AUC Score :
Short-term Tactic1 :
Dominant Strategy :
Time series to forecast n:
ML Model Testing : Modular Neural Network (Market News Sentiment Analysis)
Hypothesis Testing : Wilcoxon Rank-Sum Test
Surveillance : Major exchange and OTC
1Short-term revised.
2Time series is updated based on short-term trends.
Key Points
Seadrill's shares are expected to experience moderate volatility. Anticipated positive catalysts include the continued recovery in the offshore drilling market, particularly for harsh environment assets, and the successful execution of its current contracts and future projects. Potential risks involve delays or cancellations of new projects, fluctuations in oil prices impacting demand, competition from rival drilling companies, and potential setbacks in ongoing restructuring efforts. The company's substantial debt burden, combined with its exposure to cyclical commodity markets, could lead to considerable downside risks. Further downside could be triggered by unforeseen operational issues, changes in environmental regulations, or shifts in geopolitical dynamics that destabilize the offshore drilling sector.About Seadrill Limited
Seadrill is a Bermuda-based offshore drilling contractor, providing services to the oil and gas industry worldwide. The company operates a fleet of offshore drilling rigs, including ultra-deepwater drillships, harsh environment semi-submersible rigs, and jack-up rigs. These rigs are used for drilling and completing oil and gas wells, primarily in deep and ultra-deepwater environments. Sdr, along with its subsidiaries, offers services throughout the lifecycle of offshore oil and gas fields, which include exploration, development, and production phases.
The company's clients are primarily major and independent oil and gas companies. Seadrill has a global presence, with operations in key offshore drilling regions such as the Americas, Africa, the North Sea, and Southeast Asia. Sdr's business model relies heavily on long-term contracts with its clients to secure revenue. The company is subject to the cyclical nature of the offshore drilling industry and is influenced by factors such as oil prices, exploration and production spending, and rig supply and demand.
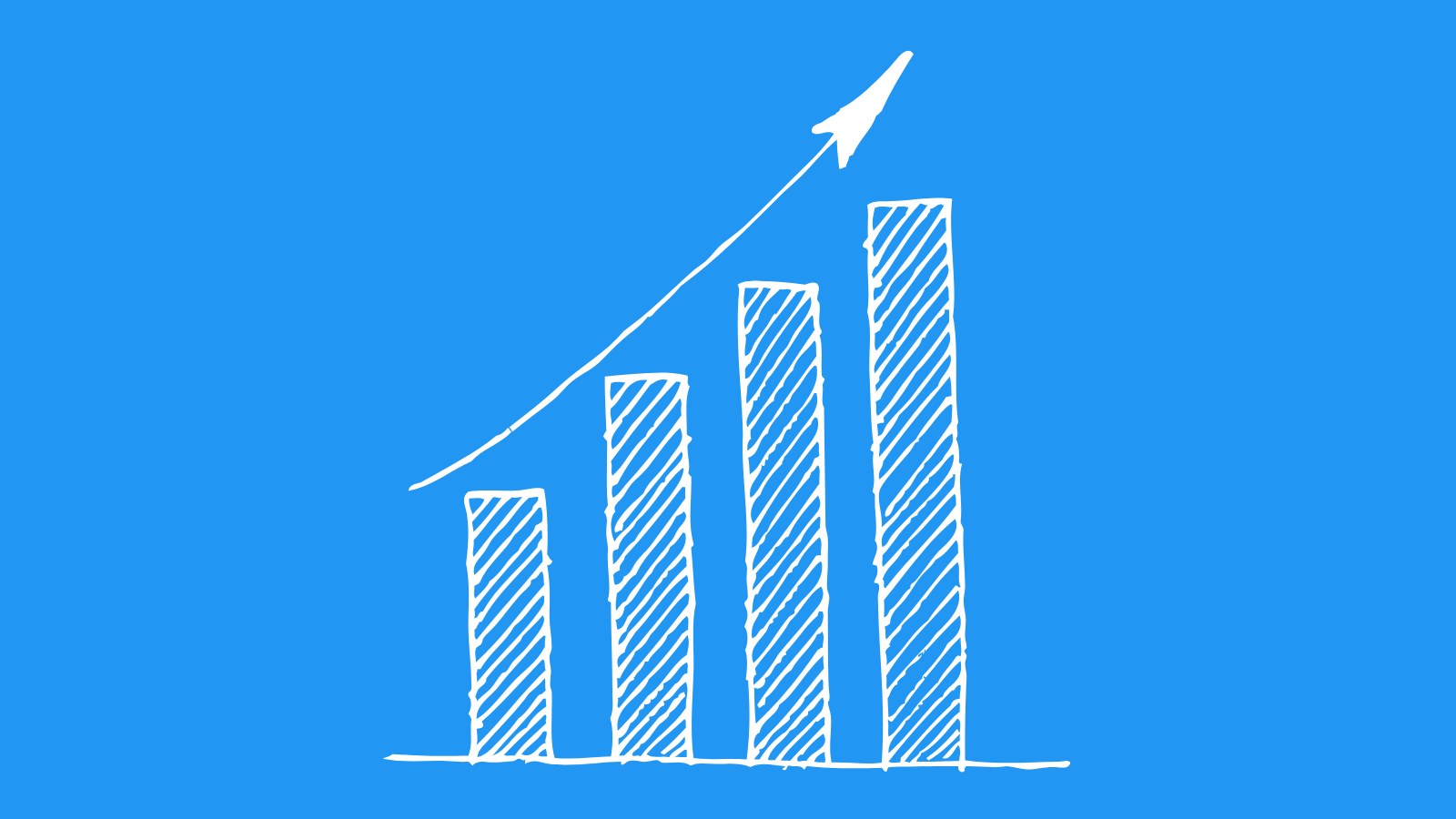
SDRL Stock Forecast: A Machine Learning Model Approach
Our team of data scientists and economists has developed a sophisticated machine learning model to forecast the performance of Seadrill Limited Common Shares (SDRL). The model integrates a diverse range of data sources, including historical SDRL trading data (e.g., volume, open, close, high, low), macroeconomic indicators (e.g., oil prices, global economic growth, interest rates), and industry-specific information (e.g., offshore drilling rig utilization rates, competitor performance, and supply/demand dynamics in the energy sector). The model utilizes advanced algorithms such as Recurrent Neural Networks (RNNs) and Long Short-Term Memory (LSTM) networks, which are particularly adept at capturing temporal dependencies within the data. We've incorporated techniques to address potential issues like multicollinearity and non-stationarity to improve the reliability of our predictions.
