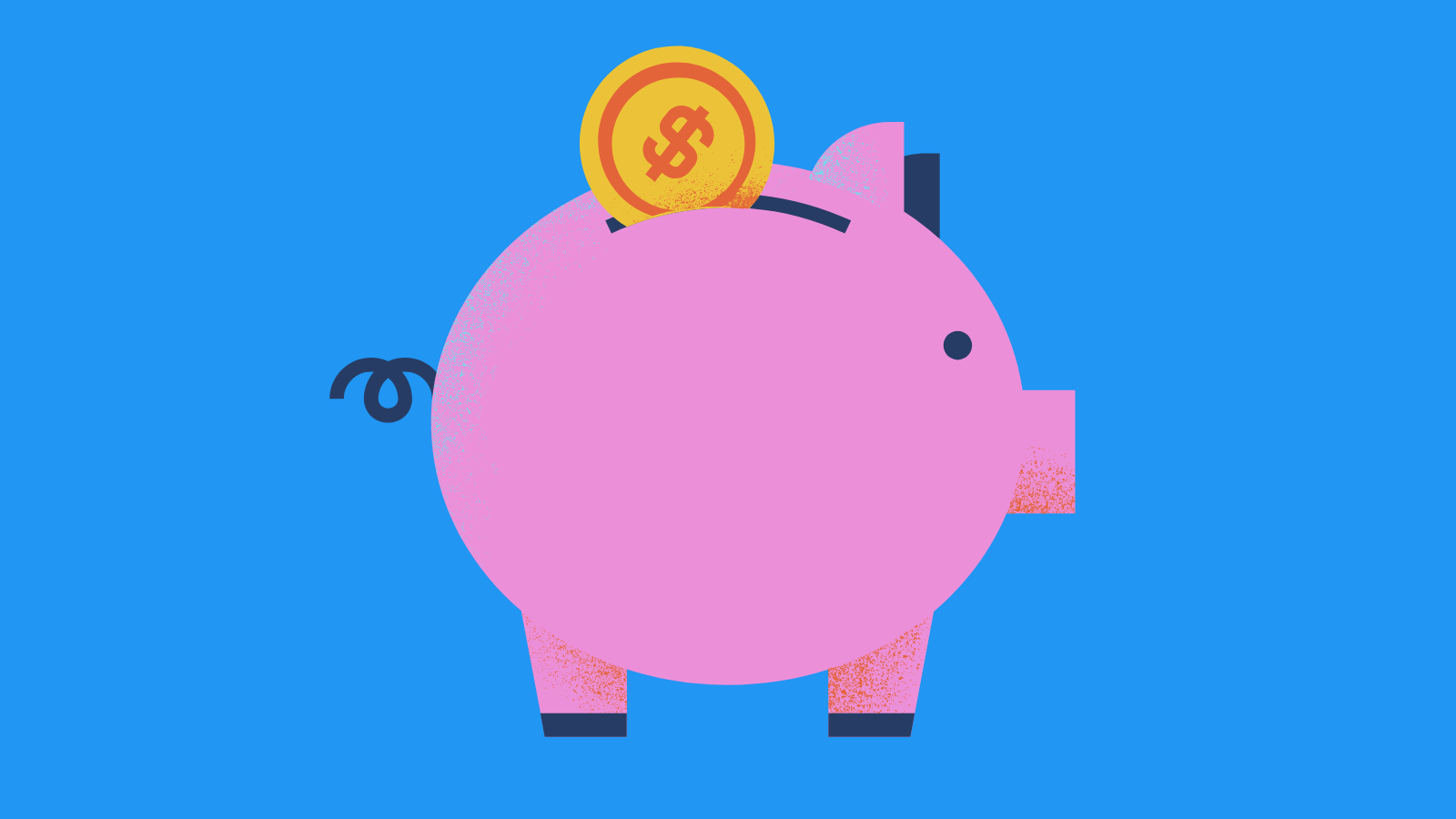
TR/CC CRB Index Forecasting Model
Our team of data scientists and economists proposes a machine learning model for forecasting the Thomson Reuters/CoreCommodity CRB (TR/CC CRB) index. The model's architecture will incorporate a hybrid approach, leveraging the strengths of both time series analysis and macroeconomic indicators. We will employ a **Recurrent Neural Network (RNN)**, specifically a Long Short-Term Memory (LSTM) network, to capture the temporal dependencies and non-linear patterns inherent in the historical TR/CC CRB index data. This network is well-suited for handling sequential data, allowing it to effectively learn and predict future index movements based on past trends. To augment the model's predictive power, we will integrate a set of leading economic indicators as exogenous variables.
