AUC Score :
Short-term Tactic1 :
Dominant Strategy :
Time series to forecast n:
ML Model Testing : Modular Neural Network (DNN Layer)
Hypothesis Testing : Chi-Square
Surveillance : Major exchange and OTC
1Short-term revised.
2Time series is updated based on short-term trends.
Key Points
Sun Life stock is anticipated to experience steady growth, fueled by its robust insurance and asset management segments. Positive trends in the global insurance market and strategic acquisitions are likely to boost revenue and profitability. However, this outlook faces several risks. Changes in interest rates could negatively affect investment returns and the value of insurance liabilities. Economic downturns or market volatility may reduce demand for financial products. Increased competition within the insurance and asset management industries and regulatory changes pose potential threats. Furthermore, the company's exposure to catastrophic events and related claims could strain its financial performance.About Sun Life Financial
Sun Life Financial Inc., a prominent financial services firm, offers a wide array of insurance and wealth management solutions. Operating globally, the company provides life insurance, health insurance, and various investment products and services. These offerings cater to individual clients, businesses, and institutional investors across several key markets, including Canada, the United States, the United Kingdom, and Asia. Sun Life's core business revolves around helping customers achieve financial security and manage risk through a diversified portfolio of financial services.
The company's strategic priorities are centered on customer focus, innovation, and expansion. Sun Life emphasizes building strong relationships with its clients and leveraging technology to enhance service delivery. Moreover, the firm actively seeks opportunities to grow its business through strategic acquisitions and geographic diversification. This commitment to growth and customer satisfaction positions Sun Life as a significant player in the global financial services landscape.
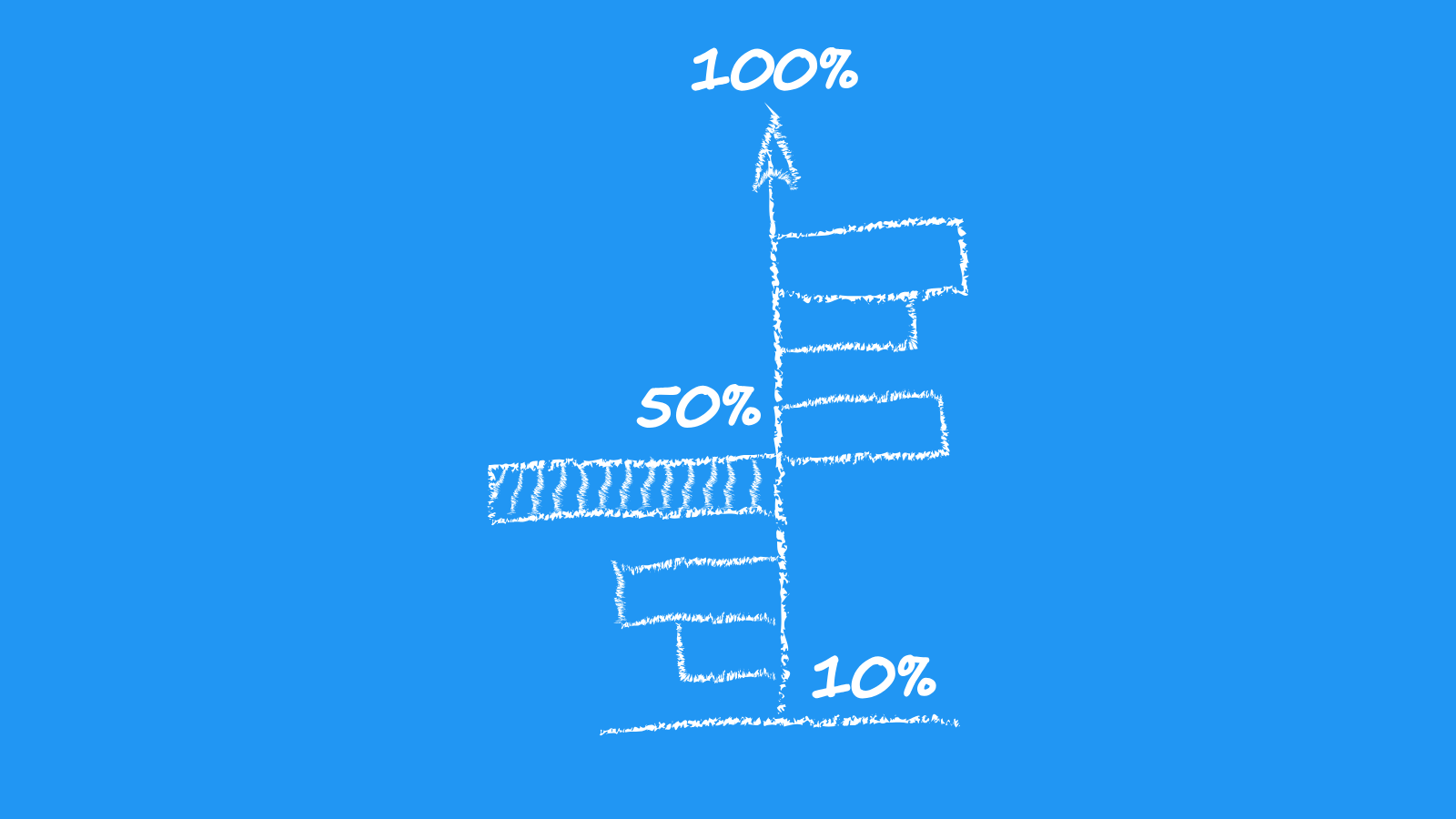
SLF Stock Prediction Model
Our team, comprised of data scientists and economists, proposes a comprehensive machine learning model to forecast the performance of Sun Life Financial Inc. (SLF) common stock. This model integrates several key data sources. First, we will incorporate historical stock prices and trading volumes to capture time-series trends, volatility patterns, and momentum signals using algorithms such as Recurrent Neural Networks (RNNs), specifically LSTMs, and ARIMA models. Second, macroeconomic indicators, including interest rates (e.g., the 10-year Treasury yield), inflation rates (e.g., CPI), GDP growth, and unemployment rates, will be included. These factors heavily influence the financial services sector. Third, financial data extracted from SLF's financial statements (e.g., revenue, earnings per share, book value, debt-to-equity ratio) will be utilized. These financial data will be key for assessing the company's financial health and profitability.