The model's architecture includes several key components. First, we preprocess the raw data by cleaning missing values, scaling features, and transforming variables to improve model convergence. Second, we split the dataset into training, validation, and test sets to rigorously assess model performance. The training set is used to train the model parameters, while the validation set is used to tune hyperparameters and prevent overfitting. Finally, the test set is used to evaluate the model's accuracy in predicting future SDRL performance. During the model's training phase, we have employed several feature engineering strategies, including the creation of technical indicators (e.g., moving averages, Relative Strength Index) to capture short-term price movements.
The output of our model is a probabilistic forecast, providing not only point estimates but also confidence intervals. This allows investors to assess the range of potential outcomes, accounting for inherent market uncertainties. The model's performance is continually monitored and updated through backtesting and regular retraining with new data. We also incorporate a layer of human oversight, where our economists scrutinize the model's outputs and provide context and insights based on their understanding of the industry and market conditions. This combined approach ensures the model's relevance and its effective application in informing investment decisions related to SDRL stock.
```
ML Model Testing
n:Time series to forecast
p:Price signals of Seadrill Limited stock
j:Nash equilibria (Neural Network)
k:Dominated move of Seadrill Limited stock holders
a:Best response for Seadrill Limited target price
For further technical information as per how our model work we invite you to visit the article below:
How do KappaSignal algorithms actually work?
Seadrill Limited Stock Forecast (Buy or Sell) Strategic Interaction Table
Strategic Interaction Table Legend:
X axis: *Likelihood% (The higher the percentage value, the more likely the event will occur.)
Y axis: *Potential Impact% (The higher the percentage value, the more likely the price will deviate.)
Z axis (Grey to Black): *Technical Analysis%
Seadrill Limited Common Shares: Financial Outlook and Forecast
The financial outlook for SDRL is currently characterized by a complex interplay of factors within the offshore drilling industry. The company, emerging from a period of restructuring, is now positioned to benefit from anticipated improvements in the global energy market. Increased oil prices, driven by geopolitical instability and rising demand, have created a more favorable environment for offshore exploration and production. This translates to greater demand for drilling services, potentially increasing utilization rates and day rates for SDRL's fleet. Furthermore, SDRL's recent strategic initiatives, including fleet optimization and cost reduction measures, are expected to contribute to improved profitability and enhanced financial stability. However, the company faces challenges from competition and the global energy market.