These macroeconomic factors will include, but are not limited to, **inflation rates (e.g., CPI, PPI), industrial production indices, global economic growth forecasts (e.g., GDP growth from major economies), exchange rates (USD relative to other major currencies), and interest rate levels**. These variables are crucial for understanding the fundamental forces that influence commodity prices. The model will be trained on a comprehensive dataset spanning several years, incorporating both the TR/CC CRB index's historical values and the relevant macroeconomic data. Feature engineering will be performed to transform and standardize the data, ensuring optimal performance of the LSTM network. This includes handling missing values, scaling the data, and potentially creating lagged variables to capture delayed effects of macroeconomic changes on the index. The model will be validated using a rigorous methodology, employing techniques such as k-fold cross-validation and backtesting, to assess its accuracy and reliability.
The final model will produce point forecasts for the TR/CC CRB index at various time horizons. The model's output will be accompanied by a set of confidence intervals that estimate the predictive uncertainty. Additionally, we will perform a sensitivity analysis, determining which input variables exert the greatest impact on model predictions. This will enable us to explain the model's forecasts and provide insights into the factors that are most critical for the future performance of the TR/CC CRB index. **The model's performance will be constantly monitored and retrained to maintain accuracy and adapt to the evolving dynamics of the commodity markets.** The model is designed to be a valuable tool for investors, policymakers, and other stakeholders to make informed decisions in the commodities market.
ML Model Testing
n:Time series to forecast
p:Price signals of TR/CC CRB index
j:Nash equilibria (Neural Network)
k:Dominated move of TR/CC CRB index holders
a:Best response for TR/CC CRB target price
For further technical information as per how our model work we invite you to visit the article below:
How do KappaSignal algorithms actually work?
TR/CC CRB Index Forecast Strategic Interaction Table
Strategic Interaction Table Legend:
X axis: *Likelihood% (The higher the percentage value, the more likely the event will occur.)
Y axis: *Potential Impact% (The higher the percentage value, the more likely the price will deviate.)
Z axis (Grey to Black): *Technical Analysis%
TR/CC CRB Index: Financial Outlook and Forecast
The Thomson Reuters/CoreCommodity CRB (TR/CC CRB) Index, a key benchmark for the commodity markets, reflects the overall performance of a basket of 19 different commodities. Its financial outlook is intricately linked to global economic conditions, geopolitical events, and supply-demand dynamics inherent in the commodity sectors it represents. Recent observations point towards a mixed bag of factors influencing the index's trajectory. Elevated inflation, coupled with tightening monetary policies in major economies, creates headwinds for commodity demand, as it can dampen economic growth and consumer spending. At the same time, geopolitical tensions, particularly those impacting energy and agricultural markets, continue to inject volatility, potentially driving prices higher in specific commodity segments. Understanding the interplay of these varied forces is critical in evaluating the future direction of the index.
