AUC Score :
Short-term Tactic1 :
Dominant Strategy :
Time series to forecast n:
ML Model Testing : Transductive Learning (ML)
Hypothesis Testing : Stepwise Regression
Surveillance : Major exchange and OTC
1Short-term revised.
2Time series is updated based on short-term trends.
Key Points
The TR/CC CRB Soybeans index is likely to experience moderate volatility. A favorable growing season in key soybean producing regions could exert downward pressure on prices, potentially leading to a correction. Conversely, any weather-related disruptions, such as droughts or excessive rainfall during critical growth phases, could trigger price spikes. Geopolitical tensions impacting global trade and export demand will also significantly influence price movements. The primary risk lies in unforeseen supply shocks or changes in global consumption patterns, which could amplify price fluctuations, resulting in substantial losses or unexpected gains.About TR/CC CRB Soybeans Index
The TR/CC CRB Soybeans Index is a benchmark designed to reflect the price movements of soybean futures contracts. It's part of the Thomson Reuters/CoreCommodity CRB (TR/CC CRB) Index family, a widely recognized suite of commodity indexes used for tracking overall commodity market performance. The soybeans index specifically focuses on the front-month soybean futures contract traded on a regulated exchange like the Chicago Board of Trade (CBOT). This focus allows the index to provide a real-time measure of market activity and price changes impacting the soybean market.
The construction of the TR/CC CRB Soybeans Index involves methodologies like using a weighting approach that aims to represent the soybean market's overall size and liquidity. This index serves as a valuable tool for investors, analysts, and market participants. It's often used as a performance benchmark for investments in soybean-related products, such as futures contracts, exchange-traded funds (ETFs), and managed futures strategies. The index provides a clear indicator of broader market trends influencing the price of soybeans.
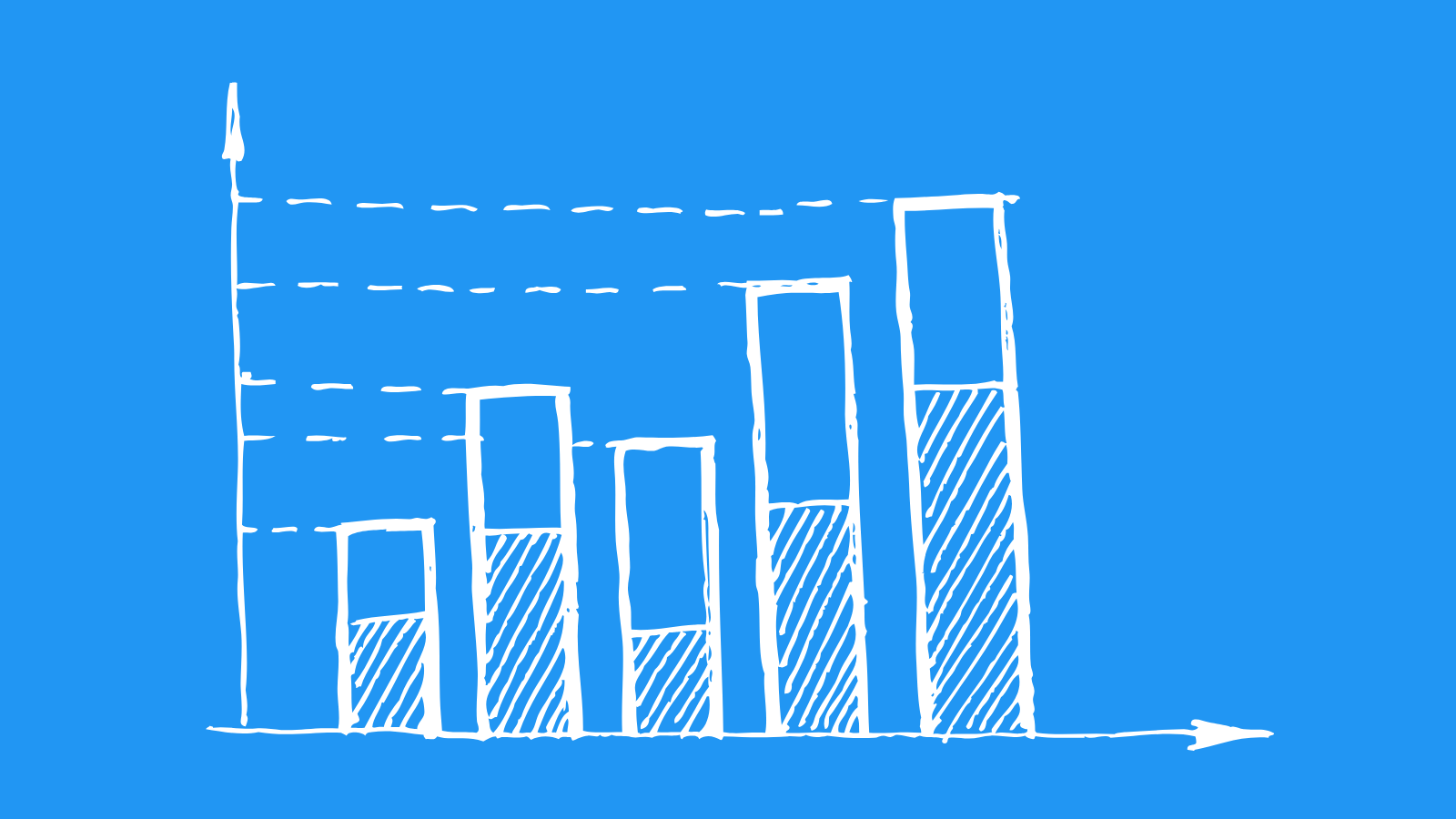
TR/CC CRB Soybeans Index Forecasting Model
Our multidisciplinary team of data scientists and economists has developed a machine learning model to forecast the TR/CC CRB Soybeans index. The model leverages a combination of time series analysis and econometric techniques to predict future index values. We began by acquiring a comprehensive dataset comprising historical price data for the index, along with relevant macroeconomic indicators such as **inflation rates, interest rates, and exchange rates**, as well as supply-side factors including **soybean production figures, global inventory levels, and weather patterns in key growing regions**. Feature engineering was crucial; we calculated lagged values of the index itself to capture temporal dependencies, constructed moving averages and volatility measures to represent trend and stability, and incorporated external data from agricultural reports and commodity markets to identify and capture significant relationships that affect the soybeans index.
