AUC Score :
Short-term Tactic1 :
Dominant Strategy :
Time series to forecast n:
ML Model Testing : Modular Neural Network (CNN Layer)
Hypothesis Testing : Pearson Correlation
Surveillance : Major exchange and OTC
1Short-term revised.
2Time series is updated based on short-term trends.
Key Points
SQNS faces a future that is highly dependent on the success of its 5G and satellite IoT product offerings. The company is expected to experience moderate revenue growth if it successfully penetrates new markets, including agricultural, industrial, and utility sectors, fueled by increasing demand for IoT connectivity solutions. However, significant risk exists, including competition from larger, more established semiconductor companies, potential delays in product development and deployment, and dependency on the overall health of the global semiconductor market. SQNS's profitability is vulnerable to fluctuating component costs and potential supply chain disruptions, which could negatively impact margins and earnings. Another challenge would be the rapid technological shifts in wireless communication standards, thus, the company must be agile in adapting to maintain a competitive edge.About Sequans Communications
Sequans Communications S.A. is a fabless designer, developer and supplier of advanced, single-mode 4G and 5G semiconductor solutions. Headquartered in Paris, France, the company focuses on delivering chipsets and modules for the Internet of Things (IoT) and broadband connectivity markets. Sequans offers a wide range of products that enable cellular connectivity for various applications, including industrial IoT, asset tracking, wearables, and home automation. Their technology supports various cellular standards, ensuring compatibility across diverse geographical regions and applications.
The company's solutions are designed to provide high performance, low power consumption, and seamless integration. Sequans collaborates with leading industry partners and ecosystem providers to ensure compatibility and accelerate time-to-market for its customers. They are committed to continuous innovation and research, expanding their product portfolio and advancing cellular technology. Sequans aims to facilitate the widespread adoption of cellular IoT by providing reliable, cost-effective, and technologically advanced solutions globally.
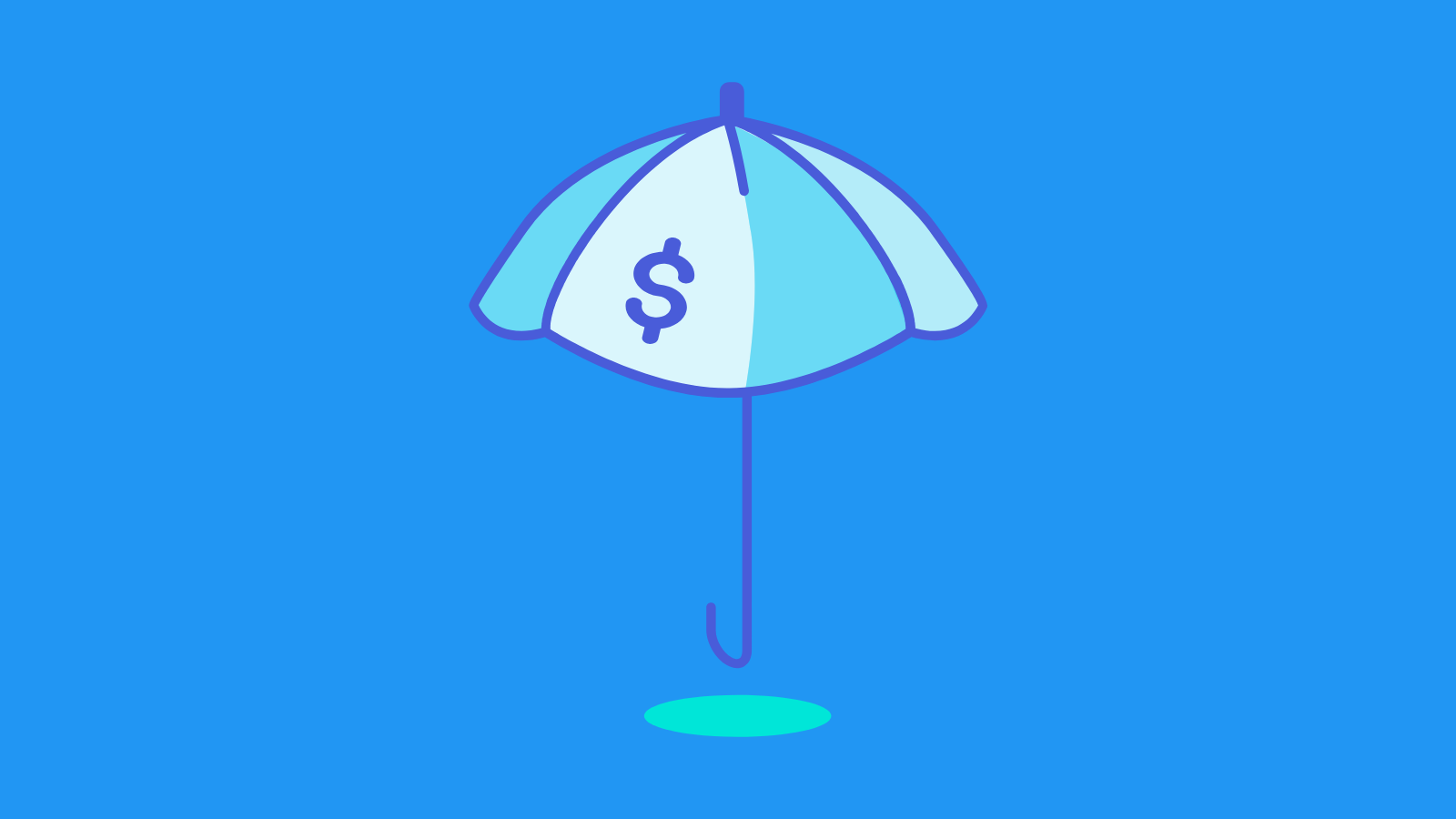
SQNS Stock Forecast: A Machine Learning Model
Our team, comprised of data scientists and economists, proposes a comprehensive machine learning model to forecast the performance of Sequans Communications S.A. (SQNS). The model will leverage a diverse dataset, encompassing both technical indicators and macroeconomic variables. Technical indicators will include moving averages, Relative Strength Index (RSI), and Moving Average Convergence Divergence (MACD), calculated over various time horizons to capture short-term and long-term trends. Econometric data, such as economic growth indicators, inflation rates, and