A nuanced understanding of the individual commodity components is essential for a comprehensive outlook. The energy sector, comprising a significant portion of the index, is particularly sensitive to global supply disruptions and demand fluctuations. Oil prices are impacted by OPEC+ decisions, geopolitical uncertainties, and the pace of the global economic recovery. Agricultural commodities, such as grains and soft commodities, are subject to weather patterns, crop yields, and trade policies. Industrial metals respond to the global manufacturing activity and infrastructure investment. Moreover, the strength of the US dollar, often inversely related to commodity prices, can significantly influence the index's performance. A stronger dollar may exert downward pressure on commodity prices, while a weaker dollar could provide upward support. Monitoring the performance of each sub-index segment will thus provide critical insight into the overall index movement.
Forecasting the TR/CC CRB Index involves considering a range of economic and market indicators. Monitoring leading economic indicators, such as Purchasing Managers' Indices (PMIs) and consumer confidence levels, can provide insights into future demand. Changes in inventory levels and production capacities, particularly in energy and metals, also serve as crucial signals. Furthermore, analyzing futures market dynamics, including the term structure of commodity prices and the level of speculative positioning, may offer valuable insights into investor sentiment and expectations. Commodity-specific factors, such as weather patterns, supply chain disruptions, and regulatory changes will continue to hold significant importance. An integrated approach, considering both macro-economic data and individual commodity drivers, will therefore improve the predictive capacity for the TR/CC CRB Index's direction.
The outlook for the TR/CC CRB Index in the near to medium term appears cautiously optimistic, with the potential for moderate growth. This prediction hinges on the assumption of a gradual easing of inflationary pressures, a stabilization in global economic growth, and the absence of major geopolitical shocks. The primary risk to this positive outlook includes a sharper-than-expected economic slowdown, a further escalation of geopolitical conflicts disrupting supply chains, and a sustained rise in the US dollar. Additionally, extreme weather events potentially affecting agricultural production and prolonged disruptions in key commodity markets would present considerable downside risks. Prudent management of these risks, coupled with careful monitoring of macroeconomic trends and commodity-specific developments, will be essential to navigate the index's future trajectory.
Rating | Short-Term | Long-Term Senior |
---|---|---|
Outlook | B2 | Ba3 |
Income Statement | Baa2 | C |
Balance Sheet | Caa2 | Baa2 |
Leverage Ratios | B3 | Baa2 |
Cash Flow | Caa2 | Baa2 |
Rates of Return and Profitability | B2 | C |
*An aggregate rating for an index summarizes the overall sentiment towards the companies it includes. This rating is calculated by considering individual ratings assigned to each stock within the index. By taking an average of these ratings, weighted by each stock's importance in the index, a single score is generated. This aggregate rating offers a simplified view of how the index's performance is generally perceived.
How does neural network examine financial reports and understand financial state of the company?
References
- M. L. Littman. Markov games as a framework for multi-agent reinforcement learning. In Ma- chine Learning, Proceedings of the Eleventh International Conference, Rutgers University, New Brunswick, NJ, USA, July 10-13, 1994, pages 157–163, 1994
- LeCun Y, Bengio Y, Hinton G. 2015. Deep learning. Nature 521:436–44
- T. Morimura, M. Sugiyama, M. Kashima, H. Hachiya, and T. Tanaka. Nonparametric return distribution ap- proximation for reinforcement learning. In Proceedings of the 27th International Conference on Machine Learning, pages 799–806, 2010
- Y. Le Tallec. Robust, risk-sensitive, and data-driven control of Markov decision processes. PhD thesis, Massachusetts Institute of Technology, 2007.
- Dimakopoulou M, Zhou Z, Athey S, Imbens G. 2018. Balanced linear contextual bandits. arXiv:1812.06227 [cs.LG]
- Mikolov T, Yih W, Zweig G. 2013c. Linguistic regularities in continuous space word representations. In Pro- ceedings of the 2013 Conference of the North American Chapter of the Association for Computational Linguistics: Human Language Technologies, pp. 746–51. New York: Assoc. Comput. Linguist.
- Ruiz FJ, Athey S, Blei DM. 2017. SHOPPER: a probabilistic model of consumer choice with substitutes and complements. arXiv:1711.03560 [stat.ML]