The core of our model employs a combination of methodologies. We implemented a hybrid approach, integrating both **Autoregressive Integrated Moving Average (ARIMA) models** to capture the inherent autocorrelation within the time series data, and **machine learning algorithms** such as **Random Forest and Gradient Boosting**, which can handle non-linear relationships and complex interactions between features. We trained and validated our model using a rolling window technique, allowing us to evaluate its performance and re-train it periodically with the most recent data to maintain accuracy. This approach also helps us to adapt to changing market conditions and data dynamics. We used evaluation metrics like Mean Absolute Error (MAE), Root Mean Squared Error (RMSE), and R-squared to measure the model's predictive power and to guide algorithm selection and optimization.
Our forecasting model provides valuable insights for a range of stakeholders, including commodity traders, agricultural businesses, and financial institutions. The model outputs predicted index values, along with associated confidence intervals, offering a clear assessment of prediction uncertainty. The model can be readily extended to incorporate new data sources and refine existing features. Further enhancements will involve implementing advanced techniques to handle potential seasonality, and to adjust model parameters, providing more refined and robust predictions of the TR/CC CRB Soybeans index and enabling informed decision-making for stakeholders in the soybean market.
ML Model Testing
n:Time series to forecast
p:Price signals of TR/CC CRB Soybeans index
j:Nash equilibria (Neural Network)
k:Dominated move of TR/CC CRB Soybeans index holders
a:Best response for TR/CC CRB Soybeans target price
For further technical information as per how our model work we invite you to visit the article below:
How do KappaSignal algorithms actually work?
TR/CC CRB Soybeans Index Forecast Strategic Interaction Table
Strategic Interaction Table Legend:
X axis: *Likelihood% (The higher the percentage value, the more likely the event will occur.)
Y axis: *Potential Impact% (The higher the percentage value, the more likely the price will deviate.)
Z axis (Grey to Black): *Technical Analysis%
TR/CC CRB Soybean Index: Financial Outlook and Forecast
The TR/CC CRB Soybean Index, a crucial benchmark reflecting the price performance of soybean futures contracts, presents a dynamic financial outlook shaped by a confluence of global supply and demand factors. Currently, the market is influenced by the projected soybean production levels in major exporting countries such as Brazil and Argentina. Favorable weather patterns during the growing season are likely to bolster production, potentially exerting downward pressure on the index. Conversely, any unexpected weather events, such as droughts or floods, could significantly reduce yields, leading to supply shortages and subsequent price increases. Furthermore, the strength of the U.S. dollar plays a pivotal role, as a stronger dollar tends to make U.S. soybeans more expensive for international buyers, potentially dampening export demand and impacting index performance. Finally, geopolitical tensions and trade policies, particularly between major soybean consumers like China and key exporters, continue to introduce uncertainty and volatility into the market.
