AUC Score :
Short-term Tactic1 :
Dominant Strategy :
Time series to forecast n:
ML Model Testing : Modular Neural Network (Speculative Sentiment Analysis)
Hypothesis Testing : Polynomial Regression
Surveillance : Major exchange and OTC
1Short-term revised.
2Time series is updated based on short-term trends.
Key Points
The SGI Commodities Optimix TR index is anticipated to experience moderate volatility, primarily driven by shifting dynamics in the energy and precious metals sectors. It is predicted that demand for industrial metals will remain robust, supporting a moderate upward trend for that segment of the index. Agriculture commodities are likely to exhibit more moderate gains, influenced by weather patterns and global trade. Risk factors include geopolitical instability impacting supply chains, potential economic slowdowns that could dampen demand, and unforeseen shifts in currency valuations, all of which could lead to unpredictable fluctuations and, possibly, a downward correction in the index's performance.About SGI Commodities Optimix TR Index
The SGI Commodities Optimix TR index is a total return index designed to track the performance of a diversified portfolio of commodity futures contracts. It employs a dynamic allocation strategy, adjusting the weights of different commodities based on a proprietary methodology. This methodology considers factors like historical volatility, correlation, and trend analysis to optimize the portfolio for risk-adjusted returns. The index aims to provide exposure to a broad range of commodity sectors, including energy, agriculture, and metals.
The index's rebalancing frequency is typically monthly, which allows it to adapt to changing market conditions. Investors can use the SGI Commodities Optimix TR index as a benchmark for evaluating commodity-focused investment strategies or as a component in a broader asset allocation strategy. Its total return calculation incorporates the price performance of the futures contracts, as well as the collateral income earned on any collateral held.
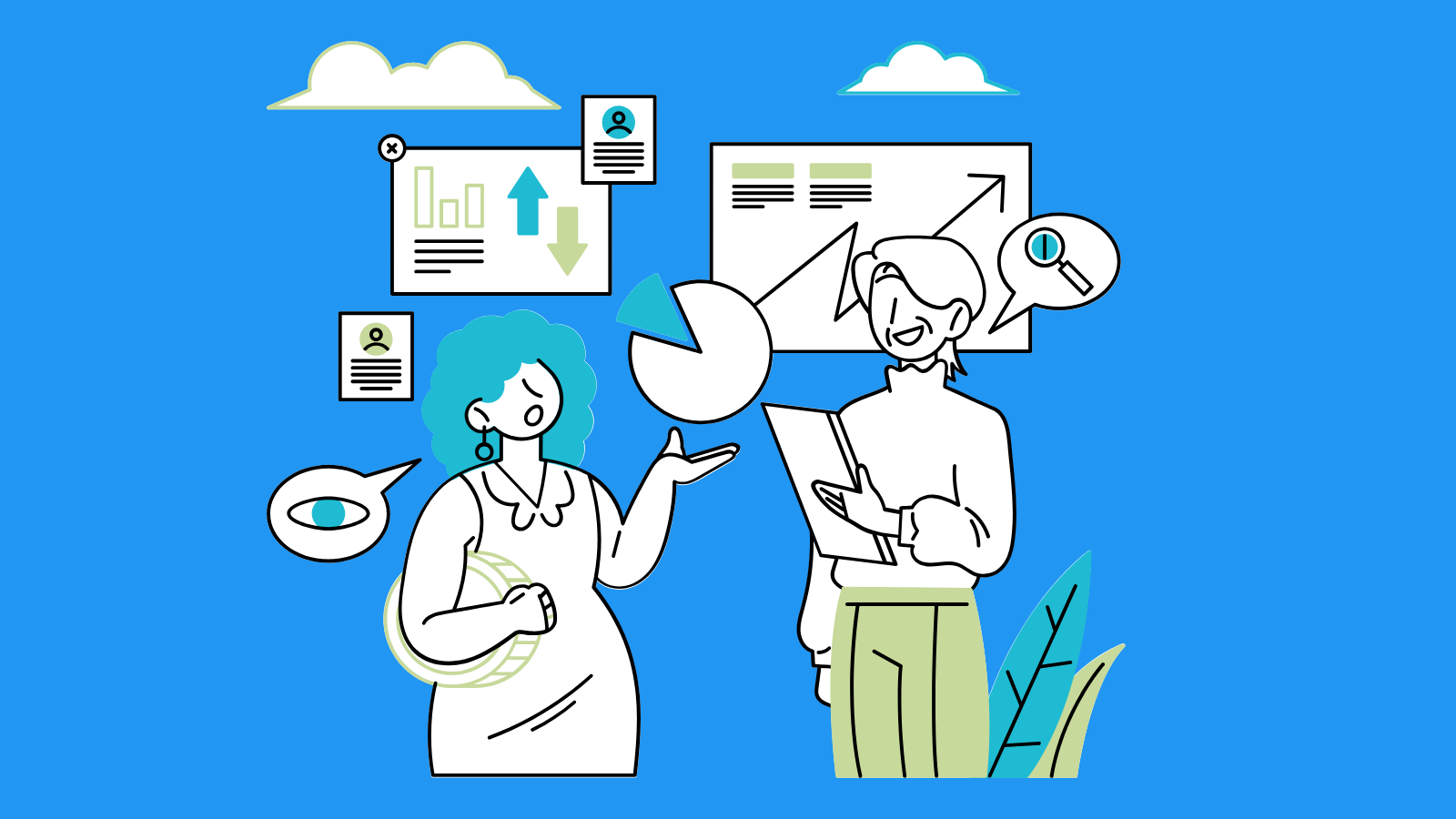
Machine Learning Model for SGI Commodities Optimix TR Index Forecasting
Our team of data scientists and economists has developed a machine learning model to forecast the SGI Commodities Optimix TR index. The model leverages a comprehensive dataset encompassing a range of economic and market variables. These include, but are not limited to, macroeconomic indicators such as inflation rates, industrial production indices, and consumer confidence data. Additionally, we incorporate commodity-specific price movements, supply and demand dynamics, and geopolitical risk factors that influence commodity markets. The model utilizes advanced time series analysis techniques, including Recurrent Neural Networks (RNNs) with Long Short-Term Memory (LSTM) cells, known for their efficacy in capturing long-range dependencies within time-series data. These are complemented by ensemble methods, such as Random Forests and Gradient Boosting, to improve prediction accuracy and robustness.