The model will employ a hybrid approach. The initial phase involves feature engineering, where raw data are transformed into informative variables. This includes calculating moving averages, volatility measures, and deriving economic indicators like yield curve slopes. We will then implement a combination of machine learning algorithms to address the inherent complexity of stock market predictions. Our primary model will utilize an ensemble approach, integrating predictions from various algorithms, including the aforementioned RNNs/LSTMs, ARIMA models, and potentially gradient boosting machines (e.g., XGBoost) that are adept at capturing non-linear relationships. We will also test the addition of Support Vector Machines (SVM) and random forests. The outputs of these models will be combined using a weighted averaging method, which assigns weights based on each model's past performance, evaluated using time-series cross-validation.
Finally, the model's performance will be evaluated using rigorous backtesting and out-of-sample validation. We will employ metrics like Mean Squared Error (MSE), Root Mean Squared Error (RMSE), Mean Absolute Error (MAE), and directional accuracy to assess the model's forecasting capabilities. Regular model retraining and recalibration based on the newest data will be a crucial part of our strategy to maintain forecasting accuracy over time. The findings of the forecasts will assist stakeholders in making well-informed decisions. By continuously refining our methodology, the model aims to provide reliable insights into the future of SLF stock performance, enabling proactive investment and risk management strategies.
ML Model Testing
n:Time series to forecast
p:Price signals of Sun Life Financial stock
j:Nash equilibria (Neural Network)
k:Dominated move of Sun Life Financial stock holders
a:Best response for Sun Life Financial target price
For further technical information as per how our model work we invite you to visit the article below:
How do KappaSignal algorithms actually work?
Sun Life Financial Stock Forecast (Buy or Sell) Strategic Interaction Table
Strategic Interaction Table Legend:
X axis: *Likelihood% (The higher the percentage value, the more likely the event will occur.)
Y axis: *Potential Impact% (The higher the percentage value, the more likely the price will deviate.)
Z axis (Grey to Black): *Technical Analysis%
Sun Life Financial Inc. (SLF) - Financial Outlook and Forecast
The financial outlook for SLF appears cautiously optimistic, reflecting the company's robust performance across its diverse business segments, including insurance, wealth management, and asset management. The company's strategic focus on growth in Asia, particularly in key markets like China and India, is expected to continue driving significant expansion. SLF's investments in digital transformation and technology are also anticipated to improve operational efficiency and enhance customer experience. Moreover, the company's strong capital position, coupled with its disciplined approach to risk management, positions it favorably to weather potential economic headwinds and capitalize on emerging opportunities. Recent acquisitions and partnerships further support SLF's strategic goals of geographic diversification and product innovation. The company's integrated approach to financial services offers synergistic benefits and potential for cross-selling opportunities, contributing to sustainable revenue growth.