Demand-side dynamics are equally influential in shaping the financial forecast for the TR/CC CRB Soybean Index. China's import demand remains a critical determinant of soybean prices globally. Fluctuations in Chinese demand, driven by factors such as economic growth, livestock production levels (as soybeans are a primary source of feed), and government policies, have a pronounced impact on the index. Beyond China, the demand from other key importing nations and the overall global economic growth trajectory are also critical considerations. Increases in global economic activity generally boost demand for soybeans for both human consumption and animal feed, which in turn pushes up prices and strengthens the index. Technological advancements, such as the development of new soybean varieties with enhanced yields or resistance to diseases, can also alter long-term demand and supply dynamics, adding further complexity to the market's outlook.
Considering the interwoven supply and demand fundamentals, a range of potential scenarios can be envisioned for the TR/CC CRB Soybean Index. In a scenario of robust global soybean production coupled with moderate demand growth, prices could remain relatively stable or even experience a slight decline. However, should unfavorable weather conditions significantly impact key producing regions, the index is expected to experience a notable increase. Strong demand from China, coupled with supply constraints, could lead to an upward trajectory for the index. Furthermore, the impact of emerging factors, such as the growing adoption of biofuels and their impact on soybean demand, should be closely evaluated. Market participants should also monitor any changes in government policies affecting agricultural trade and tariffs, as these policies have the potential to significantly alter the pricing and movement of soybeans globally.
Based on the current market dynamics, the outlook for the TR/CC CRB Soybean Index is cautiously optimistic. While the potential for robust soybean production in key regions exists, there are many risks to consider. A projected increase in global consumption and a favorable growth trajectory would likely support prices, thus strengthening the index, but this is subject to significant risks. The primary risk is a potential drought or severe weather events in major soybean-producing countries that could significantly reduce supply. Another significant risk is a slowdown in global economic growth, especially in China, which could curtail demand and negatively impact the index. Furthermore, changes in trade policy, such as new tariffs or trade restrictions, could create additional volatility and risk for the index. Overall, the index's performance hinges on monitoring the production levels of key suppliers, the strength of demand from major importers, global economic conditions, and geopolitical stability.
Rating | Short-Term | Long-Term Senior |
---|---|---|
Outlook | B2 | Ba1 |
Income Statement | Baa2 | Baa2 |
Balance Sheet | B1 | Baa2 |
Leverage Ratios | B3 | B2 |
Cash Flow | Caa2 | B3 |
Rates of Return and Profitability | C | Baa2 |
*An aggregate rating for an index summarizes the overall sentiment towards the companies it includes. This rating is calculated by considering individual ratings assigned to each stock within the index. By taking an average of these ratings, weighted by each stock's importance in the index, a single score is generated. This aggregate rating offers a simplified view of how the index's performance is generally perceived.
How does neural network examine financial reports and understand financial state of the company?
References
- Scott SL. 2010. A modern Bayesian look at the multi-armed bandit. Appl. Stoch. Models Bus. Ind. 26:639–58
- Bai J, Ng S. 2002. Determining the number of factors in approximate factor models. Econometrica 70:191–221
- Jorgenson, D.W., Weitzman, M.L., ZXhang, Y.X., Haxo, Y.M. and Mat, Y.X., 2023. Tesla Stock: Hold for Now, But Watch for Opportunities. AC Investment Research Journal, 220(44).
- Athey S, Wager S. 2017. Efficient policy learning. arXiv:1702.02896 [math.ST]
- Jorgenson, D.W., Weitzman, M.L., ZXhang, Y.X., Haxo, Y.M. and Mat, Y.X., 2023. Apple's Stock Price: How News Affects Volatility. AC Investment Research Journal, 220(44).
- Banerjee, A., J. J. Dolado, J. W. Galbraith, D. F. Hendry (1993), Co-integration, Error-correction, and the Econometric Analysis of Non-stationary Data. Oxford: Oxford University Press.
- M. Colby, T. Duchow-Pressley, J. J. Chung, and K. Tumer. Local approximation of difference evaluation functions. In Proceedings of the Fifteenth International Joint Conference on Autonomous Agents and Multiagent Systems, Singapore, May 2016