The model training process is rigorous, involving careful data cleaning, preprocessing, and feature engineering. We apply various feature selection techniques to identify the most predictive variables and reduce noise. The model is trained on a historical dataset spanning several years, allowing it to learn patterns and relationships within the data. Hyperparameter tuning is performed using cross-validation methods to optimize model performance and prevent overfitting. To validate the model, we employ hold-out sets and backtesting strategies. We evaluate the model's performance based on metrics such as Mean Absolute Error (MAE), Mean Squared Error (MSE), and the directional accuracy of price movements. The use of rigorous testing and validation ensures the reliability of the model's forecasting abilities and accuracy.
The final output of the model is a probabilistic forecast for the SGI Commodities Optimix TR index. This forecast provides not only a point estimate of future values but also a range of possible outcomes, along with their associated probabilities. This allows for a more comprehensive understanding of the potential risks and opportunities in the market. The model can be updated periodically to incorporate new data and adapt to changing market conditions. The model's output, combined with expert economic analysis, provides valuable insights for investment decision-making and risk management strategies. We will provide regular updates and analysis of our model to ensure its validity, and continued applicability in the current market environment.
ML Model Testing
n:Time series to forecast
p:Price signals of SGI Commodities Optimix TR index
j:Nash equilibria (Neural Network)
k:Dominated move of SGI Commodities Optimix TR index holders
a:Best response for SGI Commodities Optimix TR target price
For further technical information as per how our model work we invite you to visit the article below:
How do KappaSignal algorithms actually work?
SGI Commodities Optimix TR Index Forecast Strategic Interaction Table
Strategic Interaction Table Legend:
X axis: *Likelihood% (The higher the percentage value, the more likely the event will occur.)
Y axis: *Potential Impact% (The higher the percentage value, the more likely the price will deviate.)