The future financial performance of SDRL will be heavily influenced by several key factors. Fleet utilization rates are paramount; higher rates directly translate to increased revenue. The level of day rates obtained for SDRL's rigs is also crucial. These rates depend on the specific type of rig, its location, and the overall market conditions. Long-term contracts provide stability in revenue streams, while spot market exposure can offer opportunities for higher day rates but also introduces volatility. Moreover, SDRL's ability to manage its debt, which is significant following its restructuring, is a critical determinant of its financial health. The company must efficiently service its debt obligations and proactively manage its capital structure to maintain a sustainable financial position. Additionally, SDRL's operational efficiency and its ability to control operating costs will significantly impact its profitability.
The macroeconomic environment plays a significant role in the forecast. Factors such as global oil supply and demand dynamics, geopolitical risks, and fluctuations in commodity prices directly influence the offshore drilling market. Continued investment in exploration and production projects by oil and gas companies is essential for SDRL's success. Conversely, economic downturns or shifts in energy policy that favor renewable sources could negatively impact demand for offshore drilling services. The capital expenditure (CAPEX) decisions of oil and gas companies are crucial; increased CAPEX translates to more drilling activity and higher demand for SDRL's services. Regulatory changes related to environmental sustainability and emissions standards also present challenges and opportunities for the company, requiring significant investment in rig upgrades and compliance.
Overall, the financial outlook for SDRL appears cautiously optimistic. It is predicted that the company will experience moderate growth in the coming years, supported by improving market conditions and its ongoing efforts to improve efficiency. However, this prediction is subject to several risks. The volatility of oil prices, potential oversupply of drilling rigs, and geopolitical uncertainties could significantly impact the company's performance. Additionally, any unforeseen operational disruptions, such as major rig failures or delays in projects, could negatively affect revenues and profitability. The increasing emphasis on energy transition towards renewable sources creates a long-term threat to the offshore drilling market. Careful management of debt, prudent capital allocation, and adaptability to changing market dynamics will be essential for SDRL to achieve its financial objectives and secure sustainable growth.
Rating | Short-Term | Long-Term Senior |
---|---|---|
Outlook | Ba2 | B2 |
Income Statement | B2 | Baa2 |
Balance Sheet | Baa2 | C |
Leverage Ratios | Baa2 | C |
Cash Flow | C | B2 |
Rates of Return and Profitability | Baa2 | B3 |
*Financial analysis is the process of evaluating a company's financial performance and position by neural network. It involves reviewing the company's financial statements, including the balance sheet, income statement, and cash flow statement, as well as other financial reports and documents.
How does neural network examine financial reports and understand financial state of the company?
References
- A. Y. Ng, D. Harada, and S. J. Russell. Policy invariance under reward transformations: Theory and application to reward shaping. In Proceedings of the Sixteenth International Conference on Machine Learning (ICML 1999), Bled, Slovenia, June 27 - 30, 1999, pages 278–287, 1999.
- Athey S, Tibshirani J, Wager S. 2016b. Generalized random forests. arXiv:1610.01271 [stat.ME]
- L. Prashanth and M. Ghavamzadeh. Actor-critic algorithms for risk-sensitive MDPs. In Proceedings of Advances in Neural Information Processing Systems 26, pages 252–260, 2013.
- E. Altman, K. Avrachenkov, and R. N ́u ̃nez-Queija. Perturbation analysis for denumerable Markov chains with application to queueing models. Advances in Applied Probability, pages 839–853, 2004
- Künzel S, Sekhon J, Bickel P, Yu B. 2017. Meta-learners for estimating heterogeneous treatment effects using machine learning. arXiv:1706.03461 [math.ST]
- Ruiz FJ, Athey S, Blei DM. 2017. SHOPPER: a probabilistic model of consumer choice with substitutes and complements. arXiv:1711.03560 [stat.ML]
- V. Konda and J. Tsitsiklis. Actor-Critic algorithms. In Proceedings of Advances in Neural Information Processing Systems 12, pages 1008–1014, 2000