interest rates, will be incorporated to understand the broader economic context that affects SQNS's operational environment. We will also consider industry-specific factors, including 5G adoption rates, competition in the chipset market, and news sentiment analysis. The model's training and testing will be performed on historical data from SQNS and the relevant economic dataset. This dataset will be split into training, validation and testing sets to assess the model's predictive capabilities.
The machine learning model will utilize an ensemble approach to enhance forecast accuracy and robustness. We will explore a combination of algorithms, including Recurrent Neural Networks (RNNs), Long Short-Term Memory (LSTM) networks, and Gradient Boosting Machines. RNNs and LSTMs are well-suited for time-series data, enabling the model to discern sequential patterns in SQNS's financial results and market behavior. Gradient Boosting Machines will be employed to identify the most important predictors and potential interactions. The model's performance will be evaluated using established metrics such as Mean Absolute Error (MAE), Mean Squared Error (MSE), and Root Mean Squared Error (RMSE). We will use cross-validation techniques to prevent overfitting and estimate generalization performance. The final output of the model will be a probability distribution of future stock performance, providing valuable insights for decision-making.
The implementation of this model involves rigorous data preprocessing, feature engineering, and hyperparameter tuning. Data cleaning will be performed to handle missing values and outliers, ensuring the model is provided with reliable data. Feature engineering will encompass the construction of additional variables from existing ones to capture relationships that might be otherwise missed. Hyperparameter optimization will be implemented to fine-tune the machine learning algorithm's settings to achieve the best performance. This involves automated search methods to identify the optimal configuration. The model's output will be presented in an easily understandable format, offering a forecast of SQNS's performance and explaining the factors influencing those predictions. This will enable stakeholders to make data-driven decisions and to mitigate risk. The model is designed to be dynamic, and it will be periodically updated and improved as new data becomes available.
ML Model Testing
n:Time series to forecast
p:Price signals of Sequans Communications stock
j:Nash equilibria (Neural Network)
k:Dominated move of Sequans Communications stock holders
a:Best response for Sequans Communications target price
For further technical information as per how our model work we invite you to visit the article below:
How do KappaSignal algorithms actually work?
Sequans Communications Stock Forecast (Buy or Sell) Strategic Interaction Table
Strategic Interaction Table Legend:
X axis: *Likelihood% (The higher the percentage value, the more likely the event will occur.)