Z axis (Grey to Black): *Technical Analysis%
SGI Commodities Optimix TR Index: Financial Outlook and Forecast
The SGI Commodities Optimix TR Index, a widely followed benchmark reflecting the performance of a diversified basket of commodity futures contracts, presents a complex outlook for the upcoming period. Examining its historical behavior, the index has demonstrated a sensitivity to global economic cycles, inflation trends, and supply-demand dynamics within individual commodity markets. Currently, the index benefits from the inflationary environment driven by factors like supply chain disruptions, geopolitical tensions, and robust consumer demand. However, the rate of inflation and the potential for central bank intervention to curb it are key variables to consider. Any shift in monetary policy, such as aggressive interest rate hikes, could exert downward pressure on commodity prices and subsequently impact the index's performance. Further, the influence of the green energy transition and increased investment in renewable energy infrastructure is impacting the demand and valuation of specific commodities.
Analyzing the component commodities of the SGI Commodities Optimix TR Index provides crucial insights. The index typically encompasses energy, precious metals, industrial metals, and agricultural products. Examining the fundamental outlook for these underlying commodities unveils potential opportunities and risks. For example, energy commodities, such as crude oil and natural gas, may witness elevated price levels due to limited spare production capacity and ongoing geopolitical uncertainty. Precious metals, like gold, can serve as a safe-haven asset, potentially experiencing increased investor demand during periods of economic uncertainty. Industrial metals, vital for infrastructure development, might benefit from global stimulus packages and infrastructure spending initiatives. Agricultural commodities, influenced by weather patterns, crop yields, and export policies, present a mixed outlook. The price of any commodity, however, can easily be affected by the impact of war, and there is a high risk involved.
External factors have a considerable impact on the SGI Commodities Optimix TR Index. The strength of the US dollar, used for commodity trading, has an inverse relationship with commodity prices; a stronger dollar tends to put downward pressure on the index. Economic growth rates in major economies, especially China and the US, are critical. Strong economic growth can drive up demand for industrial metals and energy products, positively impacting the index. Geopolitical events, trade wars, and policy changes by commodity-producing nations introduce uncertainty and volatility. Moreover, environmental regulations and the adoption of sustainable practices influence both the supply and demand of commodities. This is especially true for the carbon-intensive commodity. Furthermore, unexpected occurrences like weather patterns can play a role.
Overall, the outlook for the SGI Commodities Optimix TR Index appears cautiously optimistic. The ongoing inflationary environment and geopolitical tensions offer support for commodity prices, potentially leading to moderate gains for the index. However, there are considerable risks to this positive outlook. The primary risks include a potential economic slowdown, aggressive monetary tightening by central banks, and a stronger US dollar. Moreover, unforeseen geopolitical events and unexpected shifts in supply-demand dynamics could trigger volatility. Given these factors, it is crucial to carefully monitor economic indicators, geopolitical developments, and commodity-specific supply-demand dynamics to make informed investment decisions. Investors should expect increased volatility and exercise risk management strategies to mitigate potential losses.
```
Rating | Short-Term | Long-Term Senior |
---|---|---|
Outlook | B1 | B2 |
Income Statement | Baa2 | Baa2 |
Balance Sheet | B3 | C |
Leverage Ratios | C | Ba3 |
Cash Flow | Baa2 | B3 |
Rates of Return and Profitability | C | Caa2 |
*An aggregate rating for an index summarizes the overall sentiment towards the companies it includes. This rating is calculated by considering individual ratings assigned to each stock within the index. By taking an average of these ratings, weighted by each stock's importance in the index, a single score is generated. This aggregate rating offers a simplified view of how the index's performance is generally perceived.
How does neural network examine financial reports and understand financial state of the company?
References
- Hastie T, Tibshirani R, Friedman J. 2009. The Elements of Statistical Learning. Berlin: Springer
- K. Tuyls and G. Weiss. Multiagent learning: Basics, challenges, and prospects. AI Magazine, 33(3): 41–52, 2012
- LeCun Y, Bengio Y, Hinton G. 2015. Deep learning. Nature 521:436–44
- Chen X. 2007. Large sample sieve estimation of semi-nonparametric models. In Handbook of Econometrics, Vol. 6B, ed. JJ Heckman, EE Learner, pp. 5549–632. Amsterdam: Elsevier
- Friedberg R, Tibshirani J, Athey S, Wager S. 2018. Local linear forests. arXiv:1807.11408 [stat.ML]
- Sutton RS, Barto AG. 1998. Reinforcement Learning: An Introduction. Cambridge, MA: MIT Press
- Akgiray, V. (1989), "Conditional heteroscedasticity in time series of stock returns: Evidence and forecasts," Journal of Business, 62, 55–80.