SLF's wealth management business is expected to benefit from the growing demand for retirement planning and investment solutions, fueled by an aging population and increasing financial literacy. The company's asset management arm, MFS Investment Management, is well-positioned to capitalize on the ongoing demand for diversified investment products and active management strategies. Furthermore, the insurance segment is expected to remain a stable contributor to earnings, supported by the company's focus on product innovation, strategic partnerships, and distribution capabilities. The company's emphasis on sustainable and responsible investing is likely to resonate with increasingly environmentally and socially conscious investors, further supporting SLF's growth prospects. Management's consistent track record of delivering strong financial results and returning capital to shareholders through dividends and share repurchases underscores its commitment to value creation.
Key factors that will influence SLF's future performance include the evolving regulatory landscape, particularly in the insurance and wealth management sectors, and the potential for economic slowdowns in key markets. Interest rate fluctuations, inflation, and geopolitical uncertainties also represent important considerations. Competition from established financial institutions and fintech companies necessitates continued innovation and adaptability. SLF's ability to effectively manage its exposure to market volatility, credit risk, and operational risk will be critical in ensuring its financial stability. The company's success in integrating recent acquisitions, achieving cost synergies, and expanding its distribution network are also key performance indicators. Furthermore, the ability to navigate changing consumer preferences, technological advancements, and the evolving insurance and investment landscape will be crucial for SLF's long-term success.
Overall, the outlook for SLF is positive, driven by its strategic initiatives, diversified business model, and solid financial foundation. We anticipate continued growth in key markets, particularly Asia, and sustained performance across its core business segments. This positive outlook is predicated on SLF's ability to effectively manage risks and adapt to changing market dynamics. Potential risks to this positive outlook include unexpected economic downturns, increased regulatory scrutiny, and a failure to adequately respond to disruptive technological advancements. However, SLF's history of prudent financial management and strategic execution suggests a strong capacity to navigate these challenges and deliver value to its stakeholders.
Rating | Short-Term | Long-Term Senior |
---|---|---|
Outlook | B1 | B2 |
Income Statement | C | Ba1 |
Balance Sheet | Baa2 | Caa2 |
Leverage Ratios | Baa2 | B2 |
Cash Flow | C | B3 |
Rates of Return and Profitability | Ba2 | Caa2 |
*Financial analysis is the process of evaluating a company's financial performance and position by neural network. It involves reviewing the company's financial statements, including the balance sheet, income statement, and cash flow statement, as well as other financial reports and documents.
How does neural network examine financial reports and understand financial state of the company?
References
- Chernozhukov V, Chetverikov D, Demirer M, Duflo E, Hansen C, Newey W. 2017. Double/debiased/ Neyman machine learning of treatment effects. Am. Econ. Rev. 107:261–65
- Mnih A, Kavukcuoglu K. 2013. Learning word embeddings efficiently with noise-contrastive estimation. In Advances in Neural Information Processing Systems, Vol. 26, ed. Z Ghahramani, M Welling, C Cortes, ND Lawrence, KQ Weinberger, pp. 2265–73. San Diego, CA: Neural Inf. Process. Syst. Found.
- Jiang N, Li L. 2016. Doubly robust off-policy value evaluation for reinforcement learning. In Proceedings of the 33rd International Conference on Machine Learning, pp. 652–61. La Jolla, CA: Int. Mach. Learn. Soc.
- Bottou L. 2012. Stochastic gradient descent tricks. In Neural Networks: Tricks of the Trade, ed. G Montavon, G Orr, K-R Müller, pp. 421–36. Berlin: Springer
- Hastie T, Tibshirani R, Friedman J. 2009. The Elements of Statistical Learning. Berlin: Springer
- C. Claus and C. Boutilier. The dynamics of reinforcement learning in cooperative multiagent systems. In Proceedings of the Fifteenth National Conference on Artificial Intelligence and Tenth Innovative Applications of Artificial Intelligence Conference, AAAI 98, IAAI 98, July 26-30, 1998, Madison, Wisconsin, USA., pages 746–752, 1998.
- Belsley, D. A. (1988), "Modelling and forecast reliability," International Journal of Forecasting, 4, 427–447.