Y axis: *Potential Impact% (The higher the percentage value, the more likely the price will deviate.)
Z axis (Grey to Black): *Technical Analysis%
Sequans Communications S.A. (SQNS) Financial Outlook and Forecast
The financial outlook for Sequans (SQNS) appears cautiously optimistic, underpinned by the growing demand for 5G and massive IoT connectivity solutions. The company's focus on providing advanced 5G and LTE-Advanced chipsets for various applications, including broadband, industrial IoT, and massive IoT, positions it favorably within a rapidly expanding market. Revenue growth is projected to be driven by increased adoption of 5G technologies globally, coupled with the continued expansion of IoT deployments across different industries. Furthermore, SQNS's strategic partnerships and collaborations with key players in the telecommunications and technology sectors are expected to contribute to its market penetration and revenue streams. The company's commitment to innovation, as evidenced by its ongoing research and development efforts, suggests that SQNS can adapt and capitalize on future technological advancements.
SQNS's forecast points towards solid revenue growth in the coming years. Analysts predict a significant upswing in sales as the company continues to secure design wins and scale production of its 5G and LTE-Advanced chipsets. The expansion of 5G infrastructure, coupled with the proliferation of IoT devices, creates a supportive backdrop for its growth. The company's ability to deliver high-performance, low-power solutions makes it attractive to a wide range of customers in the growing IoT market. Profitability, though, may be influenced by several factors, including the costs associated with research and development, the competitive landscape, and the pricing strategies. SQNS's management anticipates the benefits from economies of scale will provide some improvement in profit margins as sales volumes increase.
SQNS is well-positioned to benefit from favorable macro trends. The increased demand for high-speed data connectivity, particularly in areas like fixed wireless access (FWA), is expected to fuel the demand for SQNS's solutions. The company's focus on the massive IoT segment offers a long-term growth opportunity as more devices connect to networks and require connectivity. The company has been focused on cost optimization which is expected to contribute to its profitability moving forward. The company's strong financial position, enhanced by the ability to secure funding, provides it with flexibility to invest in future growth. Furthermore, its diversified customer base helps mitigate the risks associated with relying on any one particular customer or application.
In conclusion, SQNS shows a positive outlook, driven by strong demand for its products, technological leadership, and strategic positioning. The forecast is for sustained revenue growth and improved profitability in the long-term. However, it is important to consider the risks associated with the business. Intense competition, supply chain constraints, and the cyclical nature of the semiconductor industry could impact the company's financial performance. There is a risk of unexpected challenges in product development and commercialization. Despite the risks, the predicted outcome is positive, with SQNS likely to capitalize on the expanding 5G and massive IoT markets.
Rating | Short-Term | Long-Term Senior |
---|---|---|
Outlook | Ba3 | Ba3 |
Income Statement | Baa2 | Baa2 |
Balance Sheet | C | Baa2 |
Leverage Ratios | Baa2 | Ba1 |
Cash Flow | Baa2 | B1 |
Rates of Return and Profitability | C | C |
*Financial analysis is the process of evaluating a company's financial performance and position by neural network. It involves reviewing the company's financial statements, including the balance sheet, income statement, and cash flow statement, as well as other financial reports and documents.
How does neural network examine financial reports and understand financial state of the company?
References
- G. Theocharous and A. Hallak. Lifetime value marketing using reinforcement learning. RLDM 2013, page 19, 2013
- Mazumder R, Hastie T, Tibshirani R. 2010. Spectral regularization algorithms for learning large incomplete matrices. J. Mach. Learn. Res. 11:2287–322
- Jacobs B, Donkers B, Fok D. 2014. Product Recommendations Based on Latent Purchase Motivations. Rotterdam, Neth.: ERIM
- Chow, G. C. (1960), "Tests of equality between sets of coefficients in two linear regressions," Econometrica, 28, 591–605.
- Breusch, T. S. A. R. Pagan (1979), "A simple test for heteroskedasticity and random coefficient variation," Econometrica, 47, 1287–1294.
- G. J. Laurent, L. Matignon, and N. L. Fort-Piat. The world of independent learners is not Markovian. Int. J. Know.-Based Intell. Eng. Syst., 15(1):55–64, 2011
- L. Panait and S. Luke. Cooperative multi-agent learning: The state of the art. Autonomous Agents and Multi-Agent Systems, 11(3):387–434, 2005.